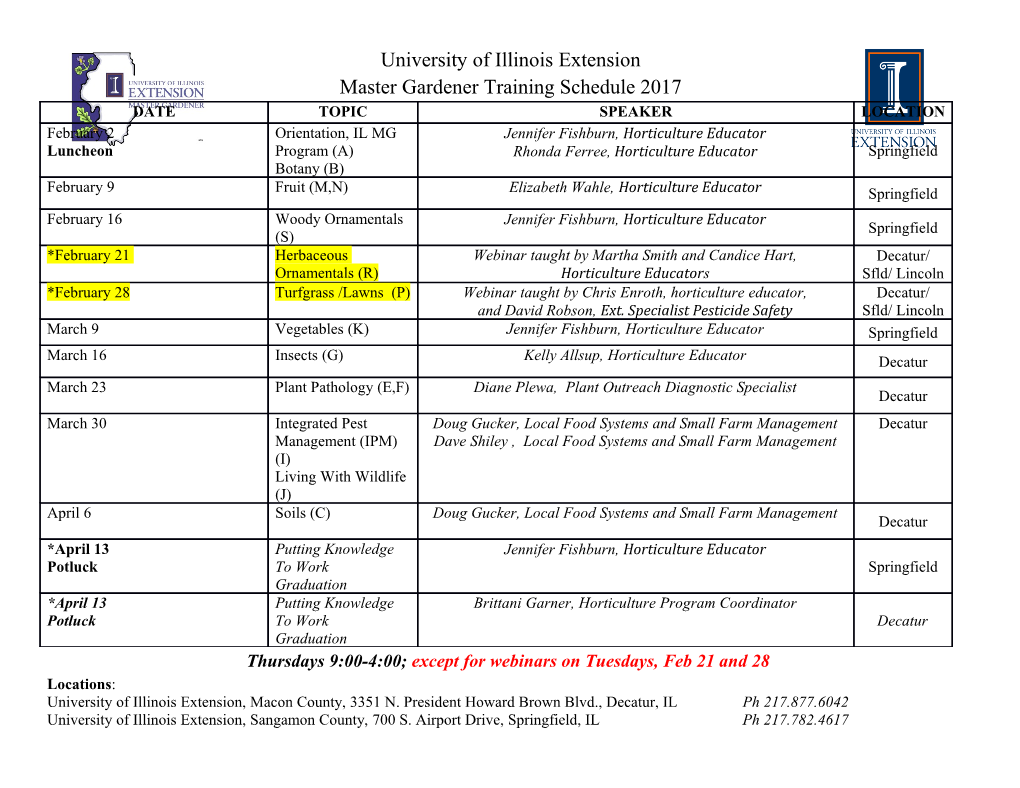
University of Pennsylvania ScholarlyCommons Publicly Accessible Penn Dissertations 2017 Methods For Survival Analysis In Small Samples Rengyi Xu University of Pennsylvania, [email protected] Follow this and additional works at: https://repository.upenn.edu/edissertations Part of the Biostatistics Commons Recommended Citation Xu, Rengyi, "Methods For Survival Analysis In Small Samples" (2017). Publicly Accessible Penn Dissertations. 2649. https://repository.upenn.edu/edissertations/2649 This paper is posted at ScholarlyCommons. https://repository.upenn.edu/edissertations/2649 For more information, please contact [email protected]. Methods For Survival Analysis In Small Samples Abstract Studies with time-to-event endpoints and small sample sizes are commonly seen; however, most statistical methods are based on large sample considerations. We develop novel methods for analyzing crossover and parallel study designs with small sample sizes and time-to-event outcomes. For two- period, two-treatment (2x2) crossover designs, we propose a method in which censored values are treated as missing data and multiply imputed using pre-specified parametric failure time models. The failure times in each imputed dataset are then log-transformed and analyzed using ANCOVA. Results obtained from the imputed datasets are synthesized for point and confidence interval estimation of the treatment-ratio of geometric mean failure times using model-averaging in conjunction with Rubin's combination rule. We use simulations to illustrate the favorable operating characteristics of our method relative to two other existing methods. We apply the proposed method to study the effect of an experimental drug relative to placebo in delaying a symptomatic cardiac-related event during a 10-minute treadmill walking test. For parallel designs for comparing survival times between two groups in the setting of proportional hazards, we propose a refined generalized log-rank (RGLR) statistic by eliminating an unnecessary approximation in the development of Mehrotra and Roth's GLR approach (2001). We show across a variety of simulated scenarios that the RGLR approach provides a smaller bias than the commonly used Cox model, parametric models and the GLR approach in small samples (up to 40 subjects per group), and has notably better efficiency relative to Cox and parametric models in terms of mean squared error. The RGLR approach also consistently delivers adequate confidence interval coverage and type I error control. We further show that while the performance of the parametric model can be significantly influencedy b misspecification of the true underlying survival distribution, the RGLR approach provides a consistently low bias and high relative efficiency. We apply all competing methods to data from two clinical trials studying lung cancer and bladder cancer, respectively. Finally, we further extend the RGLR method to allow for stratification, where stratum-specific estimates are first obtained using RGLR and then combined across strata for overall estimation and inference using two different weighting schemes. We show through simulations the stratified RGLR approach delivers smaller bias and higher efficiency than the commonly used stratified Cox model analysis in small samples, notably so when the assumption of a constant hazard ratio across strata is violated. A dataset is used to illustrate the utility of the proposed new method. Degree Type Dissertation Degree Name Doctor of Philosophy (PhD) Graduate Group Epidemiology & Biostatistics First Advisor Pamela A. Shaw Second Advisor Devan V. Mehrotra Keywords Crossover Trials, Proportional Hazards, Small samples, Survival analysis Subject Categories Biostatistics This dissertation is available at ScholarlyCommons: https://repository.upenn.edu/edissertations/2649 METHODS FOR SURVIVAL ANALYSIS IN SMALL SAMPLES Rengyi Xu A DISSERTATION in Epidemiology and Biostatistics Presented to the Faculties of the University of Pennsylvania in Partial Fulfillment of the Requirements for the Degree of Doctor of Philosophy 2017 Supervisor of Dissertation Co-Supervisor of Dissertation Pamela A. Shaw Devan V. Mehrotra Associate Professor of Biostatistics Adjunct Associate Professor of Biostatistics Graduate Group Chairperson Nandita Mitra, Professor of Biostatistics Dissertation Committee Sharon X. Xie, Professor of Biostatistics Warren B. Bilker, Professor of Biostatistics Shannon L. Maude, Assistant Professor of Pediatrics METHODS FOR SURVIVAL ANALYSIS IN SMALL SAMPLES c COPYRIGHT 2017 Rengyi Xu This work is licensed under the Creative Commons Attribution NonCommercial-ShareAlike 3.0 License To view a copy of this license, visit http://creativecommons.org/licenses/by-nc-sa/3.0/ ACKNOWLEDGEMENT I would like to express my deepest appreciation to my dissertation advisors, Dr. Pamela Shaw and Dr. Devan Mehrotra, for their guidance, dedication and support throughout the process of completing this thesis. I thank them for devoting an incredible amount of energy and time into my dissertation research, and also for teaching me become an independent biostatistician. I would also like to thank my committee members, Dr. Sharon Xie, Dr. Warren Bilker and Dr. Shannon Maude, for their insightful suggestions and feedbacks that greatly improved my dissertation. My sincere appreciation goes to the faculty, staff and students in the Divison of Biostatistics, in- cluding my research advisors, Dr. Mary Putt, Dr. Kathleen Propert and Dr. Sarah Ratcliffe, and my master’s thesis advisor, Dr. Rui Feng, for the collaborative research opportunity and the in- valuable advice and guidance. I would also like to thank Dr. Wei-Ting Hwang and the Superfund Research Program for providing me with the opportunity to collaborate on interesting projects in environmental health. I would also like to acknowledge the friendship and support from my fellow students; my graduate school journey would not be complete without the lovely people I have met at the University of Pennsylvania. Finally, I would like to give my most appreciative and loving gratitude to my parents for their uncon- ditional love and support throughout my life. I thank them for always being there for me every step of the way. This dissertation is dedicated to them. iii ABSTRACT METHODS FOR SURVIVAL ANALYSIS IN SMALL SAMPLES Rengyi Xu Pamela A. Shaw Devan V. Mehrotra Studies with time-to-event endpoints and small sample sizes are commonly seen; however, most statistical methods are based on large sample considerations. We develop novel methods for an- alyzing crossover and parallel study designs with small sample sizes and time-to-event outcomes. For two-period, two-treatment (2×2) crossover designs, we propose a method in which censored values are treated as missing data and multiply imputed using pre-specified parametric failure time models. The failure times in each imputed dataset are then log-transformed and analyzed using ANCOVA. Results obtained from the imputed datasets are synthesized for point and confidence interval estimation of the treatment-ratio of geometric mean failure times using model-averaging in conjunction with Rubin’s combination rule. We use simulations to illustrate the favorable operat- ing characteristics of our method relative to two other existing methods. We apply the proposed method to study the effect of an experimental drug relative to placebo in delaying a symptomatic cardiac-related event during a 10-minute treadmill walking test. For parallel designs for comparing survival times between two groups in the setting of proportional hazards, we propose a refined gen- eralized log-rank (RGLR) statistic by eliminating an unnecessary approximation in the development of Mehrotra and Roth’s GLR approach (2001). We show across a variety of simulated scenarios that the RGLR approach provides a smaller bias than the commonly used Cox model, parametric models and the GLR approach in small samples (up to 40 subjects per group), and has notably better efficiency relative to Cox and parametric models in terms of mean squared error. The RGLR approach also consistently delivers adequate confidence interval coverage and type I error control. We further show that while the performance of the parametric model can be significantly influenced by misspecification of the true underlying survival distribution, the RGLR approach provides a con- sistently low bias and high relative efficiency. We apply all competing methods to data from two clinical trials studying lung cancer and bladder cancer, respectively. Finally, we further extend the iv RGLR method to allow for stratification, where stratum-specific estimates are first obtained using RGLR and then combined across strata for overall estimation and inference using two different weighting schemes. We show through simulations the stratified RGLR approach delivers smaller bias and higher efficiency than the commonly used stratified Cox model analysis in small samples, notably so when the assumption of a constant hazard ratio across strata is violated. A dataset is used to illustrate the utility of the proposed new method. v TABLE OF CONTENTS ACKNOWLEDGEMENT . iii ABSTRACT . iv LIST OF TABLES . ix LIST OF ILLUSTRATIONS . xi CHAPTER 1 : INTRODUCTION .................................. 1 1.1 Background . 1 1.2 Novel Developments . 4 CHAPTER 2 : INCORPORATING BASELINE MEASUREMENTS IN CROSSOVER TRIALS WITH TIME-TO-EVENT ENDPOINTS ........................... 6 2.1 Introduction . 6 2.2 Methods . 8 2.3 Simulation . 12 2.4 Data Application . 16 2.5
Details
-
File Typepdf
-
Upload Time-
-
Content LanguagesEnglish
-
Upload UserAnonymous/Not logged-in
-
File Pages91 Page
-
File Size-