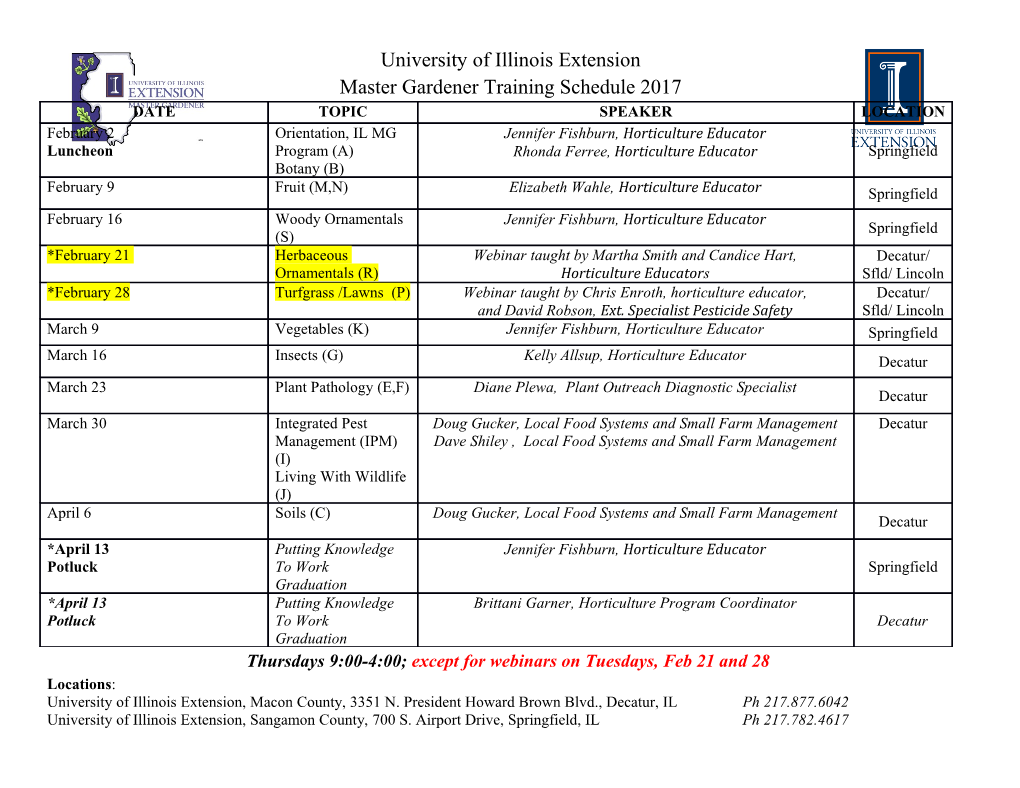
Automatic Labeling of Hypernymy-Troponymy Relation for Chinese Verbs -文動^上下M關Âê動標記法 國Ë台c+Ä'xñ語xû©ë論文 Master Thesis Department of English, National Taiwan Normal University 指導Y授: 謝舒ñZë Advisor: Dr. Shu-Kai Hsieh 研v生: 羅巧Ê Student: Chiao-Shan Lo -華民國]Akt七月 July, 2009 Abstract 近t來,^Y²路(Wordnet)已成º計算語言xø關領域-最ºnM)(的Ç源K一,對¼Ç 訊¢"(Information Retrieval)或/ê6語言U理(Natural Language Processing)的|U有øv '的k©。^Y²路/1同©^Æ(Synset)以Ê^Y語意關Â(Lexical Semantic Relation)@ú Ë而成,例如以ñ語º;的n林¯頓^²(Princeton WordNet)、以ÊP合多個P2語言的P 語^²(EuroWordNet)I,úË皆已øv完善。6而,一個^²的úË&^一B一ºK力@能 完成,v@需要的º力以Ê耗»的B間øv可觀。因d,如U有H率&有ûq的úË一個^² /近t來研v致力的目標。而^Y間的語意關Â/Ë成一個^²的;要C ,因d,如Uê動 化的½取^Y語©關Â/úË^²的Í要e_K一。-研b語言@已úË一個以-;^º;的 -文^Y²路(Chinese WordNet, CWN),è(Ð供完t的-文­YK^©@分。6而,(目 M-文^Y²路ûq-,同©^Æ間ø互的語意關ÂC/¡(ºº$定標記,且這些標記Kx Ï尚*T成可L應(K一定規!。因d,,研vÐú一WJê動化的¹法來ê動標記^Y間的 語意關Â,,Ç論文針對動^K間的上下M^Y語意關Â(Hypernymy-troponymy relation), Ðú一.ê動標記的¹法,&½取w有-文上下M關ÂK-文動^D對。 ,Ç論文Ðúi.&LK¹法,,一,藉1句法上y定的句型(lexical syntactic pattern), ê動½取ú-文^Y²路-w有上下M關ÂK動^D。,二,我們)(bootstrapping的¹法, 透N-研búË的-ñ雙語^²(Sinica Bow)'Ï將n林¯頓ñ語^²-的語意關Â對 至- 文。實WP果o:,dûq能ë速&'Ï0ê動½取úw有上下M語意關ÂK-文動^D,, 論文盼能將d¹法應(¼c(|U-的-文^²ê動語意關Â標記,以Ê知X,體Kê動ú Ë,2而能有H率的úË完善的-文^Y知XÇ源。 關關關uuu^^^:::語語語©©©關關關ÂÂÂêêê動動動標標標記記記、、、動動動^^^^^^YYY語語語意意意、、、動動動^^^上上上下下下MMM關關關ÂÂÂ、、、---文文文^^^²²² Abstract WordNet-like databases have become crucial sources for lexical semantic studies and compu- tational linguistic applications such as Information Retrieval (IR) and Natural Language Pro- cessing (NLP). The fundamental elements of WordNet are synsets (the synonymous grouping of words) and semantic relations among synsets. However, creating such a lexical network is a time-consuming and labor-intensive project. In particular, for those languages with few re- sources such as Chinese, is even difficult. Chinese WordNet (CWN), which composed of mid- dle frequency words, has been launched by Academia Sinica based on the similar paradigm as Princeton WordNet. The synset that each word sense locates in CWN is manually la- beled. However, the lexical semantic relations among synsets in CWN are only partially con- structed and lack of systematic labeling. Therefore, in this thesis, two independent approaches were proposed to automatically harvesting lexical semantic relations, especially focused on the hypernymy-troponymy relation of verbs. This thesis describes two approaches for discovering hypernymy-troponymy relation among verbs. Syntactic pattern-based approach is used for that sentence structures can always denote relations and reveal information among lexical entries. Bootstrapping approach, on the other hand, aims at exploiting an already existing database and combining them within a common, standard framework. From a large scale of input data, our proposed approaches can greatly and rapidly extract verb pairs that are in hypernymy-troponymy relation in Chinese, aiding the construction of lexical database in a more effective way. In addition, it is hoped that these ap- proaches will shed light on the task of automatic acquisition of other Chinese lexical semantic relations and ontology learning as well. Key word: automatic extraction, lexical semantic relation, troponymy, Chinese WordNet i ACKNOWLEDGEMENTS B¼0了ë謝^的這一;,從開Ë撰ë論文沒多E我1一邊Ë思W謝^的g¹,因º一路 p來,實(有*多º要感謝了。ë論文/一段+wÈN¬的N程,這段N程-88G0各.挑 0與瓶8,=/讓我pÃ不已。不N很xK0,這一路上=/有1多º8ú援K,不論/xS 上的見ã,或/精^上的/持,都f予我«'的k©。(d,我要向這些ºññhT我1w的 感謝。 首H,我要感謝我的指導Y授,謝舒ñ老+。©二B,我擔û老+的©理,&且修了老+ (研v@開的Ï一堂²,對計算語言x的認X可ª/受0老+的_蒙,讓我¥ø0了語言x另 一個h新的領域。(ë論文的N程-,老+=/f我^8ê1的z間»|揮,&且對我Ðú的 疑O跟想法都f予ãT與/持,而謝老+沉i的個'_/最能安定ºÃ的力Ï,Ïv我因ºG 0瓶8而&躁不安B,老+=/有¦法不疾不徐0T©我ãz困ã。 另外,我_要感謝我的iMãf委á:台'外文û的高g明老+,以Ê?'ñ語û的~曉 ³老+。高老+(我i!ãfB,=/Ðú1多精闢的見ã,不論/(語言x¹b或/計算程 式¹b,都f了我很多很實(的úp,ãfP_後高老+更/±Ã0Ð供我需要的Ç源&回T 我的疑O。而~老+_/(~忙K-½zM來擔ûãf委á,儘¡如d,~老+還/(我的論 文á,ÆÆ»»0ë下y的意見&點ú論文的:點。感謝iM老+的k忙,這Ç論文M能完 成。 我_要感謝+'ÏM*秀的老+Ê同x,謝謝ÏM曾經YN我的老+,(+'的Ï一門² 都/既.實ÈP富,一點一滴/M我對語言x的知X以Ê撰ë論文的能力。還有班上*秀的同 x 們 ,Nancy, Caroline, David, Fu-Pin, Clara, and Jessica II , 雖 60了後來'¶因º工\或論文,各êª力很少見b,F/我們Í6會(z閒B¤換Ã得,f |d鼓õ。 ddK外,我要1w的感謝-研b語言@的程式-計+,N龍jH生。如果沒有`±Ã的 ii k忙,這Ç論文不可能完成。還要感謝-研b的!;、俞­以Ê淳涵,感謝`們(~忙K-> 下K邊的工\來T©我分析£上CF的語料。我_要感謝我的0Ë們: 7蓉、øK、徐\。 ë論文有多痛苦,真的要ëNM知S,}ª£些ã¬的日Pá有`們的j4,互吐苦4,ø互 勉勵。 最後,_/最Í要的,我要感謝我的¶º,感謝8½=/!條件的(背後/持我,不論/ (精^上或/iê上都f我«'的k©,還有¹¹不BN來的關Ã與O候,都讓我倍感©Ã。 謝謝`們一路j我pN來,/持我@Z的Ï一個z定,9以這,論文{f`們---- 我最愛 的¶º。 iii Contents 1 Introduction 1 1.1 Background . 1 1.2 Motivation . 3 1.3 Organization of the Thesis . 4 2 Related Works 5 2.1 WordNet-like Resources . 5 2.1.1 Princeton WordNet [31] . 6 2.1.2 EuroWordNet [45] . 7 2.1.3 Sinica Bow [23] . 8 2.1.4 Chinese WordNet [1] . 10 2.1.5 HowNet [14] . 12 2.2 Semantic Relations of Verbs . 13 2.2.1 Semantic Relations of Verbs in WordNet . 13 2.2.2 Semantic Relations of Verbs in EuroWordNet . 16 2.2.3 Other Relations of Verbs . 20 2.3 Troponymy . 22 2.3.1 Definition of Troponymy . 24 iv 2.3.2 Distinguishing Manner . 26 2.4 Automatic Discovery of Lexical Semantic Relation . 28 2.4.1 Lexico Syntactic Pattern–Based Approach . 29 2.4.2 Clustering-Based Approach . 32 2.4.3 Bootstrapping Approach . 33 2.5 Summary . 35 3 Methodology 37 3.1 Syntactic Pattern-Based Approach . 37 3.1.1 Database: Chinese WordNet . 37 3.1.2 Data Pre-processing . 39 3.1.3 Syntactic Patterns in Chinese . 41 3.1.4 Procedure . 42 3.2 Bootstrapping Approach . 44 3.2.1 Data Source . 46 3.2.2 Procedure . 48 3.3 Evaluation and Scoring . 49 3.3.1 Evaluation . 50 3.3.2 Scoring . 54 3.4 Summary . 55 4 Results and Error Analyses 56 4.1 Results from Syntactic Pattern- based Approach . 56 4.1.1 Error Analyses . 58 4.1.2 Interim Summary . 68 v 4.2 Results from Bootstrapping Approach . 69 4.2.1 Error Analyses . 70 4.3 Discussion . 81 4.3.1 Comparison of Two Approaches . 81 4.3.2 Comparison of the Results . 83 4.3.3 Comparison of the Error Types . 86 4.3.4 General Discussion . 89 4.4 Summary . 91 5 Conclusion 92 5.1 Summary of the Thesis . 92 5.2 Contribution . 94 5.3 Limitations of the Present Study and Suggestions for Future Work . 95 Appendix: A Programming Code 104 B Results from Syntactic Pattern-based Approach 107 C Results from Bootstrapping Approach 110 vi List of Tables 2.1 A finer-grained semantic relation among verbs. [9] . 21 2.2 Semantic relations of verbs in Wordnet, EuroWordNet and VerbOcean . 23 2.3 Three different types of Troponymy . 28 4.1 General results of syntactic pattern-based approach . 57 4.2 Error types and percentage . 59 4.3 Overall results from bootstrapping approach . 70 4.4 Non hypernymy-troponymy verb pairs (Total number of returned verb pairs= 11289) . 71 4.5 General comparison of syntactic pattern-based and bootstrapping approach . 82 4.6 Comparison of error types from results in two approaches . 86 4.7 General comparison of the two approaches . 89 vii List of Figures 2.1 The first two senses returned by CWN of the verb p ‘zao3, walk’ . 11 2.2 Four kinds of entailments among English verbs [31] . 15 2.3 Translation-mediated LSR Prediction (The complete model) . 33 2.4 Translation-mediated LSR Prediction (when translation equivalents are syn- onymous) . 34 3.1 Bootstrapping model . 45 3.2 Overall procedure of bootstrapping approach . 50 viii Chapter 1 Introduction 1.1 Background In recent years, there has been an increasing focus on the construction of lexical knowledge re- sources in the field of Natural Language Processing (NLP), such as Thesaurus, WordNets [31], EuroWordNet [45], FrameNet [6], HowNet [13], etc. Among these resources, Princeton Word- Net1, an electronic English lexical database, was started as an implementation of a psycholin- guistic model of the mental lexicon. In WordNet, English nouns, verbs, adjectives, and ad- verbs are organized into synonym sets, called synsets. Synsets in WordNet are connected with each other by various kinds of paradigmatic lexical semantic relations, such as Meronymy and Holonymy (between parts and wholes), Hypernymy and Hyponymy (between specific and more general synsets), etc. These relations act as pointers between synsets. Due to the seman- tic relation-based property, WordNet has been widely used to solve a variety of problems in the field of NLP and has sparked off most interest both in theoretical and applicational sides, such as Information Retrieval (IR), lexical acquisition, automatic extraction, Word Sense Dis- ambiguation (WSD), and so on. WordNet’s growing popularity has prompted the modeling and 1http://wordnet.princeton.edu 1 construction of wordnets in other languages and various domains as well. EuroWordNet [45], which aims to build a multilingual database for several European languages, is a successful example. To date, in the field of NLP applications, WordNet and EuroWordNet serve as very crucial sources and have become a standard norm in evaluating semantic relations. WordNet covers a large scale of sense-based English lexicons (206941 word-sense pairs 2). The extensive coverage of WordNet took immense labors and time. Further, semantic relations are unlimited, it takes years and intensive labors to steadily develop the scope and content. Consequently, there has been significant recent interest in finding methods to build a WordNet-like database in other languages with less efforts and time [5] [7] [9] [20] [21] [24] [28] [30] [32] [38] [39]. Lexical semantic relations among synsets are the foundations of a semantic network, but manually constructing all the relations is time-consuming and error-prone. Therefore, one of the most important steps toward efficiently constructing a WordNet-like database is to auto- matically extract lexical semantic relations.
Details
-
File Typepdf
-
Upload Time-
-
Content LanguagesEnglish
-
Upload UserAnonymous/Not logged-in
-
File Pages122 Page
-
File Size-