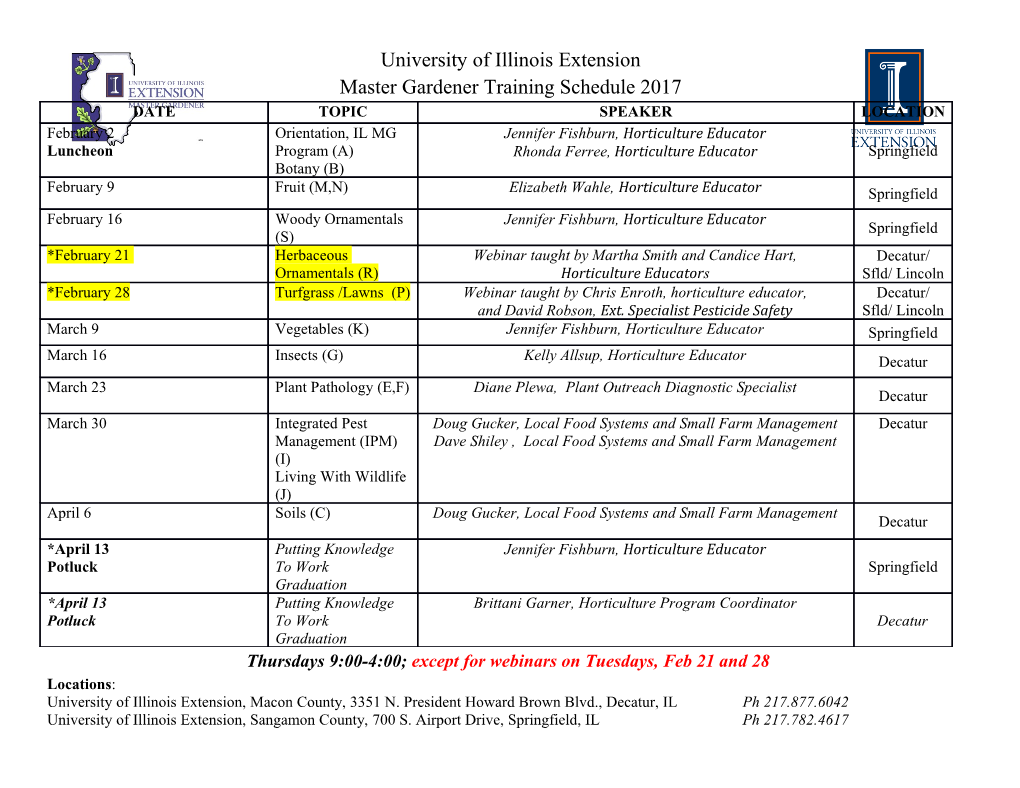
Designing a Collaborative Process to Create Bilingual Dictionaries of Indonesian Ethnic Languages 1Arbi Haza Nasution, 2Yohei Murakami, 3Toru Ishida 1;3Department of Social Informatics, Kyoto University, 2Unit of Design, Kyoto University Kyoto, Japan [email protected], [email protected], [email protected] Abstract The constraint-based approach has been proven useful for inducing bilingual dictionary for closely-related low-resource languages. When we want to create multiple bilingual dictionaries linking several languages, we need to consider manual creation by a native speaker if there are no available machine-readable dictionaries are available as input. To overcome the difficulty in planning the creation of bilingual dictionaries, the consideration of various methods and costs, plan optimization is essential. Utilizing both constraint-based approach and plan optimizer, we design a collaborative process for creating 10 bilingual dictionaries from every combination of 5 languages, i.e., Indonesian, Malay, Minangkabau, Javanese, and Sundanese. We further design an online collaborative dictionary generation to bridge spatial gap between native speakers. We define a heuristic plan that only utilizes manual investment by the native speaker to evaluate our optimal plan with total cost as an evaluation metric. The optimal plan outperformed the heuristic plan with a 63.3% cost reduction. Keywords: Bilingual Dictionary Creation, Low-resource Languages, Closely-related Languages 1. Introduction guage. Then, the bilingual dictionary DA−B can be in- duced from the triple TA−ID−B. The native speakers need Nowadays, machine-readable bilingual dictionaries are be- a tool that can bridge the spatial gap and help them collab- ing utilized in actual services (Ishida, 2011) to support orate. To actually implement our pivot-based bilingual dic- intercultural collaboration (Ishida, 2016; Nasution et al., tionary induction following the optimal plan to create mul- 2017b), but low-resource languages lack such sources. Ob- tiple Indonesian ethnic languages bilingual dictionaries, we viously bilingual lexicon extraction is highly problematic address the following research goals: for low-resource languages due to the paucity or outright omission of parallel and comparable corpora. We intro- • Designing a Collaborative Process for Creating Bilin- duced the promising approach of treating pivot-based bilin- gual Dictionaries of Indonesian Ethnic Languages: gual dictionary induction for low-resource languages as an Implementing plan optimization for creating bilin- optimization problem (Nasution et al., 2016; Nasution et gual dictionaries of low-resource languages and im- al., 2017c) where bilingual dictionaries are the only lan- plementing a generalized constraint approach to bilin- guage resource required. Despite the high potential of our gual dictionary induction for low-resource language approach in enriching low-resource languages, it faces nu- families in creating 10 bilingual dictionaries with merous issues when trying to create plans to implement 2,000 translation pairs from every combination of 5 multiple bilingual dictionaries for a set of low-resource lan- languages, i.e., Indonesian, Malay, Minangkabau, Ja- guages like Indonesian ethnic languages. When actually vanese, and Sundanese. implementing our constraint-based bilingual dictionary in- duction approach, we need to consider the inclusion of • Designing an Online Collaborative Dictionary Gen- more traditional methods like manually creating the bilin- eration: Bridging spatial gap between native speakers gual dictionaries by native speaker. In spite of the high cost, especially when doing a collaborative creation or eval- this will be unavoidable if no machine-readable dictionar- uation of bilingual dictionary. ies are available. Given the various methods and costs that The rest of this paper is organized as follows: In Section 2 may need to be considered, we recently introduced a plan and Section 3, we will briefly discuss our constraint-based optimizer to find the feasible optimal plan of creating multi- bilingual dictionary induction and plan optimizer, respec- ple bilingual dictionaries with the least total cost (Nasution tively. Section 4 details our collaborative process design. et al., 2017a). In this project, to create bilingual dictionary Finally, Section 5 concludes this paper. DA−B between ethnic language LA and ethnic language LB, there is also a difficulty in finding a bilingual native 2. Constraint-Based Bilingual Dictionary speaker of two ethnic languages. To overcome this limita- Induction tion, we can firstly create triple TA−ID−B using the com- mon language, Indonesian as pivot language LID where The traditional pivot-based approach is very suitable for SID−A, a native bilingual speaker of Indonesian language low-resource languages (Tanaka and Umemura, 1994). Un- LID - ethnic language LA and SID−B, a native bilingual fortunately, for some low-resource languages, it is often speaker of Indonesian language LID - ethnic language LB difficult to find machine-readable inverse dictionaries and collaborate by explaining the senses with Indonesian lan- corpora to identify and eliminate the erroneous translation 3397 Transgraphs Translation Output Input Dictionaries Transgraphs with new edges Results Dictionary � � � � � � �� �� �� � � � CNF Dictionary � � � 1. (�&, �() A-B % % 2. (�&, �() � � �� � � � WPMax-SAT + , �� �� � �� �� �� Dictionary Solver Dictionary C-B A-C �� � � �� Figure 1: One-to-one constraint approach to pivot-based bilingual dictionary induction. Dictionary set resource paucity because few such pairs can be found. A-B Dictionary DA-C Therefore, we generalized the constraint-based bilingual Dictionary A-C B-C dictionary induction framework by extending constraints Dictionary and translation pair candidates from the one-to-one ap- A-D Dictionary proach to attain more voluminous bilingual dictionary re- Dictionary A-C DA-B DB-C DA-D DD-C D-C sults with many-to-many translation pairs extracted from (DA-B AND DB-C) OR (DA-D AND DD-C) connected existing and new edges (Nasution et al., 2016). We further enhance our generalized method by setting two Figure 2: Modeling Bilingual Dictionary Induction Depen- steps to obtaining translation pair results. First, we identify dency. one-to-one cognates by incorporating more constraints and heuristics to improve the quality of the translation result. We then identify the cognates’ synonyms to obtain many- to-many translation pairs. In each step, we can obtain more cognate and cognate synonym pair candidates by iterating DA-B DB-C the n-cycle symmetry assumption until all possible trans- p3 p1 p9 p10 lation pair candidates have been reached (Nasution et al., p2 p12 2017c). DA-C p4 DC-D p5 p p p7 11 6 p8 3. Plan Optimizer DA-D DB-D Our constraint-based bilingual dictionary induction ap- proach has the potential to enrich low-resource languages Figure 3: AND/OR Graph as an MDP State. with the only input being machine readable bilingual dic- tionaries. Unfortunately, the scarcity of such dictionaries for low-resource languages makes it difficult to plan which bilingual dictionary should be invested first or which bilin- pair candidates. To overcome this limitation, our team gual dictionary should be induced right from the start in (Wushouer et al., 2015) proposed to treat pivot-based bilin- order to obtain all possible combination of bilingual dictio- gual lexicon induction as an optimization problem. The naries from the language set with the minimum total cost assumption was that lexicons of closely-related languages to be paid. We model the bilingual dictionary dependency offer instances of one-to-one mapping and share a signifi- with AND/OR graphs as shown in Figure 2, and employ cant number of cognates (words with similar spelling/form the Markov Decision Process (MDP) for plan optimization and meaning originating from the same root language). The where a state is defined by AND/OR graphs as shown in proposal uses a graph whose vertices represent words and Figure 3. The exponential complexity of formulating the edges indicate shared meanings; following (Soderland et bilingual dictionary creation planning into a graph theory al., 2009) it was called a transgraph. The proposal pro- problem indicates a greater complexity of obtaining the op- ceeds as follows: (1) use two bilingual dictionaries as in- timal planning with the least total cost by only following the A A put, (2) represent them as transgraphs where w1 and w2 heuristic. Nevertheless, our algorithm greatly reduced the B B are non-pivot words in language LA, w1 and w2 are pivot complexity, so that the MDP planning can find the feasible C C C words in language LB, and w1 , w2 and w3 are non-pivot optimal plan with less total cost compared to heuristic plan- words in language LC , (3) add some new edges represented ning (e.g., only use manual investment by native speaker). by dashed edges based on the one-to-one assumption, (4) Our MDP model can calculate the cumulative cost while formalize the problem into conjunctive normal form (CNF) predicting and considering the probability of the pivot ac- and use the Weighted Partial MaxSAT (WPMaxSAT) solver tion to yield a satisfying output bilingual dictionary as util- (Ansotegui´ et al., 2009) to return the optimized transla- ity for every state to better predict the most feasible optimal tion results, and (5) output the induced bilingual dictionary plan with the least total cost. Our formalization with MDP as the
Details
-
File Typepdf
-
Upload Time-
-
Content LanguagesEnglish
-
Upload UserAnonymous/Not logged-in
-
File Pages8 Page
-
File Size-