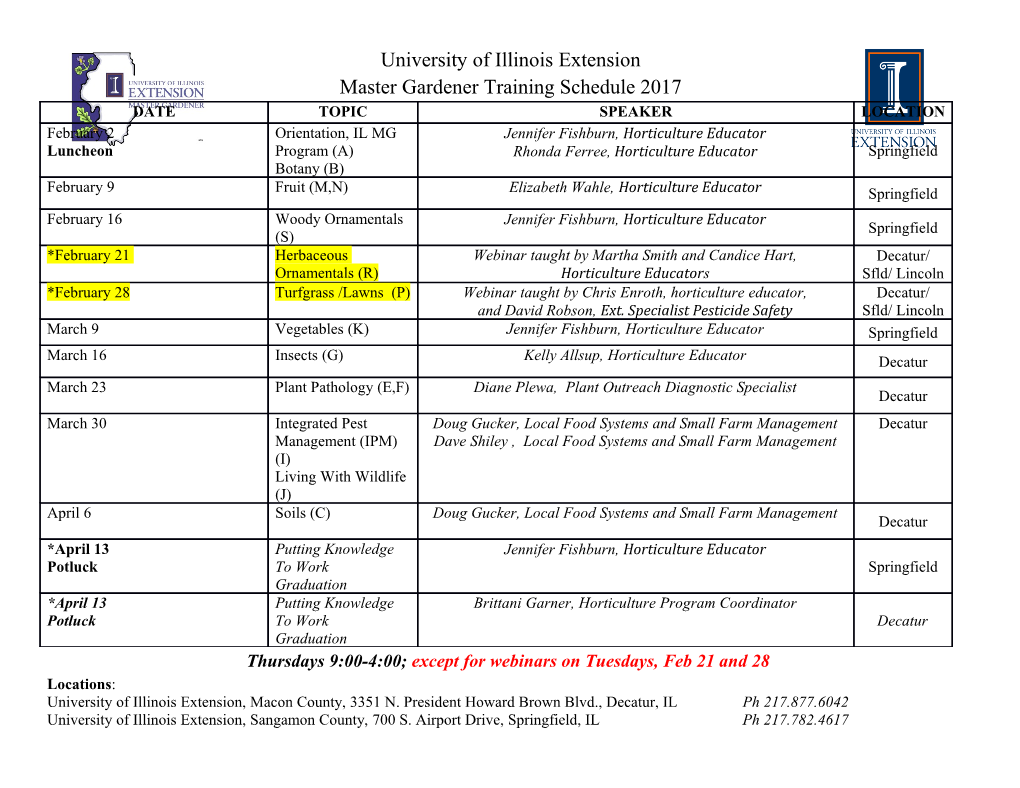
Munich Personal RePEc Archive Partially Linear Models Hardle, Wolfgang and LIang, Hua and Gao, Jiti Humboldt-Universität zu Berlin, University of Rochester, USA, Monash University, Australia 1 September 2000 Online at https://mpra.ub.uni-muenchen.de/39562/ MPRA Paper No. 39562, posted 20 Jun 2012 22:03 UTC PARTIALLY LINEAR MODELS December 23, 1999 Wolfgang H¨ardle Institut f¨urStatistik und Okonometrie¨ Humboldt-Universit¨at zu Berlin D-10178 Berlin, Germany Hua Liang Department of Statistics Texas A&M University College Station TX 77843-3143, USA and Institut f¨urStatistik und Okonometrie¨ Humboldt-Universit¨at zu Berlin D-10178 Berlin, Germany Jiti Gao School of Mathematical Sciences Queensland University of Technology Brisbane 4001, Australia and Department of Mathematics and Statistics The University of Western Australia Perth WA 6907, Australia Electronic Version: http://www.xplore-stat.de/ebooks.html 2 PREFACE In the last ten years, there has been increasing interest and activity in the general area of partially linear regression smoothing in statistics. Many methods and techniques have been proposed and studied. This monograph hopes to bring an up-to-date presentation of the state of the art of partially linear regression techniques. The emphasis of this monograph is on methodologies rather than on the theory, with a particular focus on applications of partially linear regression techniques to various statistical problems. These problems include least squares regression, asymptotically efficient estimation, bootstrap resampling, censored data analysis, linear measurement error models, nonlinear measurement models, nonlinear and nonparametric time series models. We hope that this monograph will serve as a useful reference for theoretical and applied statisticians and to graduate students and others who are interested in the area of partially linear regression. While advanced mathematical ideas have been valuable in some of the theoretical development, the methodological power of partially linear regression can be demonstrated and discussed without advanced mathematics. This monograph can be divided into three parts: part one–Chapter 1 through Chapter 4; part two–Chapter 5; and part three–Chapter 6. In the first part, we discuss various estimators for partially linear regression models, establish theo- retical results for the estimators, propose estimation procedures, and implement the proposed estimation procedures through real and simulated examples. The second part is of more theoretical interest. In this part, we construct several adaptive and efficient estimates for the parametric component. We show that the LS estimator of the parametric component can be modified to have both Bahadur asymptotic efficiency and second order asymptotic efficiency. i ii In the third part, we consider partially linear time series models. First, we propose a test procedure to determine whether a partially linear model can be used to fit a given set of data. Asymptotic test criteria and power investigations are presented. Second, we propose a Cross-Validation (CV) based criterion to select the optimum linear subset from a partially linear regression and establish a CV selection criterion for the bandwidth involved in the nonparametric ker- nel estimation. The CV selection criterion can be applied to the case where the observations fitted by the partially linear model (1.1.1) are independent and iden- tically distributed (i.i.d.). Due to this reason, we have not provided a separate chapter to discuss the selection problem for the i.i.d. case. Third, we provide recent developments in nonparametric and semiparametric time series regression. This work of the authors was supported partially by the Sonderforschungs- bereich 373 “Quantifikation und Simulation Okonomischer¨ Prozesse”. The second author was also supported by the National Natural Science Foundation of China and an Alexander von Humboldt Fellowship at the Humboldt University, while the third author was also supported by the Australian Research Council. The second and third authors would like to thank their teachers: Professors Raymond Carroll, Guijing Chen, Xiru Chen, Ping Cheng and Lincheng Zhao for their valu- able inspiration on the two authors’ research efforts. We would like to express our sincere thanks to our colleagues and collaborators for many helpful discussions and stimulating collaborations, in particular, Vo Anh, Shengyan Hong, Enno Mammen, Howell Tong, Axel Werwatz and Rodney Wolff. For various ways in which they helped us, we would like to thank Adrian Baddeley, Rong Chen, An- thony Pettitt, Maxwell King, Michael Schimek, George Seber, Alastair Scott, Naisyin Wang, Qiwei Yao, Lijian Yang and Lixing Zhu. The authors are grateful to everyone who has encouraged and supported us to finish this undertaking. Any remaining errors are ours. Berlin, Germany Wolfgang H¨ardle Texas, USA and Berlin, Germany Hua Liang Perth and Brisbane, Australia Jiti Gao Symbols and Notation The following notation is used throughout the monograph. a.s. almost surely (that is, with probability one) i.i.d. independent and identically distributed the identity matrix of order p CLTF central limit theorem LIL law of the iterated logarithm MLE maximum likelihood estimate Var(ξ) the variance of ξ N(a, σ2) normal distribution with mean a and variance σ2 U(a, b) uniform distribution on (a, b) def= denote L convergence in distribution −→ P convergence in probability −→ X (X1,...,Xn) Y (Y1,...,Yn) T (T1,...,Tn) ω ( ) or ω∗ ( ) weight functions nj · nj · ST (S ,..., S ) with S = S n ω (T )S , 1 n i i − j=1 nj i j where Si represents a random variable or a function. e e e e P G (g ,..., g )T with g = g(T ) n ω (T )g(T ). 1 n i i − j=1 nj i j ξn = Op(ηn) P ξn M ηn <ζ P f fore{| each|≥eζ >| 0,|} somee M and large enough n ξ = o (η ) P ξ ζ η 0 for each ζ > 0 n p n {| n|≥ | n|} → ξn = op(1) ξn converges to zero in probability Op(1) stochastically bounded ST the transpose of vector or matrix S 2 T S⊗ SS 1 ij S− =(s )p p the inverse of S =(sij)p p × × Φ(x) standard normal distribution function φ(x) standard normal density function For convenience and simplicity, we always let C denote some positive constant which may have different values at each appearance throughout this monograph. iii iv Contents Preface i Symbols and Notation iii List of Figures ix 1 INTRODUCTION 1 1.1 Background, History and Practical Examples ............ 1 1.2 The Least Squares Estimators .................... 10 1.3 Assumptions and Remarks ...................... 11 1.4 The Scope of the Monograph ..................... 14 1.5 The Structure of the Monograph ................... 14 2 ESTIMATION OF THE PARAMETRIC COMPONENT 21 2.1 Estimation with Heteroscedastic Errors ............... 21 2.1.1 Introduction .......................... 21 2.1.2 Estimation of the Non-constant Variance Functions .... 25 2.1.3 Selection of Smoothing Parameters ............. 28 2.1.4 Simulation Comparisons ................... 29 2.1.5 Technical Details ....................... 32 2.2 Estimation with Censored Data ................... 35 2.2.1 Introduction .......................... 35 2.2.2 Synthetic Data and Statement of the Main Results .... 36 2.2.3 Estimation of the Asymptotic Variance ........... 40 2.2.4 A Numerical Example .................... 40 2.2.5 Technical Details ....................... 41 2.3 Bootstrap Approximations ...................... 44 v vi CONTENTS 2.3.1 Introduction .......................... 44 2.3.2 Bootstrap Approximations .................. 45 2.3.3 Numerical Results ...................... 46 3 ESTIMATION OF THE NONPARAMETRIC COMPONENT 49 3.1 Introduction .............................. 49 3.2 Consistency Results .......................... 50 3.3 Asymptotic Normality ........................ 53 3.4 Simulated and Real Examples .................... 55 3.5 Appendix ............................... 57 4 ESTIMATION WITH MEASUREMENT ERRORS 61 4.1 Linear Variables with Measurement Errors ............. 61 4.1.1 Introduction and Motivation ................. 61 4.1.2 Asymptotic Normality for the Parameters ......... 62 4.1.3 Asymptotic Results for the Nonparametric Part ...... 64 4.1.4 Estimation of Error Variance ................ 64 4.1.5 Numerical Example ...................... 65 4.1.6 Discussions .......................... 67 4.1.7 Technical Details ....................... 68 4.2 Nonlinear Variables with Measurement Errors ........... 72 4.2.1 Introduction .......................... 72 4.2.2 Construction of Estimators .................. 73 4.2.3 Asymptotic Normality .................... 74 4.2.4 Simulation Investigations ................... 75 4.2.5 Technical Details ....................... 78 5 SOME RELATED THEORETIC TOPICS 83 5.1 The Laws of the Iterated Logarithm ................. 83 5.1.1 Introduction .......................... 83 5.1.2 Preliminary Processes .................... 84 5.1.3 Appendix ........................... 86 5.2 The Berry-Esseen Bounds ...................... 88 5.2.1 Introduction and Results ................... 88 5.2.2 Basic Facts .......................... 89 CONTENTS vii 5.2.3 Technical Details ....................... 93 5.3 Asymptotically Efficient Estimation ................. 100 5.3.1 Motivation ........................... 100 5.3.2 Construction of Asymptotically Efficient Estimators . 101 5.3.3 Four Lemmas ......................... 103 5.3.4 Appendix ........................... 106 5.4 Bahadur Asymptotic Efficiency ................... 110 5.4.1 Definition ........................... 110 5.4.2 Tail Probability ........................ 112
Details
-
File Typepdf
-
Upload Time-
-
Content LanguagesEnglish
-
Upload UserAnonymous/Not logged-in
-
File Pages216 Page
-
File Size-