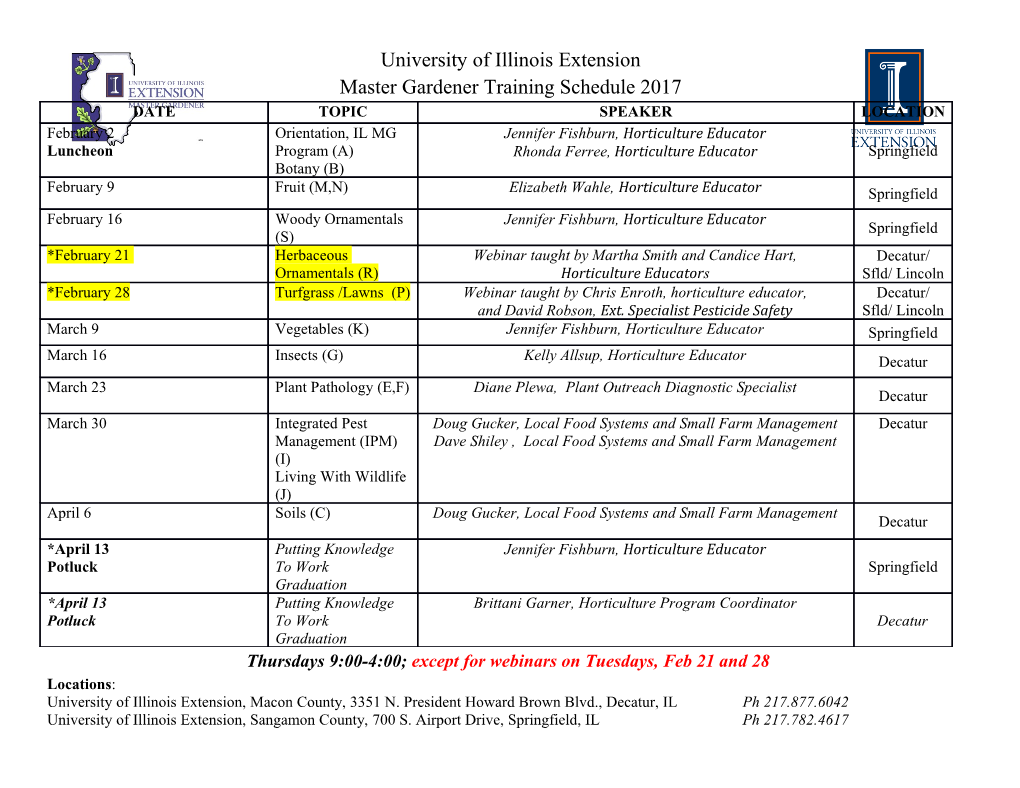
DISSERTATION submitted to the Combined Faculty of Natural Sciences and Mathematics of the Ruprecht–Karls University Heidelberg for the degree of Doctor of Natural Sciences put forward by M.Sc. Felix Zahn born in Mannheim, Baden-Württemberg Heidelberg, 2020 Energy-Efficient Interconnection Networks for High-Performance Computing Advisor: Professor Dr. Holger Fröning Date of oral exam: ........................... Abstract In recent years, energy has become one of the most important factors for de- signing and operating large scale computing systems. This is particularly true in high-performance computing, where systems often consist of thousands of nodes. Especially after the end of Dennard’s scaling, the demand for energy- proportionality in components, where energy is depending linearly on utilization, increases continuously. As the main contributor to the overall power consump- tion, processors have received the main attention so far. The increasing energy proportionality of processors, however, shifts the focus to other components such as interconnection networks. Their share of the overall power consumption is expected to increase to 20% or more while other components further increase their efficiency in the near future. Hence, it is crucial to improve energy propor- tionality in interconnection networks likewise to reduce overall power and energy consumption. To facilitate these attempts, this work provides comprehensive studies about energy saving in interconnection networks at different levels. First, interconnection networks differ fundamentally from other components in their underlying technology. To gain a deeper understanding of these differences and to identify targets for energy savings, this work provides a detailed power analysis of current network hardware. Furthermore, various applications at different scales are analyzed regarding their communication patterns and locality properties. The findings show that communication makes up only a small fraction of the execution time and networks are actually idling most of the time. Another observation is that point-to-point communication often only occurs within various small subsets of all participants, which indicates that a coordinated mapping could further decrease network traffic. Based on these studies, three different energy-saving policies are designed, which all differ in their implementation and focus. Then, these policies are v evaluated in an event-based, power-aware network simulator. While two policies that operate completely local at link level, enable significant energy savings of more than 90% in most analyses, the hybrid one does not provide further benefits despite significant additional design effort. Additionally, these studies include network design parameters, such as transition time between different link configurations, as well as the three most common topologies in supercomputing systems. The final part of this work addresses the interactions of congestion manage- ment and energy-saving policies. Although both network management strategies aim for different goals and use opposite approaches, they complement each other and can increase energy efficiency in all studies as well as improve the performance overhead as opposed to plain energy saving. Zusammenfassung In den letzten Jahren ist Energie zu einem der wichtigsten Faktoren für En- twurf und Betreiben großer Rechensysteme geworden. Dies gilt insbesondere für das Hochleistungsrechnen, wo Systeme oft aus Tausenden von einzelnen Rechenknoten bestehen. Besonders nach dem Ende des Dennard Scaling steigt der Bedarf an energieproportionalen Komponenten, bei denen die Energie lin- ear von der Auslastung abhängt, kontinuierlich an. Als hauptverantwortliche Komponente für den Gesamtstromverbrauch haben die Prozessoren bisher die größte Aufmerksamkeit erhalten. Die zunehmende Energieproportionalität von Prozessoren verlagert jedoch den Schwerpunkt auf andere Komponenten wie zum Beispiel Verbindungsnetzwerke. Es wird erwartet, dass ihr Anteil am Gesamt- stromverbrauch auf 20% oder mehr ansteigt, während andere Komponenten in naher Zukunft ihre Effizienz weiter steigern werden. Daher ist es von entschei- dender Bedeutung, die Energieproportionalität in Verbindungsnetzwerken zu verbessern, um den Gesamtleistungs- und Energieverbrauch ebenfalls zu senken. Diese Arbeit trägt zu dieser Aufgabe bei, indem sie Energieeinsparungen in Verbindungsnetzwerken auf verschiedenen Ebenen umfassend analysiert. Verbindungsnetzwerke unterscheiden sich in ihrer zugrunde liegenden Tech- nologie elementar von anderen Komponenten. Um ein tieferes Verständnis dieser Unterschiede zu gewinnen und Ziele für Energieeinsparungen zu identifizieren, bietet diese Arbeit eine detaillierte Leistungsanalyse der aktuellen Netzwerk- Hardware. Darüber hinaus werden verschiedene Anwendungen unterschiedlicher Skalierung hinsichtlich ihrer Kommunikationsmuster und Loakalitätseigen- schaften analysiert. Die Ergebnisse zeigen, dass die Kommunikation nur einen kleinen Bruchteil der Ausführungszeit ausmacht und die Netzwerke die meiste Zeit tatsächlich ungenutzt bleiben. Eine weitere Beobachtung ist, dass Ende- zu-Ende-Kommunikation oft nur innerhalb verschiedener kleiner Teilmengen vii aller Teilnehmer stattfindet, was darauf hindeutet, dass ein maßgeschneidertes Zuordnen von Prozessen auf physikalische Rechenkerne die Netzwerkauslastung weiter verringern könnte. Auf der Grundlage dieser Studien werden drei verschiedene Energiesparstrate- gien entworfen, die sich alle in ihrer Umsetzung und ihrem Schwerpunkt unter- scheiden. Diese Strategien werden dann in einem ereignisbasierten Netzwerksim- ulator mit integriertem Energiemodell evaluiert. Während zwei Strategien, die vollständig lokal auf Linkebene arbeiten, in den meisten Analysen signifikante Energieeinsparungen von mehr als 90% ermöglichen, bietet die hybride Strategie trotz erheblichen zusätzlichen Designaufwands keine weiteren Vorteile. Darüber hinaus umfassen diese Studien Netzwerkdesignparameter, wie z.B. die Transi- tionzeit zwischen verschiedenen Linkkonfigurationen, sowie die drei häufigsten Topologien, die genutzt werden um Supercomputer zu entwerfen. Abschließend werden im letzten Teil der Arbeit Wechselwirkungen von Con- gestion Management und Energiesparmaßnahmen thematisiert. Obwohl beide Netzmanagementstrategien unterschiedliche Ziele verfolgen und entgegengesetzte Ansätze verwenden, ergänzen sie sich gegenseitig und können die Energieeffizienz in allen Studien erhöhen und Performanzindikatoren im Gegensatz den reinen Energiesparstrategien verbessern. Table of contents 1 Introduction1 2 Background: Interconnection Networks9 2.1 Switch Level Architecture . 11 2.1.1 Data Transmission . 11 2.1.2 Network Interface . 12 2.1.3 Links . 12 2.1.4 Switches . 14 2.1.5 Message Switching . 15 2.2 System Level Network Design . 18 2.2.1 Topologies . 19 2.2.2 Routing . 24 2.3 Message Passing Interface . 29 3 Energy Proportionality in Interconnection Networks 31 3.1 Power Consumtion . 32 3.1.1 CMOS . 33 3.1.2 CML . 35 3.2 Switch Core Power . 36 3.2.1 Frequency Scaling . 37 3.2.2 Radix Scaling . 38 3.3 Link Power . 40 3.3.1 Design . 41 3.3.2 Power Scaling . 41 3.4 Optical Links . 44 3.4.1 Overview . 44 3.4.2 Limitations . 44 4 Application Analyses 47 4.1 SONAR . 48 4.1.1 Metrics . 48 4.1.2 Concept . 50 4.2 Locality and Selectivity in Exascale Proxy Miniapps . 51 4.2.1 Metrics . 52 4.2.2 Methodology . 56 4.2.3 Hardware Parameters . 57 4.2.4 Results . 60 5 Simulation Tools 73 5.1 Network Simulator . 73 5.1.1 SAURON Simulator . 74 5.2 Energy-Aware Simulations . 78 5.2.1 Energy Features . 79 5.2.2 Traffic Pattern . 80 5.3 MPI Traces . 81 5.3.1 DUMPI Traces . 82 5.3.2 VEF Traces . 83 6 Energy Saving in Interconnection Networks 87 6.1 Approach . 88 6.1.1 Energy Saving Management . 88 6.1.2 Power State Granularity . 90 6.2 Energy Saving Policies . 92 6.2.1 On/Off . 92 6.2.2 High/Low . 96 6.2.3 Awake . 98 6.3 Evaluating Policies . 98 6.3.1 Applications . 99 6.3.2 Methodology . 101 6.3.3 Evaluation . 105 6.4 Combining Energy Saving Policies and Congestion Management 110 6.4.1 Congestion Management . 111 6.4.2 Methodology . 112 6.4.3 Evaluation . 115 7 Discussion 125 7.1 Related Work . 125 7.2 Workload Analysis . 128 7.2.1 Locality and Selectivity . 128 7.2.2 Topology Effects . 129 7.2.3 Network Utilization . 130 7.3 Energy Savings . 131 7.3.1 Policies . 131 7.3.2 Energy-Saving Parameters . 132 7.3.3 Topologies . 133 7.4 Congestion Management . 134 7.5 Outlook . 136 8 Conclusion 139 List of figures 145 List of tables 149 References 155 1 C h a p t e r Introduction Historically, the steady increase of computational power was driven by the decreasing size of integrated circuits and rising clock rates, described by Moore’s law. This performance increase was facilitated by Dennard’s scaling [1], which states that decreasing feature sizes also result in a proportional decrease in power consumption so that the power consumption per area remains constant. Additionally, pipelining and Instruction-Level Parallelism (ILP) ensured further performance scaling within a single Central Processing Unit (CPU). With mitigating clock rate growth in the 2000s, parallelism ensured further performance scaling. At about 2005, multi-core processors began to take over from single-core processors, which means multiple cores were integrated into one die. In order to take advantage of these parallel cores, software engineers followed this trend by shifting to Thread-Level Parallelism (TLP). While Moore’s law remains still valid,
Details
-
File Typepdf
-
Upload Time-
-
Content LanguagesEnglish
-
Upload UserAnonymous/Not logged-in
-
File Pages179 Page
-
File Size-