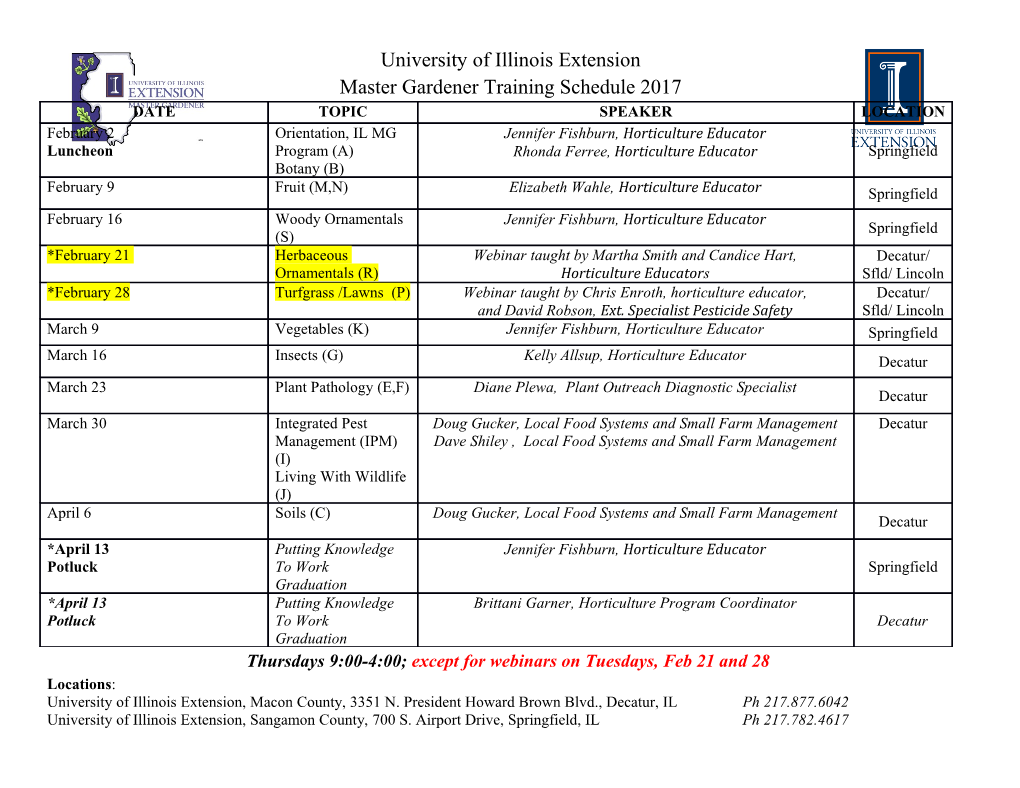
From: ISMB-95 Proceedings. Copyright © 1995, AAAI (www.aaai.org). All rights reserved. Relation between Protein Structure, Sequence Homology and Composition of Amino Acids Eddy Mayoraz Inna Dubchak Ilya Muchnik RUTCOl~--Rutgers University’s Department of Chemistry, RUTCOl~--ttutgers University’s Center for Operations Research, University of California Center for Operations Research, P.O. Box 5062, New Brunswick, at Berkeley, Berkeley, P.O. Box 5062, New Brunswick, NJ 08903-5062, CA 94720, NJ 08903-5062, [email protected] dubchak%lcbvax.hepnet @lbl.gov [email protected] factor are identical in the topology of the chain fold and similar in overall structure, yet the two proteins Abstract are dissimilar in sequence with less than 20%identical A methodof quantitative comparisonof two classifica- residues (Sander & Schneider 1991). Opposite example tions rules applied to protein folding problemis pre- sented. Classification of proteins based on sequence (Kabsch & Sander 1984): octapeptides from subtilisin homologyand based on amino acid composition were (2SBT) and imInunoglobulin (3FAB) are dissimilar compared and analyzed according to this approach. structure yet 75%identical in sequences. The main ob- The coefficient of correlation betweenthese classifica- stacle to developmentof strict criteria for calculation tion methods and the procedure of estimation of ro- of homologythreshold is the limited size of empirical bustness of the coefficient are discussed. database. Our study is based on the classification scheme 1 Introduction 3D_ALIof Pascarella and Argos (Pascarella & Argos One of the most powerful methods of protein structure 1992) that merges protein structures and sequence in- prediction is the model building by homology(Hilbert formation. It classifies the majority of the knownX-ray et al. 1993). Chothia and Lesk (Chothia & Lesk 1986) three-dimensional (3D) structures (254 proteins suggested that if two sequences can be aligned with protein domains) into 83 folding classes, 38 of them 50%or greater residue identity they have a similar fold. having two or more representatives, and the other 45 This threshold of 50%is usually used as a "safe defini- containing only a single protein example. This group- tion of sequence homology" (Pascarella & Argos 1992) ing is based on a structural superposition amongpro- and in conventional opinion grants a reasonable confi- tein structures with a similar main-chain fold, either dence that a protein sequence has chain conformation performed by the authors or collected from the litera- of the template excluding less conserved regions. Note ture. that in biology, homology implies an evolutionary re- Sequences from protein primary structure data bank lationship which is not measurable, but in this paper, (SWISSPROT)are associated with all 254 3D struc- according to Pascarella & Argos 1992, we use this term tures providing that they have not less than 50%se- to denote some measure of sequence similarity. quence homology and at least 50% of 3D structure But it was shown that structure information can residues were alignable (Sander & Schneider 1991). be transferred to homologous proteins only when se- Each of them is labeled by the number of one of 83 quence similarity is high and aligned fragments are long classes that includes a protein with maximumhomol- (Sander & Schneider 1991). It is known that homolo- ogy. This labeling can be considered as a classification gous proteins can have completely different 3D struc- procedure based on sequence homology. tures. For example, ras p21 protein and elongation Another simple measure of protein similarity often 1First author gratefully acknowledgesthe support of used is the distance function in the 20-dimensional vec- RUTCORand DIMACS. tor space of amino acid composition. Several groups 2Thesecond author is workingat the Structural Biology (Nakashima e~ al. 1986; Chou 1989; Dubchak et al. Division of LawrenceBerkeley Laboratory (Division Direc- 1993) have shown that the amino acid composition tor Prof. Sung-HouKim), supported by US Department of of a protein provides significant correlation with its Energy. SThe third author gratefully acknowledges the sup- structural class, and a numberof studies were devoted port of DIMACS(grant NSF-CCR-91-1999) and RUTCOR to a quantitative description of this relationship. In (grant F49620-93-1-0041). all these studies, a protein is characterized as a vec- 240 ISMB-95 tor of 20-dimensional space where each of its compo- Thesecond dataset, used as ~es~ingset~ contains 2338 nent is defined by the composition of one of 20 amino proteinsequences. This set, disjoint from the training acids. Recognition schemes based on percent composi- set,is the subsetof all the sequencesof the SWIS- tion are fairly effective for simple classifications where SPROT database,whose homology with at leastone proteins are described in terms of the following struc- of the254 sequences of thetraining set is greaterthan tural classes: all a, allfl, c~+~, a/fl, and irregular. It is or equalto 50%.For eachof these2338 sequences, the obvious that the difficulty of recognition grows rapidly followingitems are available: with the number of classes. Even in the distinction between a +/3 and a/fl classes, serious problems exist . completesequence of aminoacids; because parameter vectors of these types of structure ¯ to the the trainingset are located too close in the parameter space. That is a pointer sequence in which hasthe largesthomology with this sequence; why amino acid composition is considered a crude rep- resentation of a protein sequence and not a tool for ¯ the valueof the homologywith thismost similar protein structure prediction in a context where more sequencein thetraining set. than four structural classes are to be distinguished. In this study, we investigate the correlation between Homology,denoted dh in this work,is a real num- two classifications of protein sequences with unknown ber between0 and 1 showinga proportionof homol- structure, which are obtained by the same simplest ogousresidues in a sequence.Besides homology, we nearest neighbor association procedure (Duda & Hart willstudy simpler measures of similaritybetween se- 1973). These two classifications differ only in the mea- quences,based only on the rate of each ~mlnoacid sure of similarity of proteins: one is based on homology, in thesequence, and independent of therelative posi- while the other uses a distance in the space of amino tionof theseamino acids. The compositionof a protein acid composition. Wedefine and calculate correlation sequenceis definedas a 20-dimensionalvector of coeffi- coefficient between them and propose a technique to cientsin [0,1] indicatingthe rate (number of instances estimate the robustness of this coefficient of correla- of theacid divided by thetotal length of thesequence) tion. High correlation between these two classifications of eachamino acid in the sequence.Very natural dis- would show a hidden power of amino acid composition tancemeasures on the compositionspace [0, i]2° are for folding class prediction and at the same time would providedby the normsLp and willbe denotedalp: cast some doubt on the application of homologyto this prediction without a careful examination. 20 The remaining part of this paper is divided into - - 3 sections. Section 2 describes the methods and data i----I used in this study. The results of our analysis are re- In thisstudy, we willretain only dl andd½, since the ported in section 3. The last section contains a brief formeris easyto interpret,and the latter provides the discussion. highestcorrelation with dh, as we observedempirically. 2 Material and methods 2.2 Scheme of analysis Thissection describes a methodfor the evaluationof In a first stage,20-dimensional vectors of composition correlationsbetween two measuresof proteinsequence are constructedfor each sequence of trainingand test- similarityas wellas a techniqueto measuretheir ro- ingsets. For any distance measure d, theclosest neigh- bustness,by experimentsbased on two setsof data. bor(according to d) in tr~inlng set is identified for all sequences in the testing set. This operation induces 2.1 Material a new classification of the testing set, where each se- quence is associated with the class of its closest neigh- As it was mentionedin the introduction,our first bor. Note that this procedure, executed here for dl dataset,from now on referredto as ~rainingse~, consistsof 254 proteinsequences with known 3D- and d½, is identical to that performed by Pascarella structure.For eachof the proteinsequences in the and Argos (PascareUa & Argos 1992) for dh. trainingset we have: Next step is the comparison of two different classi- fications based on dh, dl and d~. These comparisons * completesequence of aminoacids; are carried out by the computation of the coe~Ncien~of agreemen~between two classifications, i.e. the number ¯ a labelin {1,...,83}that denotes the classrepre- of times when two classifications agree. More detailed sentingits 3D structure. comparisons containing a table indicating the number Mayoraz 241 of times two classifications agree, versus the intensity other uses, it aims at the extraction of simple logical of each of distance measures, was also performed. patterns able to explain the repartition of the samples The coefficients of agreement between different clas- of a training set between their various classes. Since sifications obtained this way are random variables de- the methodessentially deals with logical variables,
Details
-
File Typepdf
-
Upload Time-
-
Content LanguagesEnglish
-
Upload UserAnonymous/Not logged-in
-
File Pages9 Page
-
File Size-