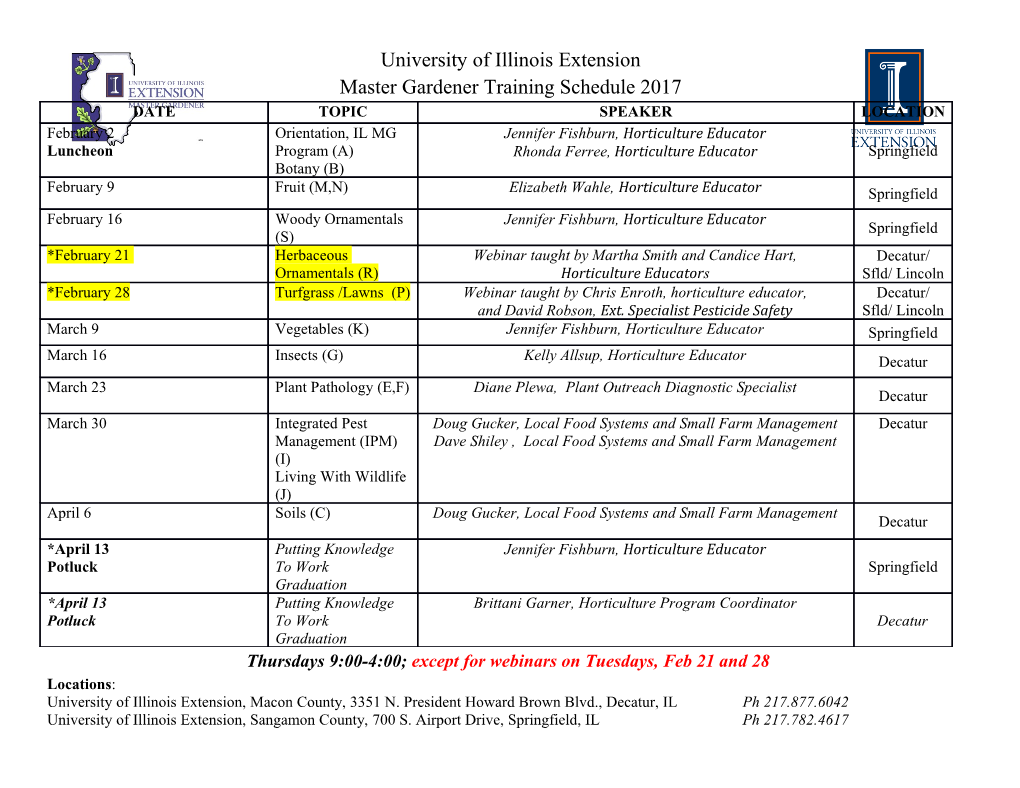
TREATMENT AND SPILLOVER EFFECTS UNDER NETWORK INTERFERENCE Michael P. Leung* Abstract—We study nonparametric and regression estimators of treatment within cluster and identifies the spillover effect using varia- and spillover effects when interference is mediated by a network. Infer- tion in treatment saturation across clusters. ence is nonstandard due to dependence induced by treatment spillovers and network-correlated effects. We derive restrictions on the network degree Partial interference is a special case of network interference distribution under which the estimators are consistent and asymptotically because we can define a block-diagonal network adjacency normal and show they can be verified under a strategic model of network matrix that captures the interference structure by connecting formation. We also construct consistent variance estimators robust to het- eroskedasticity and network dependence. Our results allow for the estima- two units if and only if they are members of the same cluster. tion of spillover effects using data from only a single, possibly sampled, In this paper, we study a general network interference setting network. in which the network need not be block-diagonal. This is important in settings where interference is mediated through a social network. For example, Cai et al. (2015) and Oster I. Introduction and Thornton (2012) study the diffusion of product adop- vast literature in econometrics and statistics studies in- tion through social networks by randomizing knowledge of, Aference on treatment effects. A fundamental assumption or access to, the product across units. To separately identify in this literature is the stable unit treatment value assumption treatment and spillover effects, we can consider subpopula- (SUTVA), which states that the ego’s treatment response is tions of units with the same degree, or number of network invariant to the treatment assignment of any alter. In other neighbors. In such subpopulations, variation in the number words, there are no treatment “spillovers.” However, there of treated neighbors identifies the spillover effect, and varia- are many important contexts in which this assumption fails tion in the ego’s treatment assignment identifies the treatment to hold, and the measurement of spillover effects is often of effect. inherent interest. This paper studies inference on treatment Whereas inference in the partial interference setting is stan- and spillover effects in the absence of SUTVA. We assume dard using conventional clustered standard errors, inference spillovers are mediated through a network, which may be par- with network interference is challenging because a social net- tially observed by the econometrician, and that treatments are work often cannot be partitioned into a large set of plausi- randomly assigned. This setting is relevant for a wide vari- bly independent clusters. For example, Miguel and Kremer ety of experimental contexts, including those in development (2004) consider a setting with a single network where units economics (Bhattacharya, Dupas, & Kanaya, 2013; Duflo, are students, students are treated if they underwent deworm- Dupas, & Kremer, 2011; Miguel & Kremer, 2004), social ing, and students are connected if they study at the same economics (Kling, Liebman, & Katz, 2007; Sobel, 2006), school or nearby schools. The authors are interested in the medical science (Christakis, 2004; Kim et al., 2015; Valente spillover effect of deworming on other students’ absenteeism. et al., 2007), and the study of online social networks (Bond While it appears natural to cluster standard errors at the school et al., 2012; Kramer, Guillory, & Hancock, 2014).1 level, this may not adequately account for the dependence To identify spillover effects in settings without network structure for two reasons. First, the outcome of student i de- data, researchers often employ two-stage randomization de- pends on Ni, the number of treated students in neighboring signs, where each cluster c is randomly assigned a treatment schools, due to the spillover effect. Consequently, the data are not i.i.d. across students because Ni is a function of the saturation sc ∈ [0, 1] and then each individual i in c is ran- domized into treatment with probability s (Baird et al., 2018; treatment status of any student j in a neighboring school. c ε Hudgens & Halloran, 2008). Under the partial interference Second, the error term i may exhibit network dependence. assumption of no spillovers across clusters, this design iden- The underlying causal mechanism behind absenteeism im- tifies the treatment effect using variation in treatment status plies that the error is a function of whether the student has a worm infection, and this is correlated between neighbors i and j. Clustering standard errors at the school level assumes Received for publication July 5, 2016. Revision accepted for publication students in neighboring schools are independent, which is January 11, 2019. Editor: Bryan S. Graham. violated in both of these scenarios. ∗Leung: University of Southern California. Todeal with network dependence, some studies simply col- I thank the editor and referees for comments that helped improve the lect data on many plausibly independent (usually geograph- exposition of the paper. This work benefited from research support by MIT IDSS. ically isolated) networks, but this can be costly in practice, A supplemental appendix is available online at http://www.mitpress since it requires a network survey in addition to a random- journals.org/doi/suppl/10.1162/rest_a_00818. ized control trial in each cluster. Other studies choose some 1Other papers in applied economics that estimate treatment spillovers in experimental settings include Bandiera, Barankay, and Rasul (2009), rule to partition the network into many subnetworks, which Bursztyn et al. (2014), Cai, De Janvry, and Sadoulet (2015), Duflo and Saez are assumed to be independent. For example, they may clus- (2003), Dupas (2014), Kim et al. (2015), and Oster and Thornton (2012). ter standard errors according to some observed groups, like The Review of Economics and Statistics, May 2020, 102(2): 368–380 © 2019 by the President and Fellows of Harvard College and the Massachusetts Institute of Technology https://doi.org/10.1162/rest_a_00818 Downloaded from http://www.mitpressjournals.org/doi/pdf/10.1162/rest_a_00818 by guest on 26 September 2021 TREATMENT AND SPILLOVER EFFECTS 369 schools or classrooms, or they may apply a community de- tunately, it is quite common in practice for the network to tection algorithm. However, as in the deworming example, be sampled and partially observed. We therefore consider a the independence assumption may not be credible, since it superpopulation model, allowing network data to be obtained ignores links that bridge these subnetworks. through a common snowball-sampling scheme. Nonetheless, We study nonparametric and regression estimators of the general central limit theorem we derive that underlies treatment and spillover effects, develop conditions under our main results can be applied to either the finite- or super- which they are consistent and asymptotically normal in the population case. single-network setting, and provide new variance estimators The econometrics literature predominantly focuses on robust to heteroskedasticity and network dependence. The asymptotic inference in the many-networks case, whereas practical relevance of these results is twofold. First, they ob- we consider the single, large network case. Manski (2013) viate the need to collect data on a large sample of plausi- and Lazzati (2015) study partial identification of treatment bly independent clusters or networks. Instead, only a single response under group interference. There is also a large lit- network is required, which substantially reduces the cost of erature on identifying and estimating peer effects, usually in network experiments. We illustrate this point in an empirical the specific context of the linear-in-means model (Bramoullé, application, qualitatively replicating the results of Cai et al. Djebbari, & Fortin, 2009; Brock & Durlauf, 2001; De Giorgi, (2015) but using data from the largest two villages in their Pellizzari, & Redaelli, 2010; Graham, 2008; Hirano & Hahn, sample, comprising 142 of the 1,225 available units. Second, 2010; Manski, 1993). the assumption that SUTVAholds across clusters in two-stage A growing literature in statistics studies estimation of treat- randomized designs may be unreasonable in settings where ment effects when SUTVA is violated (Basse & Airoldi, clusters are not sufficiently geographically isolated and cross- 2015; Hudgens & Halloran, 2008; Toulis & Kao, 2013). Most cluster links exist. Using our results, it is unnecessary to par- papers assume that the sample contains many independent tition the data into many independent clusters, as the entire clusters or networks. Aronow and Samii (2017), Liu and Hud- sample can be treated as a single network. gens (2014), Sofrygin and van der Laan (2015), and van der Our results exploit the fact that under restrictions on Laan (2014) discuss inference with a single large cluster or spillover effects widely used in practice and restrictions on network. Aronow and Samii (2017) do not explicitly impose network-correlated effects, a single network can hold a great a network structure and instead require high-level restrictions deal of independent information. For example in Miguel and on potential outcomes that ensure weak dependence. In con- Kremer (2004),
Details
-
File Typepdf
-
Upload Time-
-
Content LanguagesEnglish
-
Upload UserAnonymous/Not logged-in
-
File Pages13 Page
-
File Size-