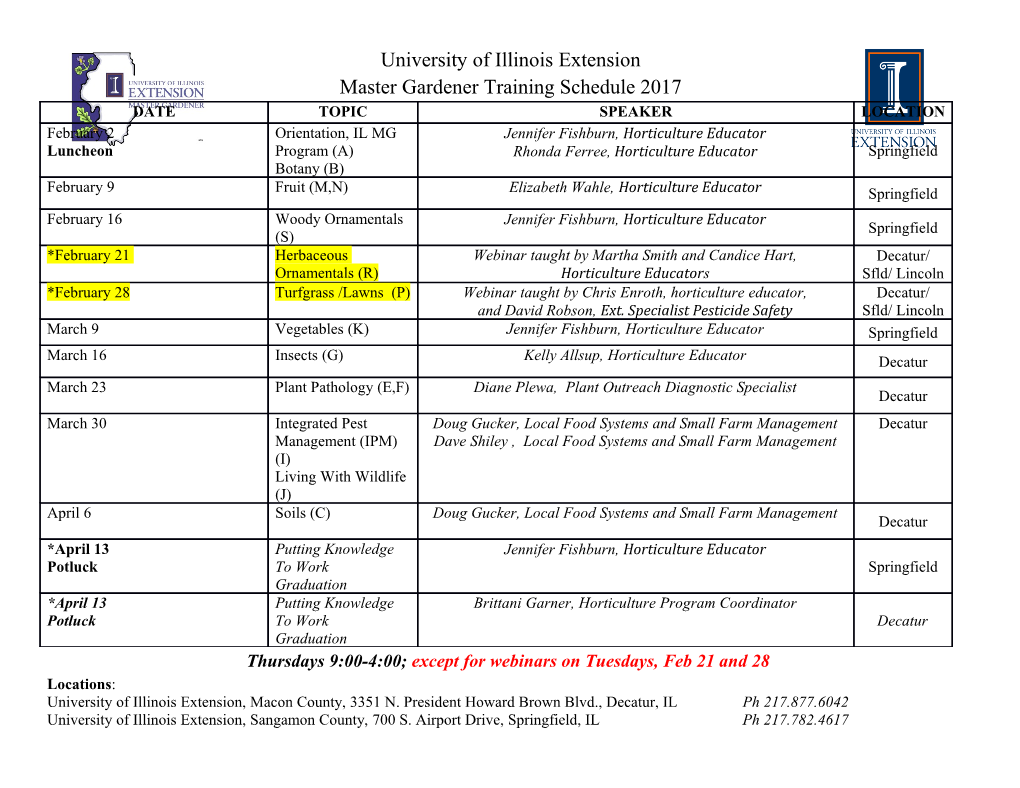
Interior-Point and Augmented Lagrangian Algorithms for Optimization and Control Stephen Wright University of Wisconsin-Madison May 2014 Wright (UW-Madison) Constrained Optimization May 2014 1 / 46 In This Section... My first talk was about optimization formulations, optimality conditions, and duality | for LP, QP, LCP, and nonlinear optimization. This section will review some algorithms, in particular: Primal-dual interior-point (PDIP) methods Augmented Lagrangian (AL) methods. Both are useful in control applications. We'll say something about PDIP methods for model-predictive control, and how they exploit the structure in that problem. Wright (UW-Madison) Constrained Optimization May 2014 2 / 46 Recapping Gradient Methods Considering unconstrained minimization min f (x) where f is smooth and convex, or the constrained version in which x is restricted to the set Ω, usually closed and convex. First-order or gradient methods take steps of the form xk+1 = xk − αk gk ; where αk 2 R+ is a steplength and gk is a search direction. dk is constructed from knowledge of the gradient rf (x) at the current iterate x = xk and possibly previous iterates xk−1; xk−2;::: . Can extend to nonsmooth f by using the subgradient @f (x). Extend to constrained minimization by projecting the search line onto the convex set Ω, or (similarly) minimizing a linear approximation to f over Ω. Wright (UW-Madison) Constrained Optimization May 2014 3 / 46 Prox Interpretation of Line Search Can view the gradient method step xk+1 = xk − αk gk as the minimization of a first-order model of f plus a \prox-term" which prevents the step from being too long: T 1 2 xk+1 = arg min f (xk ) + gk (x − xk ) + kx − xk k2: x 2αk Taking the gradient the quadratic and setting to zero, we obtain 1 gk + (xk+1 − xk ) = 0; αk which gives the formula for xk+1. This viewpoint is the key to several extensions. Wright (UW-Madison) Constrained Optimization May 2014 4 / 46 Extensions: Constraints When a constraint set Ω is present we can simply minimize the quadratic model function over Ω: T 1 2 xk+1 = arg min f (xk ) + gk (x − xk ) + kx − xk k2: x2Ω 2αk Gradient Projection has this form. We can replace the `2-norm measure of distance with some other measure φ(x; xk ): T 1 xk+1 = arg min f (xk ) + gk (x − xk ) + φ(x; xk ): x2Ω 2αk Could choose φ to \match" Ω. For example, a measure derived from the entropy function is a good match for the simplex Ω := fx j x ≥ 0; eT x = 1g: Wright (UW-Madison) Constrained Optimization May 2014 5 / 46 Extensions: Regularizers In many modern applications of optimization, f has the form f (x) = l(x) + τ (x): |{z} | {z } smooth function simple nonsmooth function Can extend the prox approach above by choosing gk to contain gradient information from l(x) only; including τ (x) explicitly in the subproblem. Subproblems are thus: T 1 2 xk+1 = arg min l(xk ) + gk (x − xk ) + kx − xk k + τ (x): x 2αk Wright (UW-Madison) Constrained Optimization May 2014 6 / 46 Extensions: Explicit Trust Regions Rather than penalizing distance moved from current xk , we can enforce an explicit constraint: a trust region. T xk+1 = arg min f (xk ) + g (x − xk ) + Ikx−x k≤∆ (x); x k k k where IΛ(x) denotes an indicator function with ( 0 if x 2 Λ IΛ(x) = 1 otherwise: Adjust trust-region radius ∆k to ensure progress e.g. descent in f . Wright (UW-Madison) Constrained Optimization May 2014 7 / 46 Extension: Proximal Point Could use the original f in the subproblem rather than a simpler model function: 1 2 xk+1 = arg min f (x) + kx − xk k2: x 2αk although the subproblem seems \just as hard" to solve as the original, the prox-term may make it easier by introducing strong convexity, and may stabilize progress. Can extend to constrained and regularized cases also. Wright (UW-Madison) Constrained Optimization May 2014 8 / 46 Quadratic Models: Newton's Method We can extend the iterative strategy further by adding a quadratic term to the model, instead of (or in addition to) the simple prox-term above. Taylor's Theorem suggests basing this term on the Hessian (second-derivative) matrix. That is, obtain the step from T 1 T 2 xk+1 := arg min f (xk ) + rf (xk ) (x − xk ) + (x − xk ) r f (xk )(x − xk ): x 2 Can reformulate to solve for the step dk : Then have xk+1 = xk + dk , where T 1 T 2 dk := arg min f (xk ) + rf (xk ) d + d r f (xk )d: d 2 2 See immediately that this model won't have a bounded solution if r f (xk ) is not positive definite. It usually is positive definite near a strict local solution x∗, but need something that works more globally. Wright (UW-Madison) Constrained Optimization May 2014 9 / 46 Practical Newton Method One \obvious" strategy is to add the prox-term to the quadratic model: T 1 T 2 1 dk := arg min f (xk ) + rf (xk ) d + d r f (xk ) + I d; d 2 αk choosing αk so that The quadratic term is positive definite; Some other desirable property holds, e.g. descent f (xk + dk ) < f (xk ). We can also impose the trust-region explicitly: T 1 T 2 dk := arg min f (xk ) + rf (xk ) d + d r f (xk )d + Ikdk≤∆ (x); d 2 k or alternatively: T 1 T 2 dk := arg min f (xk ) + rf (xk ) d + d r f (xk )d: d : kdk≤∆k 2 But this is equivalent: For any ∆k , there exists αk such that the solutions of the prox form and the trust-region form are identical. Wright (UW-Madison) Constrained Optimization May 2014 10 / 46 Quasi-Newton Methods Another disadvantage of Newton is that the Hessian may be difficult to evaluate or otherwise work with. The quadratic model is still useful when we use first-order information to learn about the Hessian. Key observation (from Taylor's theorem): the secant condition: 2 r f (xk )(xk+1 − xk ) ≈ rf (xk+1) − rf (xk ): The difference of gradients tells us how the Hessian behaves along the direction xk+1 − xk . By aggregating such information over multiple steps, we can build up an approximation to the Hessian than is valid along multiple directions. 2 Quasi-Newton Methods maintain an approximation Bk to r f (xk ) that respects the secant condition. The approximation may be implicit rather than explicit, and we may store an approximation to the inverse Hessian instead. Wright (UW-Madison) Constrained Optimization May 2014 11 / 46 L-BFGS A particularly populat quasi-Newton method, suitable for large-scale problems is the limited-memory BFGS method (L-BFGS) which stores the Hessian or inverse Hessian approximation implicitly. L-BFGS stores the last 5 − 10 update pairs: sj := xj+1 − xj ; yj := rf (xj+1) − rf (xj ): for j = k; k − 1; k − 2;:::; k − m. Can implicitly construct Hk+1 that satisfies Hk+1yj = sj : In fact, an efficient recursive formula is available for evaluating dk+1 := −Hk+1rf (xk+1) | the next search direction | directly from the (sj ; yj ) pairs and from some initial estimate of the form (1/αk+1)I . Wright (UW-Madison) Constrained Optimization May 2014 12 / 46 Newton for Nonlinear Equations There is also a variant of Newton's method for nonlinear equations: Find x such that F (x) = 0; n n where F : R ! R (n equations in n unknowns.) Newton's method forms a linear approximation to this system, based on another variant of Taylor's Theorem which says Z 1 F (x + d) = F (x) + J(x)d + [J(x + td) − J(x)]d dt; 0 where J(x) is the Jacobian matrix of first partial derivatives: 2 @F1 ··· @F1 3 @x1 @xn 6 . 7 J(x) = 4 . 5 (usually not symmetric). @Fn ··· @Fn @x1 @xn Wright (UW-Madison) Constrained Optimization May 2014 13 / 46 When F is continuously differentiable, we have F (xk + d) ≈ F (xk ) + J(xk )d; so the Newton step is the one that make the right-hand side zero: −1 dk := −J(xk ) F (xk ): The basis Newton method takes steps xk+1 := xk + dk . Its effectiveness can be improved by Doing a line search: xk+1 := xk + αk dk , for some αk > 0; −1 Levenberg strategy: add λI to J and set dk := −(J(xk ) + λI ) F (xk ). Guide progress via a merit function, usually 1 φ(x) := kF (x)k2: 2 2 Achtung! Can get stuck in a local min of φ that's not a solution of F (x) = 0. Wright (UW-Madison) Constrained Optimization May 2014 14 / 46 Homotopy Tries to avoid the local-min issue with the merit function. Start with an \easier" set of nonlinear equations, and gradually deform it to the system F (x) = 0, tracking changes to the solution as you go. F (x; λ) := λF (x) + (1 − λ)F0(x); λ 2 [0; 1]: Assume that F (x; 0) = F0(x) = 0 has solution x0. Homotopy methods trace the curve of solutions (x; λ) until λ = 1 is reached. The corresponding value of x then solves the original problem. Many variants. Some supporting theory. Typically more expensive than enhanced Newton methods, but better at finding solutions to F (x) = 0. We mention homotopy mostly because of its connection to interior-point methods. Wright (UW-Madison) Constrained Optimization May 2014 15 / 46 Interior-Point Methods n Recall the monotone LCP: Find z 2 R such that 0 ≤ z ? Mz + q ≥ 0; n×n n where M 2 R is positive semidefinite, and q 2 R .
Details
-
File Typepdf
-
Upload Time-
-
Content LanguagesEnglish
-
Upload UserAnonymous/Not logged-in
-
File Pages47 Page
-
File Size-