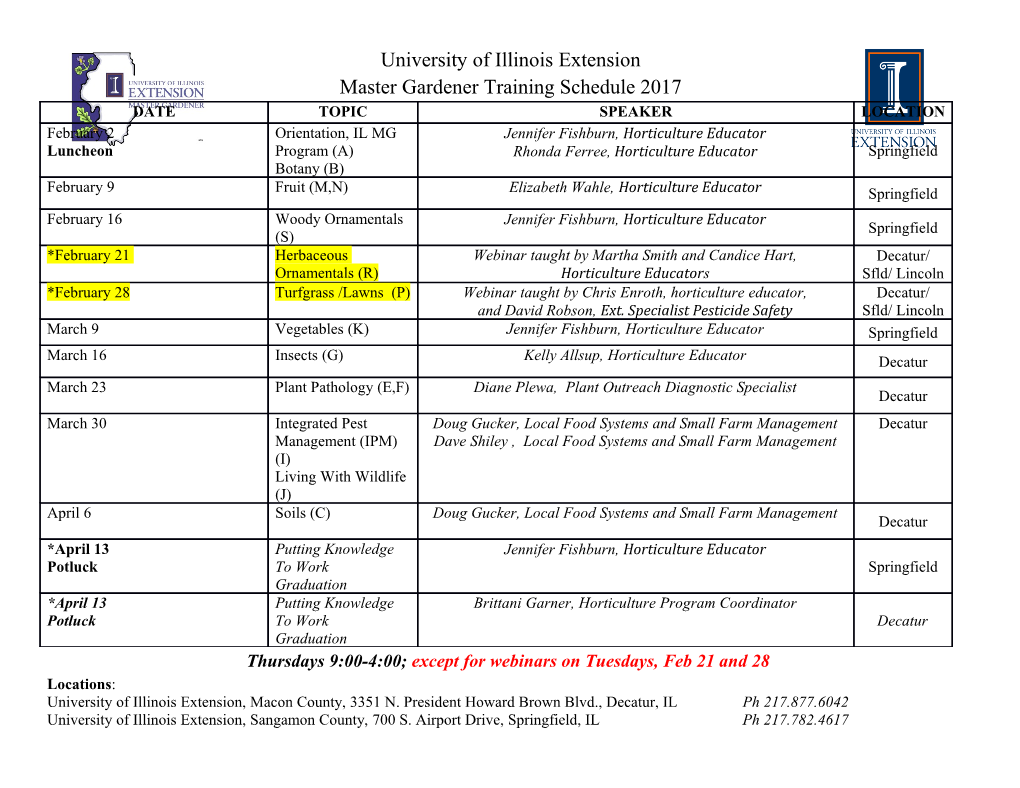
2012 IEEE International Conference on Robotics and Automation RiverCentre, Saint Paul, Minnesota, USA May 14-18, 2012 Wind field estimation for autonomous dynamic soaring Jack W. Langelaan John Spletzer, Corey Montella Joachim Grenestedt Aerospace Engineering, Penn State University Computer Science and Engineering Mechanical Engineering email: [email protected] Lehigh University Lehigh University Abstract— A method for distributed parameter estimation of uncertainty in the map must be computed to enable robust a previously unknown wind field is described. The application trajectory planning. is dynamic soaring for small unmanned air vehicles, which severely constrains available computing while simultaneously In [12] Lawrance and Sukkarieh describe a method for requiring updates that are fast compared with a typical dynamic wind field estimation based on Gaussian Process Regression. soaring cycle. A polynomial parameterization of the wind field Their approach has the benefit of being applicable to arbitrary is used, allowing implementation of a linear Kalman filter wind fields, but suffers from slow updates, making real-time for parameter estimation. Results of Monte Carlo simulations implementation problematic. In contrast to that work, here show the effectiveness of the approach. In addition, in-flight measurements of wind speeds are compared with data obtained we seek to develop a method for wind field estimation that from video tracking of balloon launches to assess the accuracy uses known structure to simplify estimation. of wind field estimates obtained using commercial autopilot During dynamic soaring, the cyclic trajectory implies that modules. the volume of air “inhabited” by the vehicle is limited in size, I. INTRODUCTION with dimensions dependent on the trajectory parameters. For soaring in the near-ground shear layer the vertical dimension Dynamic soaring of birds was first described by Lord is of order 10–100m with similar scales for the cross- Rayleigh in 1883 [1] and discussed in more detail in 1889 wind and downwind dimensions. The preliminary results [2]. While it seems to be a much more recent idea, the described above for jet stream dynamic soaring show that potential for dynamic soaring of aircraft has actually been a volume approximately 1 km × 1 km × 1 km is required. recognized since at least 1909 [3], and dynamic soaring In addition, dynamic soaring (especially that employed by of full sized aircraft has been studied at several times albatrosses and human RC glider pilots) typically exploits over the past century [4], [5], [6]. With the advent of low wind fields with fairly simple structure. This wind field cost electronics and the first demonstrations of radio-control consists of a mean spatially varying component with tem- dynamic soaring, the potential for long duration flight by porally varying terms (i.e. gusts) superimposed. Generally unmanned aerial vehicles (UAVs) has recently become an the vertical gradient is significantly stronger than horizontal area of research [7]. The possibility of dynamic soaring in gradients, and the rate of change of the mean wind field is the shear layer near the jet stream is discussed by Sachs and fairly slow compared with the time required to fly a single da Costa [8]. cycle. Combined with the relatively limited volume occupied An overview of the instrumentation required for full scale during dynamic soaring, this structure can be exploited to dynamic soaring is discussed by Kiceniuk [9]. Detailed simplify the mapping problem, making it tractable for real- results of dynamic soaring flight tests conducted at the time implementation. The estimation problem is thus cast as Dryden Flight Research Center are described by Gordon a parameter estimation problem, where a smooth function [10]. is used to model the wind field and the parameters of Typically dynamic soaring is cast as a trajectory optimiza- this function are estimated using measurements obtained at tion problem with an a priori known wind field [11]. Un- discrete, distributed points. fortunately this will not be available during flight; moreover, to the authors’ knowledge there are currently no sensors that Since in-flight wind field estimation relies on in situ can be carried on a small UAV that can measure the 3D measurements of wind speed, we also briefly discuss wind wind field ahead of the vehicle. A means of mapping or velocity measurements. Results of in-flight measurements predicting the wind field using only on-board measurements are compared with measurements of wind velocity collected is therefore required. There are two components to wind using vision-based tracking of balloons. While time- and mapping: first, estimating wind velocity given measurements space-synchronization of balloon and aircraft data is prob- aboard the vehicle; second, incorporating these estimates in lematic, we show that the aircraft can obtain reasonable a three-dimensional map of the mean wind field. measurements of wind velocity in-flight. Since the purpose of the wind map is to enable real-time The remainder of this paper describes wind field estima- trajectory planning it must satisfy several requirements, in- tion (Section II), presents results of Monte Carlo simulations cluding: (a) it must be updated in real time; (b) the trajectory (Section III), describes results of a hardware experiment planner must be able to query the map very quickly; and (c) (Section IV) and presents concluding remarks (Section V). 978-1-4673-1405-3/12/$31.00 ©2012 IEEE 16 II. WIND FIELD ESTIMATION the uncertainty in computed wind magnitude ranges from The problem at hand is to estimate a continuous, smooth σ = 1:26 m=s to σ = 3:71 m=s. function that captures the spatial variation of the mean wind B. Wind field estimation as distributed parameter estimation field. The mean wind field is assumed to vary slowly with 1) Wind field parameterization: If DS is limited to only time: here slowly means that several DS cycles can be flown the boundary layer (so that only albatross-style DS is per- before significant change in mean wind speed occurs. formed) then one can impose empirically-derived functions Note that direct measurements of wind velocity are not such as Prandtl’s power law relationship [14]: available. Rather, these must be computed in-flight based on data measured by an autopilot module onboard the aircraft. u h1=7 ≈ (6) The wind field is then estimated using the computed wind U δ velocities. where u is the wind speed, U is the free stream velocity, A. Wind velocity computation h is height above the surface and δ is the boundary layer A method for computing wind velocity and an associated thickness. uncertainty analysis using sensors typically available in an In this case a first order polynomial function in ln h autopilot module (e.g. GPS position and velocity, air speed, can perfectly model the wind field. However, such specific rate gyros, accelerometers) is given in [13]. For convenience parameterizations can lead to inaccurate representations if results are summarized here. they are inappropriately applied. A more generically ap- Components of the wind field can be computed from plicable parameterization is desirable: here a polynomial ground-relative velocity (available from GPS) and air speed: representation is used. 2) Polynomial representation of wind fields: For conve- wix =x _ − va cos γ cos β cos nience the wind field is represented by three components: +va cos γ sin β sin (1) horizontal magnitude, horizontal direction, and vertical com- wiy =y _ − va cos γ cos β sin ponent. Each component is assumed to vary independently. −va cos γ sin β cos (2) N X ~N−i wiz =z _ − va sin γ (3) w(h) = aih (7) i=0 Here w ; w ; w are xyz components of the wind vec- ix iy iz N tor expressed in the inertial frame, x;_ y;_ z_ are components of X φ (h) = b h~N−i (8) aircraft velocity with respect to the inertial frame (obtained w i i=0 from GPS), va is airspeed, γ is flight path angle with respect N to the air, β is sideslip angle, and is heading. It is assumed X w (h) = c h~N−i that the autopilot module provides estimates of angles. z i (9) For zero-mean Gaussian measurement noise the uncer- i=0 ~ h−h0 tainty in computed wind field can be computed by linearizing where h = ∆h is altitude shifted to a reference datum the above equations about the current flight condition so that and non-dimensionalized by a scale factor. Dynamic soaring typically exploits spatial (especially vertical) gradient in w ≈ g(z¯) + G(z − z¯) (4) magnitude, hence for compactness equations here will be developed only for vertical variation in magnitude. where w = [w w w ]T , z = [x _ y_ z_ v γ β ]T and ix iy iz a The task of the estimator is thus to compute estimates a^ of G = rg, the Jacobian of the wind computation equations i the polynomial parameters defining the wind field. Clearly (Equation 1, 2, 3) with respect to the measurements z. Then winds change with time as well as in space. The problem Σ = GΣ GT , where Σ is the noise covariance associated w z z of wind parameter estimation is thus a dynamic estimation with ground speed, orientation and air data measurements. problem, and as formulated it is a linear parameter estimation As described in [13], a measure of the uncertainty in problem, well suited to implementation as a Kalman filter. horizontal magnitude is Rw = Σw(1; 1) + Σw(2; 2): 2 2 2 2 C. Kalman Filter Design Rw = σx_ + σy_ + σv cos γ a Without an accurate model for the time rate of change of +σ2v2 sin2 γ + (σ2 + σ2 )v2 cos2 γ (5) γ a β a wind field, wind velocity is assumed to vary as a random This immediately shows that a significant contributor to walk, so that uncertainty in computed wind field is the vehicle’s airspeed ak+1 = ak + n (10) (through coupling with orientation uncertainty).
Details
-
File Typepdf
-
Upload Time-
-
Content LanguagesEnglish
-
Upload UserAnonymous/Not logged-in
-
File Pages7 Page
-
File Size-