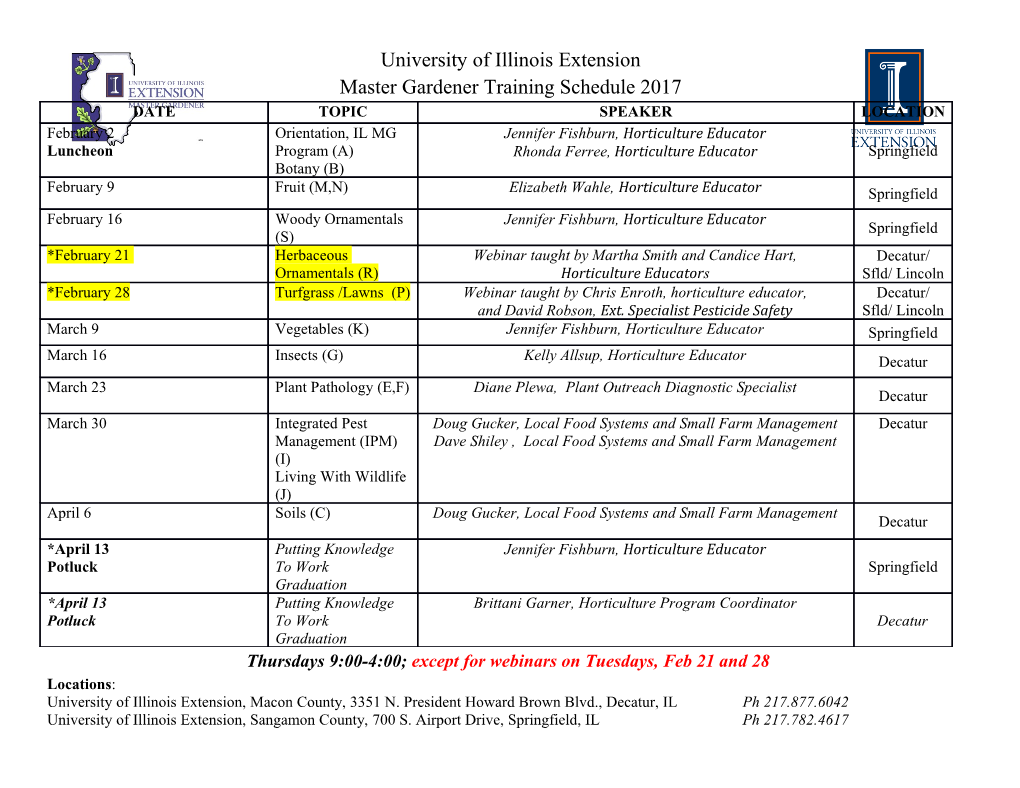
Electronic Supplementary Material (ESI) for Chemical Science This journal is © The Royal Society of Chemistry 2013 Thermodynamic and kinetic specificities of ligand binding Zhiqiang Yan, Xiliang Zheng, Erkang Wang and Jin Wang∗ Supporting information Derivation of initial atomic pairwise potentials. Here we simply introduce the main concept and basic steps of developing scoring function SPA, for detailed information, please see the paper [1]. The SPA (SPecificity and Affinity) is an optimized knowledge- based scoring function based on a set of initial atom-pair potentials. The initial potentials of SPA are directly derived from the Boltzmann relation widely used in the knowledge-based statistical potentials [2{5], which is − uk = KBT ln gk (1) where gk is the observed pair distribution function which can be calculated from the known structures of protein-ligand complexes in the training database. Optimization of potential energy function. To construct the optimizing potential energy function which aims to maximize the binding specificity and the consistence between predicted and experimental affinity, the initial energy function was rewritten by introducing a set of adjustable parameters as the coefficients ck for the potentials of atom pair, that is X E = ckfkuk (2) k where E is the total intermolecular energy of a protein-ligand complex. k stands for the type of atom pair interaction. fk represents the occurrences of the interaction type k. As discussed in main text, the ISR as quantified intrinsic specificity for a given protein-ligand complex m is calculated as δE λ = α (3) m ∆E where α is a scaling factor which accounts for the contribution of the entropy to the specificity [6, 7].q Here, it approximately depends on the number of torsional bonds of the ligands 1 α ∼ . δE is the energy gap between the energy of native conformation EN and the ntb average energy of ensemble of decoys < ED >, and ∆E is the energy fluctuation or the width of the energy distribution of the decoys. All the conformational decoys of protein- ligand complexes were generated by the molecular docking with software AutoDock4.2 [8]. 1 Electronic Supplementary Material (ESI) for Chemical Science This journal is © The Royal Society of Chemistry 2013 Combined with equations 2, the λ can be represented as m P j N − j P P α k ckuk(fk < fk >) λm = − (4) k l ckclukul(< fkfl > < fk >< fl >) where k,l are the indices of the interaction types. Once fk is computed for each interaction type in the decoys, one can readily compute the value of λm for a given set of ck. We need a single objective function that reflects the λm values for all the protein-ligand complexes in the training set. We chose the Bolzmann-like weighted average of λm as the objective function which is P λ exp (β λ ) λ = Pm m λ m (5) m exp (βλλm) where βλ is a constant value for weighting which is set as −0:1. In addition to the ISR as the quantification of specificity, the quantitative measurements of the correlation between predicted and experimental affinity is depicted with Pearson's correlation coefficient by P (Ep − < Ep >)(Ee − < Ee >) γ = pP m m m pPm m (6) p − p 2 e − e 2 m(Em < Em >) m(Em < Em >) p The predicted binding affinity Em for the protein-ligand complex is represented by the binding scores calculated from our scoring function with a given set of ck. The experi- e mentally measured affinity Em is expressed in log Kd or log Ki units, where Kd and Ki are experimentally determined dissociation constant and inhibition constant respectively for the protein-ligand complex m. The aim of optimization is to maximize the value of λ for specificity and the value of γ for affinity, a combination parameter (ρ = λγ) which couples specificity and affinity is constructed to evaluate the performance of scoring function during the optimization. The optimization is performed by Monte Carlo search with simulated annealing in the space of adjustable coefficients ck. At each MC step one of the coefficients is chosen at random and updated. This resulting change in E (E is defined as E = −ρ, minimizing E is equivalent of maximizing ρ) is accepted with the probability P = min(1; exp (−βρ∆E)) (7) −1 −1 where βρ is the optimization temperature for ρ. The temperature βρ decreases exponen- tially during the search. The convergence of both λ and γ indicates the optimized scoring function reaches the maximal performance of simultaneously quantifying the specificity and affinity. 2 Electronic Supplementary Material (ESI) for Chemical Science This journal is © The Royal Society of Chemistry 2013 Performance of SPA. The straightforward way to evaluate a novel scoring func- tion is to make a comparison with other existing scoring function on their performances. SPA was tested on a benchmark of protein-ligand complexes which is a high-quality set of protein-ligand complexes selected out from the refined set of 2007 version of the PDBbind database [9]. This benchmark was taken as testing set to compare the performance for a large collection of 16 scoring functions implemented in main-stream commercial softwares or available from academic research groups, which offers a reference for the performance of SPA. It outperformed other 16 scoring functions on both predictions of binding pose and binding affinity [1], suggesting SPA is not only capable of discriminating the specific \native" conformation out of a large number of decoys by their scores but also accurately predicting the binding affinities of different protein-ligand complexes. This result is encouraging and motivating to apply SPA in the virtual screening to identify the lead compounds for drug discovery. Statistics of discrimination. To quantify the discriminations of specific receptor target COX-2 from the competitive target COX-1 when binding with the selective drugs of COX-2, as well as the differences between selective and non-selective drugs of COX-2, the statistical method Kolmogorov-Smirnov test (KS test) was employed. The KS test quantifies a distance between two samples and determine if these two datasets differ significantly. The KS statistic is calculated as D = maxj(C1(x) − C2(x)j (8) where C1(x) and C2(x) are two cumulative distribution functions for compared datasets at cutoff x of a specific parameter X. In our work, the KS statistic were calculated for two kinds of comparisons; 1. the comparison between COX-2 and COX-1 with selective drugs, 2. the comparison between selective drugs and non-selective drugs with COX-2. The parameter X for the KS test can be set as affinity (E), ISR, residence time (RT) or the combination of them. The combinations of two parameters (E+ISR, or E+RT) or all three parameters (E+ISR+RT) were performed using logistic regression. The logistic regression is a type of regression analysis for predicting a dichotomous outcome, e.g. \Yes" vs. \No". For the first comparison, the selective drugs binding to COX-2 were set as 1 while the selective drugs binding to COX-1 were set as 0. For the second comparison, the selective drugs binding with COX-2 were set as 1 while the non-selective drugs binding with COX-2 were set as 0. The independent variables were linearly combined as one parameter by taking the regression 3 Electronic Supplementary Material (ESI) for Chemical Science This journal is © The Royal Society of Chemistry 2013 coefficients. The regressions were conducted with the package of R. [1] Z. Yan and J. Wang, Scientific reports 2 (2012). [2] M. J. Sippl, Journal of computer-aided molecular design 7, 473 (1993). [3] P. D. Thomas and K. A. Dill, Journal of molecular biology 257, 457 (1996). [4] H. Zhou and Y. Zhou, Protein Science 11, 2714 (2009). [5] S.-Y. Huang and X. Zou, Journal of computational chemistry 27, 1866 (2006). [6] J. Wang and G. M. Verkhivker, Physical review letters 90, 188101 (2003). [7] J. Wang, X. Zheng, Y. Yang, D. Drueckhammer, W. Yang, G. Verkhivker, and E. Wang, Physical review letters 99, 198101 (2007). [8] G. M. Morris, D. S. Goodsell, R. S. Halliday, R. Huey, W. E. Hart, R. K. Belew, and A. J. Olson, Journal of computational chemistry 19, 1639 (1998). [9] T. Cheng, X. Li, Y. Li, Z. Liu, and R. Wang, Journal of chemical information and modeling 49, 1079 (2009). 4 Electronic Supplementary Material (ESI) for Chemical Science This journal is © The Royal Society of Chemistry 2013 Table S1 30 selective (bold) and 20 non-selective nonsteroidal anti-inflammatory drugs (NSAIDs) of COX-2. Selective drugs (bold) are specific to inhibit COX-2, while non-selective drugs inhibit both the COX-2 and its isoenzyme COX-1. The predicted affinity (Epred (kcal/mol)), ISR and residence time (RT pred) are shown for the drugs. The known half maximal inhibitory concentrations (IC50 (uM)) and corresponding affinities (Eexp (kcal/mol)) for 20 drugs and experimentally determined half life (=0.693*residence time, RT exp (hr)) for 22 drugs are also listed. Drugs IC50 Eexp Epred ISR RT pred RT exp ns-398 0.47 -8.686 -8.273 2.638 1326.734 N/A l-745337 9.67 -6.884 -9.031 2.567 861.314 N/A celecoxib 0.87 -8.319 -10.181 3.148 3111.631 11.2 rofecoxib 0.53 -8.615 -9.662 3.508 2171.419 17.0 dup-697 0.06 -9.913 -10.864 3.341 4406.395 292.0 jte-522 0.085 -9.706 -9.936 3.823 2701.849 N/A valdecoxib 0.87 -8.319 -9.420 2.727 2146.821 9.5 etoricoxib 1.10 -8.179 -9.511 3.039 1635.223 22.0 meloxicam 0.70 -8.449 -8.991 3.301 3608.877 17.5 etodolac 3.70 -7.456 -7.566 2.332 497.923 7.3 l-776967 0.03 -10.327 -9.379 3.192 2079.798 N/A flosulide 0.75 -8.408 -8.404 2.352 559.414 N/A sulindac-sulfide 10.43 -6.838 -8.333 2.397 501.095 16.4 tolmetin 7.09 -7.069 -7.893 2.103 752.367 7.0 ketoprofen 1.08
Details
-
File Typepdf
-
Upload Time-
-
Content LanguagesEnglish
-
Upload UserAnonymous/Not logged-in
-
File Pages6 Page
-
File Size-