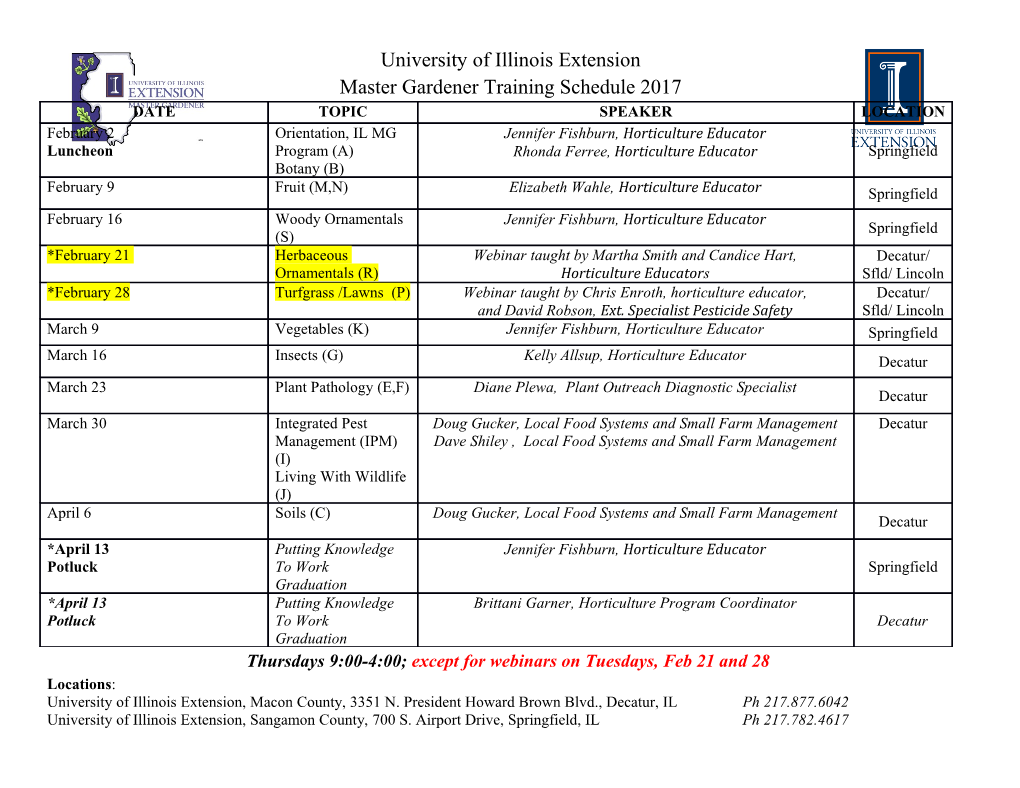
Justifications of Shannon Entropy and Mutual Information in Statistical Inference EE378A Lecture Notes Spring 2017, Stanford University April 19, 2017 Let X = fx1; x2; : : : ; xng be a finite set with jX j = n. Let Γn denote the set of probability measures on X , and let R¯ denote the extended real line. We recall the definition of the logarithmic loss as follows: ¯ Definition 1 (Logarithmic loss). The logarithmic loss `log : X × Γn 7! R is defined by 1 ` = ln ; (1) log P (x) where P (x) denotes the probability of x under measure P . In this lecture, we go over some elegant results justifying the use of the logarithmic loss in statistical inference. Recall the following lemma that were introduced in Lecture 2: Lemma 1. The true distribution minimizes the expected logarithmic loss: 1 P = arg min EP ln ; (2) Q Q(X) and 1 H(P ) = min EP ln : (3) Q Q(X) Proof. The result follows from the observation that for any Q 2 Γn, 1 1 P (X) ln − ln = ln (4) EP Q(X) EP P (X) EP Q(X) X P (x) = P (x) ln (5) Q(x) x2X X Q(x) = P (x) − ln (6) P (x) x2X ! X Q(x) ≥ − ln P (x) (7) P (x) x2X = 0; (8) where we applied Jensen's inequality for the convex function − ln x on (0; 1). Lemma 1 is the foundational result behind the use of the cross entropy loss in machine learning. A natural question arises: is the logarithmic loss the only loss function that satisfies the property that the true distribution minimizes the expected loss? Perhaps surprisingly, it is true under a natural \locality" constraint. The following result is also known as the fact that the logarithmic scoring rule is the unique proper scoring rule. 1 Theorem 1. [1] Suppose n ≥ 3. Then, the only function f such that for any P P 2 arg min EP f(Q(X)) (9) Q is of the form 1 f(p) = c ln + b for all p 2 (0; 1); (10) p where b; c ≥ 0 are constants. Theorem 1 does not hold if n = 2. We refer to [2] for detailed discussions regarding the dichotomy between the binary and non-binary alphabets. 1 Justification of the Shannon entropy (and the logarithmic loss) Csiszar [3] provided a survey of axiomatic characterizations of information measures up to 2008. Csiszar stated that \the intuitively most appealing axiomatic result is due to Aczel{Forte{Ng [4]", which characterized the Shannon entropy as a natural measure to measure uncertainty. We quote their results below. Theorem 2. [4] Let K(P ) be a general functional of discrete distribution P . We assume the following axioms: 1. Subadditivity: K(PXY ) ≤ K(PX ) + K(PY ); (11) 2. Additivity: when X and Y are independent, K(PXY ) = K(PX ) + K(PY ); (12) 3. Expansibility: K(p1; p2; : : : ; pm; 0) = K(p1; p2; : : : ; pm); (13) 4. Symmetry: for all permutations π, K(p1; p2; : : : ; pm) = K(pπ(1); pπ(2); : : : ; pπ(m)); (14) 5. Normalization: K(1=2; 1=2) = 1; (15) 6. Small for small probabilities: lim K(1 − q; q) = 0: (16) q!0+ P Then, the Shannon entropy H(P ) = x2X −P (x) ln P (x) is the only functional satisfying the axioms above. 2 Justification of mutual information (and the logarithmic loss) For the rest of the lecture, we present a characterization of mutual information that justifies the use of the logarithmic loss from another perspective. Concretely, we ask the following question: Suppose X and Y are dependent random variables. How relevant is Y for inference on X? Toward answering this question, let ` : X × X!^ R¯ be an arbitrary loss function with reconstruction alphabet R, where R is arbitrary. Given (X; Y ) ∼ PXY , it is natural to quantify the benefit of additional 2 side information Y by computing the difference between the expected losses in estimating X 2 X with and without side information Y , respectively. This motivates the following definition: ^ C(`; PXY ) , inf EP [`(X; x^1)] − inf EP [`(X; X2)]; (17) x^12R X^2(Y ) wherex ^1 2 R is deterministic, and X^2 = X^2(Y ) 2 R is any measurable function of Y . In the following discussions, we require that indeterminate forms like 1 − 1 do not appear in the definition of C(`; PXY ). By taking Y to be independent of X, this requirement implies that for all P 2 Γn, inf EP [`(X; x^1)] < 1: (18) x^12R The formulation (17) has appeared previously in the statistics literature. DeGroot [5] in 1962 defined the information contained in an experiment, which turns out to be equivalent to (17). Later, Dawid [6] defined the coherent dependence function, which is equivalent to (17), and used it to quantify the dependence between two random variables X; Y . Our framework of quantifying the predictive benefit of side information is closely connected to the notion of proper scoring rules and the literature on probability forecasting in statistics. The survey by Gneiting and Raftery [7] provides a good overview. Having introduced the yardstick in (17), we now reformulate the question of interest: Which loss func- tion(s) ` can be used to define C(`; PXY ) in a meaningful way? Of course, \meaningful" is open to in- terpretation, but it is desirable that C(`; PXY ) be well-defined, at minimum. This motivates the following axiom: Axiom 1. For all distributions PXY , the quantity C(`; PXY ) satisfies C(`; PTY ) ≤ C(`; PXY ) whenever T (X) 2 X is a statistically sufficient transformation of X for Y . We remind the reader that the statement `T is a statistically sufficient transform of X for Y ' means that the following two Markov chains hold: T − X − Y; X − T − Y (19) That is, T (X) preserves all of the information X contains about Y . In words, the Data Processing Axiom stipulates that processing the data X ! T cannot boost the predictive benefit of the side information1. To convince the reader that the Data Processing Axiom is a natural requirement, suppose instead that the Data Processing Axiom did not hold. Since X and T are mutually sufficient statistics for Y , this would imply that there is no unique value which quantifies the benefit of side information Y for the random variable of interest. Thus, the Data Processing Axiom is needed for the benefit of side information to be well-defined. Although the Data Processing Axiom may seem to be a benign requirement, it has far-reaching implica- tions for the form C(`; PXY ) can take. This is captured by our first main result: Theorem 3. [8] Let n ≥ 3. Under the Data Processing Axiom, the function C(`; PXY ) is uniquely deter- mined by the mutual information, C(`; PXY ) = I(X; Y ); (20) up to a multiplicative factor. We prove Theorem 3 below. To begin, we show that the measure of relevance defined in (17) is equivalently characterized by a bounded convex function defined on the X -simplex. The following lemma achieves this goal. Lemma 2. There exists a bounded convex function V :Γn ! R, depending on `, such that ! X C(`; PXY ) = PY (y)V (PXjY =y) − V (PX ): (21) y 1In fact, the Data Processing Axiom is weaker than this general data processing statement since it only addresses statistically sufficient transformations of X. 3 The proof of Lemma 2 follows from defining V (P ) by V (P ) = − inf EP [`(X; x^)]; (22) x^2R and its details are deferred to the appendix. In the statistics literature, the quantity −V (P ) is usually called the generalized entropy or the Bayes envelope. The next lemma asserts that we only need to consider symmetric (invariant to permutations) functions V (P ). Lemma 3. Under the Data Processing Axiom, there exists a symmetric finite convex function G :Γn ! R, such that ! X C(`; PXY ) = PY (y)G(PXjY =y) − G(PX ); (23) y and G(·) is equal to V (·) in Lemma 2 up to a linear translation: G(P ) = V (P ) + hc; P i; (24) where c 2 Rn is a constant vector. The proof of Lemma 3 follows by applying a permutation to the space X and applying the Data Processing Axiom. Details are deferred to the appendix. Now we are in a position to begin the proof of Theorem 3 in earnest. It suffices to consider the case when the side information Y is binary valued, i.e., Y 2 f1; 2g. We will show that the Data Processing Axiom mandates the usage of the logarithmic loss even when we constrain ourselves to this situation. Define α fY = 1g. Take P (t);P (t) to be two probability distribution on X parametrized in the , P λ1 λ2 following way: P (t) = (λ t; λ (1 − t); r − λ ; p ; : : : ; p ) (25) λ1 1 1 1 4 n P (t) = (λ t; λ (1 − t); r − λ ; p ; : : : ; p ); (26) λ2 2 2 2 4 n P where r , 1 − i≥4 pi; t 2 [0; 1]; 0 ≤ λ1 < λ2 ≤ r. Taking P P (t);P P (t), it follows from Lemma 2 that Xj1 , λ1 Xj2 , λ2 C(`; PXY ) = αV (P (t)) + (1 − α)V (P (t)) − V (αP (t) + (1 − α)P (t)): (27) λ1 λ2 λ1 λ2 Note that the following transformation T (X) is a statistically sufficient transformation of X for Y : ( x1 X 2 fx1; x2g; T (X) = (28) X otherwise. The Data Processing Axiom implies that for all α 2 [0; 1], t 2 [0; 1] and legitimate λ2 > λ1 ≥ 0, αV (P (t)) + (1 − α)V (P (t)) − V (αP (t) + (1 − α)P (t)) λ1 λ2 λ1 λ2 = αV (P (1)) + (1 − α)V (P (1)) − V (αP (1) + (1 − α)P (1)): (29) λ1 λ2 λ1 λ2 We now define the function (t) R(λ, t) , V (Pλ ); (30) where we note that the bi-variate function R(λ, t) implicitly depends on the parameter p4; p5 : : : pn which we shall fix for the rest of this proof.
Details
-
File Typepdf
-
Upload Time-
-
Content LanguagesEnglish
-
Upload UserAnonymous/Not logged-in
-
File Pages8 Page
-
File Size-