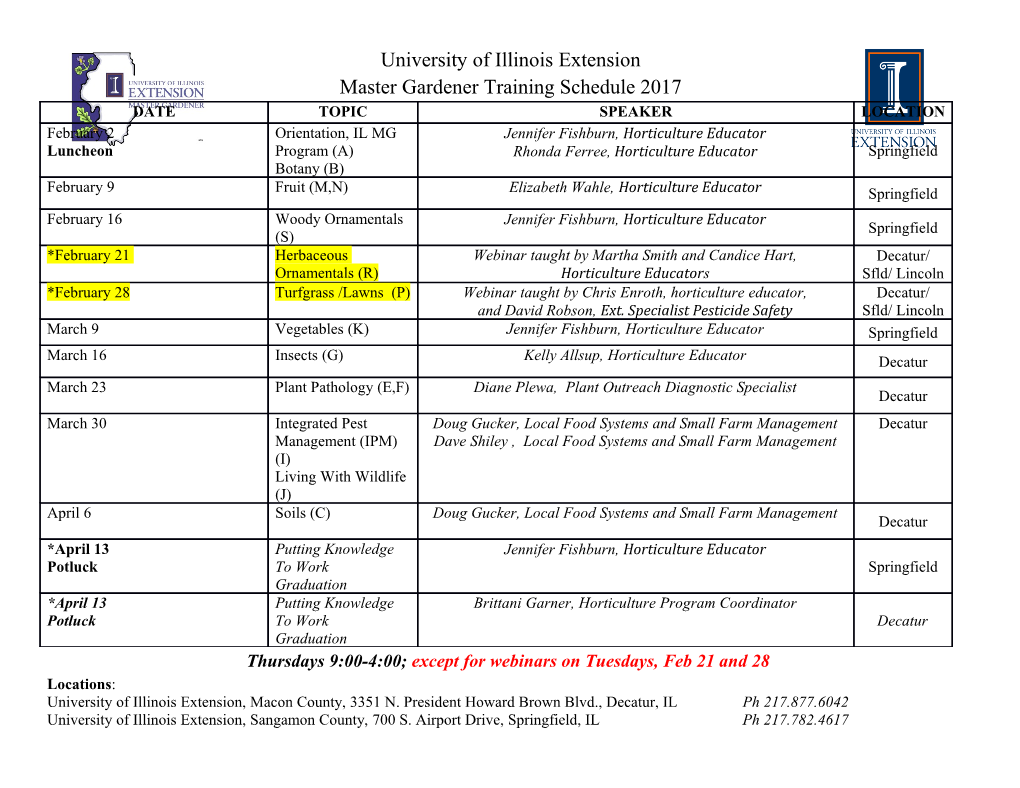
UNIVERSITÀ DEGLI STUDI DI PAVIA DOTTORATO DI RICERCA IN FISICA – XX CICLO Non-Gaussian Stochastic Models and Their Applications in Econophysics Enrica Vera Cisana Tesi per il conseguimento del titolo Università degli Dipartimento di Fisica Istituto Nazionale di Studi di Pavia Nucleare e Teorica Fisica Nucleare DOTTORATO DI RICERCA IN FISICA – XX CICLO Non-Gaussian Stochastic Models and Their Applications in Econophysics dissertation submitted by Enrica Vera Cisana to obtain the degree of DOTTORE DI RICERCA IN FISICA Supervisor: Prof. Guido Montagna Referee: Prof. Rosario N. Mantegna Cover Left: Traders at work in the New York Stock Exchange (NYSE). Right, top: Simulated paths of the time evolution of volatility process in the Heston model. More details can be found in Fig. 4.1 of this work. Right, bottom: Pictorial representation of the frontier of two-dimensional Brownian motion. Non-Gaussian Stochastic Models and Their Applications in Econophysics Enrica Vera Cisana PhD thesis – University of Pavia Printed in Pavia, Italy, November 2007 ISBN 978-88-95767-06-2 To Maria To Silvana E intanto il tempo passa e tu non passi mai... Se potessi far tornare indietro il mondo farei tornare poi senz'altro te per un attimo di eterno e di profondo in cui tutto sembra e niente c' `e Negramaro, Estate & Immenso Contents Introduction 1 1 Why non-Gaussian models for market dynamics? 5 1.1 Financial markets: complex systems and stochastic dynamics . 6 1.2 The Black and Scholes-Merton paradigm . 7 1.3 Stylized facts of real market dynamics . 9 1.3.1 Non-Gaussian nature of log-return distribution . 10 1.3.2 Random volatility . 13 1.3.3 Price-volatility correlations . 16 2 A non-Gaussian approach to risk measures 21 2.1 A brief introduction to financial risk . 22 2.2 Non-Gaussian closed-form expressions for risk measures . 25 2.3 Empirical analysis of financial data . 28 2.4 Risk analysis . 30 3 Stochastic volatility models: the theoretical approach 37 3.1 Correlated stochastic volatility models . 38 3.2 The Vasicek model . 40 3.3 The Heston model . 47 3.4 The exponential Ornstein-Uhlenbeck model . 54 4 A comparative analysis of stochastic volatility models 61 4.1 Discretization algorithm . 62 4.2 Numerical results . 64 4.2.1 Volatility and return processes . 64 4.2.2 Leverage effect and volatility autocorrelation . 69 4.3 Comparison between theory and empirical data . 71 Conclusions and perspectives 77 A The zero-mean return features 81 iii CONTENTS B The Ornstein-Uhlenbeck process 85 Bibliography 89 Acknowledgement 95 iv List of Figures 1.1 The leptokurtic and skewed probability density of Xerox stock price differences . 11 1.2 One-minute returns of Standard&Poor's 500 index . 12 1.3 Volatility clustering in the Dow Jones index . 13 1.4 Dow Jones index daily returns on the period 1900{2000 . 14 1.5 Distribution of the empirical volatility fitted by the theoretical Log- Normal and Inverse-Gamma distributions . 15 1.6 Prices of real European call options on the German DAX index versus the moneyness . 16 1.7 Smile-shape curve characterizing implied volatility . 17 1.8 Empirical form of the leverage effect . 18 1.9 Empirical form of the volatility autocorrelation . 19 2.1 Methodology to compute Value at Risk and Expected Shortfall . 24 2.2 Convergence of VaR and ES Student-t formulae toward Gaussian limit . 26 2.3 Sensitivity of VaR and ES to variation of the tail index value . 28 2.4 Comparison between empirical complementary cdfs of negative daily returns and two theoretical fits (Gaussian and Student-t) for Au- tostrade SpA, Telecom Italia, Mibtel and Mib30 time series . 30 2.5 Bootstrap histograms for the tail index and the RiskMetrics volatil- ity proxy for Autostrade SpA . 32 2.6 VaR and ES values with 68% CL intervals (bootstrap evaluations), according to different methodologies, for the Italian asset Autostrade SpA and index Mib30 . 33 3.1 Characteristic function of returns in the Vasicek model . 44 3.2 Probability density function of χ2 variables . 49 3.3 Stationary probability distribution of variance and characteristic function of the return pdf in the Heston model . 51 3.4 Volatility autocorrelation in the expOU model . 58 4.1 Simulated volatility paths for the Heston model . 65 v LIST OF FIGURES 4.2 Stationary numerical volatility in comparison with the theory of the Vasicek, Heston and expOU models . 66 4.3 Numerical returns in comparison with the theory of the expOU model 67 4.4 Numerical returns in comparison with the theory of the Vasicek and Heston models . 68 4.5 Numerical leverage effect in comparison with the theory of the Va- sicek and expOU models . 70 4.6 Numerical volatility autocorrelation in comparison with the theory of the Vasicek, Heston and expOU models . 70 4.7 Historical evolution of Fiat SpA, Brembo and Bulgari SpA shares in both linear and log-linear scales . 71 4.8 Fit to historical daily volatility distribution of Bulgari SpA and Fiat SpA . 72 4.9 Probability densities of daily returns in comparison with the Nor- mal, the Student-t and the theory of the expOU model for Fiat SpA, Brembo and Bulgari SpA . 74 4.10 Cumulative density function of daily returns and negative daily returns for Fiat SpA, Brembo and Bulgari SpA . 75 4.11 Probability densities of returns on different time lags in comparison with the theory of the expOU model for Fiat SpA, Brembo and Bulgari SpA . 76 vi List of Tables 2.1 Crossover values of the tail index for VaR and ES corresponding to different significance levels . 27 2.2 Estimated parameters values (mean, volatility and tail index) of the fitted curves of Fig. 2.4 for Autostrade SpA, Telecom Italia, Mibtel and Mib30 time series . 31 2.3 Parameters values with the 68% CL intervals (bootstrap evalua- tions) for the time series as in Tab. 2.2 . 32 2.4 Estimated VaR values with 68% CL intervals (bootstrap evalua- tions), according to different methodologies, for the time series as in Tab. 2.2 . 34 2.5 Estimated ES values with 68% CL intervals (bootstrap evalua- tions), according to different methodologies, for the time series as in Tab. 2.2 . 35 3.1 Models of volatility . 40 3.2 Theoretical features of the Vasicek, Heston and expOU stochastic volatility models . 59 4.1 Estimated mean values of Fiat SpA, Brembo and Bulgari SpA re- turn series for different time lags . 73 4.2 Estimated parameter values of the fitted curves shown in Fig. 4.9 for Fiat SpA, Brembo and Bulgari SpA time series . 74 vii LIST OF TABLES viii Introduction Physics and finance could seem, in principle, two different cultures. Nevertheless, reading the book of Emanuel Derman [1], one of the first physi- cists to put physics into Wall Street, or the paper of Fabrizio Lillo, Salvatore Miccich`e and Rosario N. Mantegna [2], the latter being one of the first physicists to put finance into academical physics, could certainly help understand how one can justify using the methods of physics and the formalism of mathematics in the frenzied world of finance. Even so, no all the doubts could have been dispelled: if the value of a certain financial good is determined by people, how can the human behaviour be described by equations or predetermined rules? This could sound, perhaps, a more \philosophical" question, arising from the dissimilar nature of knowledge of physics and social sciences. On this matter, we cannot forget the prophetical words of Ettore Majorana, who identified the analogy between physical and social sciences into the statistical character of the laws describing elementary processes, emerging in the framework of quantum mechanics [3]. More recently, Giorgio Parisi has remarked that, besides statistical physics and quantum me- chanics quoted by Majorana, another revolution has \shaken" the world of physics during the last century, that is the study of complex systems. Parisi writes [4] that these three revolutions...have changed the meaning of the word prediction and the positive consequence of this process is that the scope of physics becomes much larger and the constructions of physics find many more applications. The contri- bution of physicists to the study of economical markets must be interpreted in the light of these words: complexity turns out to be, thus, the reading-key. There are many natural phenomena which exhibit the typical features of com- plex systems, like earthquakes, the DNA, the traffic flow and, clearly, financial markets. Among the methods developed to study their complex dynamics, the theory of stochastic processes still plays a crucial role in describing and modeling economical systems. Thus, it is not so surprising that the first contact between physics and finance must be traced back to 1900, when Louis Bachelier, attempt- ing to reproduce the price dynamics of some goods traded in the Paris Stock Exchange, developed the model of random walk [5]. This physical phenomenon, which is the mathematical formulation of the Brownian motion [6], is commonly considered by physicists as the archetype of stochastic processes. 1 Introduction The relevance of Bachelier's pioneering ideas, forgotten for a long time, has been rediscovered within the economical community in the late '50s and defini- tively hallowed by the works of Fisher Black and Myron Scholes [7], and Robert Merton [8] (BSM). In their theory of option pricing, they assumed that the fluctu- ations in the value of the underlying feature a geometric Brownian motion (GBM), a stochastic process strictly related to the original Brownian motion. Since its pub- lication in 1973, this theory has become a standard in the world of quantitative finance, so that we could state that the history of quantitative finance and econo- physics after 1973 is, essentially, the history of the ways in which academics and financial practitioners have refined and extended the BSM model.
Details
-
File Typepdf
-
Upload Time-
-
Content LanguagesEnglish
-
Upload UserAnonymous/Not logged-in
-
File Pages109 Page
-
File Size-