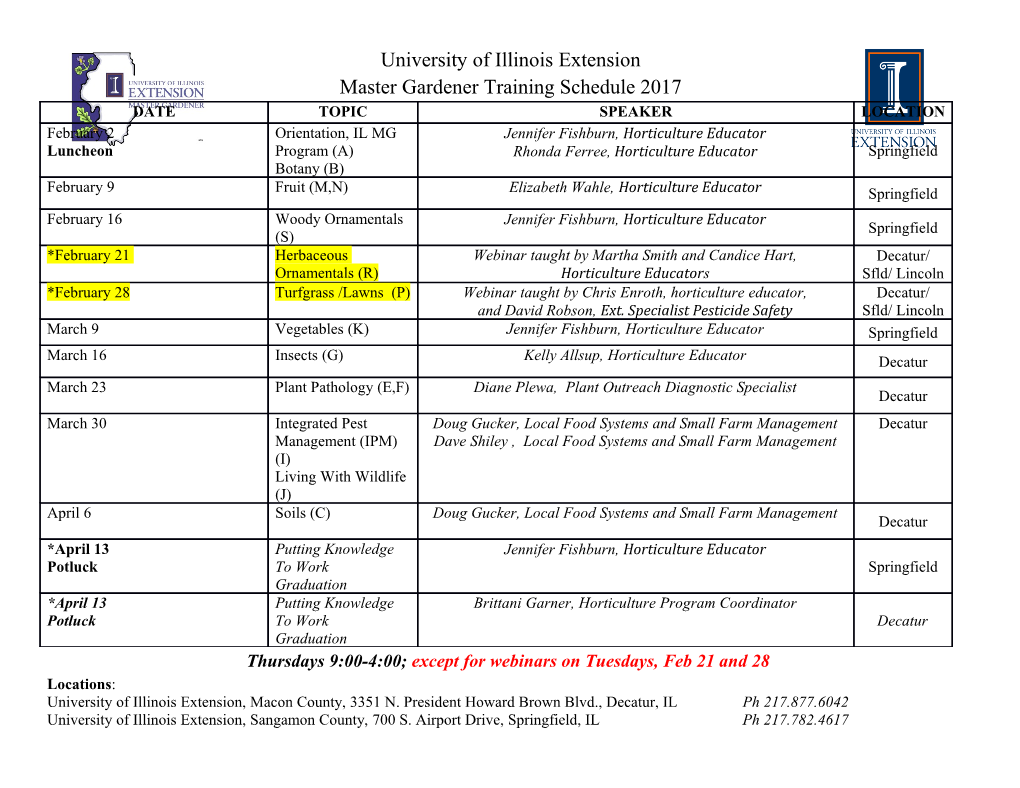
PCAmix PCArot MFAmix Multivariate analysis of mixed data: The PCAmixdata R package Marie Chavent In collaboration with: Vanessa Kuentz, Amaury Labenne, Beno^ıt Liquet, J´er^omeSaracco University of Bordeaux, France Inria Bordeaux Sud-Ouest, CQFD Team Irstea, UR ADBX, cestas, France The University of Queensland, Australia UseR!2015 June 30 - July 3, Aalborg, Denmark PCAmix PCArot MFAmix Multivariate analysis of a mixture of numerical and categorical data Three main functions: Function PCAmix for principal component analysis (PCA) of mixed data. ,! Includes standard PCA and MCA (multiple component analysis) as special cases. Function PCArot for orthogonal rotation in PCAmix. ,! Includes standard varimax rotation and rotation in MCA as special cases. Function MFAmix for multiple factor analysis (MFA) for multiple-table mixed data. https://github.com/chavent/PCAmixdata UseR!2015 June 30 - July 3, Aalborg, Denmark PCAmix A real data example PCArot The PCAmix method MFAmix Principal component prediction Outline 1 PCAmix 2 PCArot 3 MFAmix UseR!2015 June 30 - July 3, Aalborg, Denmark PCAmix A real data example PCArot The PCAmix method MFAmix Principal component prediction Principal component analysis of mixed data Several implementations already in R: Function FAMD in the R package FactoMineR. ,! Implements the method designed by Pag`es(2004). Function dudi.mix in the R package ade4. ,! Implements the method of Hill & Smith (1976). Function PCAmix in the R package PCAmixdata. ,! Implements a single PCA with metrics based on a GSVD of preprocessed data. ) Three different coding scheme but identical numerical results. UseR!2015 June 30 - July 3, Aalborg, Denmark PCAmix A real data example PCArot The PCAmix method MFAmix Principal component prediction A real data example The gironde data are available in the package library(PCAmixdata) data(gironde) They characterize living conditions in Gironde, a southwest region in France. 542 cities are described with 27 variables separated into 4 groups (Employment, Housing, Services, Environment). ,! Four datatables UseR!2015 June 30 - July 3, Aalborg, Denmark PCAmix A real data example PCArot The PCAmix method MFAmix Principal component prediction A mixed data type example The datatable housing is mixed. ,! 3 numerical and 2 categorical variables. housing <- gironde$housing head(housing) ## density primaryres owners houses council ## ABZAC 132 89 64 inf 90% sup 5% ## AILLAS 21 88 77 sup 90% inf 5% ## AMBARES 532 95 66 inf 90% sup 5% ## AMBES 101 94 67 sup 90% sup 5% ## ANDERNOS 552 62 72 inf 90% inf 5% ## ANGLADE 64 81 81 sup 90% inf 5% UseR!2015 June 30 - July 3, Aalborg, Denmark PCAmix A real data example PCArot The PCAmix method MFAmix Principal component prediction Two data sets: ,! a numerical data matrix X1 of dimension 542 × 3. ,! a categorical data matrix X2 of dimension 542 × 2. split<-splitmix(housing) X1<-split$X.quanti X2<-split$X.quali head(X1) head(X2) ## density primaryres owners ## houses council ## ABZAC 132 89 64 ## ABZAC inf 90% sup 5% ## AILLAS 21 88 77 ## AILLAS sup 90% inf 5% ## AMBARES 532 95 66 ## AMBARES inf 90% sup 5% ## AMBES 101 94 67 ## AMBES sup 90% sup 5% ## ANDERNOS 552 62 72 ## ANDERNOS inf 90% inf 5% ## ANGLADE 64 81 81 ## ANGLADE sup 90% inf 5% UseR!2015 June 30 - July 3, Aalborg, Denmark PCAmix A real data example PCArot The PCAmix method MFAmix Principal component prediction The PCAmix method An simple algorithm in three main steps 1 Preprocessing step. 2 GSVD (Generalized Singular Value Decomposition) step. 3 Scores processing step. Some notations: - Let X1 be a n × p1 numerical data matrix. - Let X2 be a n × p2 categorical data matrix. - Let m be the total number of categories. UseR!2015 June 30 - July 3, Aalborg, Denmark PCAmix A real data example PCArot The PCAmix method MFAmix Principal component prediction Preprocessing step 1 Build a numerical data matrix Z = (Z1jZ2) of dimension n × (p1 + m) with: ,! Z1 the standardized version of the matrix X1. ,! Z2 the centered indicator matrix of the levels of X2. 2 Build the diagonal matrix N of the weights of the rows. 1 ,! The n rows are weighted by n . 3 Build the diagonal matrix M of the weights of the columns. ,! The p1 first columns are weighted by1. n ,! The m last columns are weighted by , with ns the number of ns observations with level s. ,! The total variance is p1 + m − p2. UseR!2015 June 30 - July 3, Aalborg, Denmark PCAmix A real data example PCArot The PCAmix method MFAmix Principal component prediction GSVD step The GSVD (Generalized Singular Value Decomposition) of Z with the metrics N and M gives the decompositon Z = UDVt (1) where p p - D = diag( λ1;:::; λr ) is the r × r diagonal matrix of the singular values of ZMZt N and Zt NZM, and r denotes the rank of Z; - U is the n × r matrix of the first r eigenvectors of ZMZt N t such that U NU = Ir ; - V is the p × r matrix of the first r eigenvectors of Zt NZM t such that V MV = Ir . UseR!2015 June 30 - July 3, Aalborg, Denmark PCAmix A real data example PCArot The PCAmix method MFAmix Principal component prediction Scores processing step 1 The set of factor scores for rows is computed as: F = UD: ,! Principal Component scores 2 The set of factor scores for colums is computed as: A = MVD: ,! In standard PCA: A = VD. UseR!2015 June 30 - July 3, Aalborg, Denmark PCAmix A real data example PCArot The PCAmix method MFAmix Principal component prediction The R function args(PCAmix) ## function (X.quanti = NULL, X.quali = NULL, ndim = 5, rename.level = FALSE, ## weight.col = NULL, weight.row = NULL, graph = TRUE) ## NULL PCAmix(X.quanti=X1,X.quali=X2,ndim=2) ## ## Call: ## PCAmix(X.quanti = X1, X.quali = X2, ndim = 2) ## ## Method = Principal Component of mixed data (PCAmix) ## ## ## name description ## [1,] "$eig" "eigenvalues of the principal components (PC) " ## [2,] "$ind" "results for the individuals (coord,contrib,cos2)" ## [3,] "$quanti" "results for the quantitative variables (coord,contrib,cos2)" ## [4,] "$levels" "results for the levels of the qualitative variables (coord,contrib,cos2)" ## [5,] "$quali" "results for the qualitative variables (contrib,relative contrib)" ## [6,] "$sqload" "squared loadings" ## [7,] "$coef" "coef of the linear combinations defining the PC" UseR!2015 June 30 - July 3, Aalborg, Denmark PCAmix A real data example PCArot The PCAmix method MFAmix Principal component prediction Principal Component scores of the observations obj <- PCAmix(X.quanti=X1,X.quali=X2,ndim=2) head(obj$ind$coord) plot(obj,choice="ind") ## dim 1 dim 2 ## ABZAC 2.36 0.024 Individuals component map ## AILLAS -0.88 0.123 BOUSCATCENONTALENCE BORDEAUX 2 TAILLAN−MEDOC ## AMBARES 2.62 0.800 CARIGNAN−DE−BORDEAUXTRESSESIZON CARBON−BLANCSAINT−ANTOINELORMONT GAURIAGUETCAMBLANES−ET−MEYNACMARCHEPRIMECESTASSAINT−LOUBES VILLENAVE−D'ORNONEYSINES BEGLES SAINT−GENIS−DU−BOISSAINT−GENES−DE−LOMBAUDSAINT−AUBIN−DE−MEDOCPIAN−MEDOCPOMPIGNACBONNETANSAINT−SELVELUDON−MEDOCLOUPESTARNESROAILLANMONTUSSANCENACSAINT−CAPRAIS−DE−BORDEAUXSAINTE−HELENECOIMERESVERACPEUJARDBREDEARTIGUES−PRES−BORDEAUXPAREMPUYREHAILLANBRUGESPESSACFLOIRAC SAINT−SAUVEUR−DE−PUYNORMANDSAINT−GENES−DE−FRONSACSAINT−MICHEL−DE−RIEUFRETAYGUEMORTE−LES−GRAVESSAINT−LAURENT−D'ARCEBEYCHAC−ET−CAILLAUCADARSACSAINT−GERMAIN−DU−PUCHCADILLAC−EN−FRONSADAISSAINT−SEVESALLEBOEUFCUBNEZAISMARTILLACARSACCAMARSACAVENSANMARSASPERISSACVIRSACBEAUTIRANCANTENACCADAUJACVILLEGOUGEARTIGUES−DE−LUSSACQUINSACMAZERESSAINT−MEDARD−EN−JALLESAMBESMARTIGNAS−SUR−JALLEYVRAC GRADIGNANMERIGNAC SAINT−PARDON−DE−CONQUESCABANAC−ET−VILLAGRAINSLANDE−DE−FRONSACSAINT−SULPICE−ET−CAMEYRACSAVIGNAC−DE−L'ISLESAINT−MORILLONCROIGNONTABANACCUBZAC−LES−PONTSPOUTBONZACAUBIACBERTHEZSAINT−MARIENSSALAUNESCOMPSFOURSNOAILLACCURSANSAINT−COMESAUCATSCEZACVIRELADEBARONFONTETCAMBESMIOSSAINT−JEAN−D'ILLACSAINT−PIERRE−D'AURILLACSAINT−SEURIN−DE−CURSACLEOGNANBLANQUEFORTAMBARESBASSENS ## AMBES 0.93 0.919 SAINT−LAURENT−DU−BOISSAINT−CIERS−D'ABZACSAINT−MARTIN−DU−BOISSAINTE−FOY−LA−LONGUESAINT−GERMAIN−DE−LA−RIVIERECIVRAC−DE−BLAYELOUPIAC−DE−LA−REOLEPRIGNAC−ET−MARCAMPSFOSSES−ET−BALEYSSACMARCENAISLEOGEATSSAINT−VINCENT−DE−PAULBROUQUEYRANSAINT−PIERRE−DE−MONSSAINT−DENIS−DE−PILEFALEYRASJUGAZANLAROQUEBLESIGNACCASTRES−GIRONDENERIGEANBLAIGNACMARANSINSAMONACCAPIANSOUSSANSCANTOISSABLONSSAVIGNACSADIRACSAINT−MAIXANTSALIGNACDAIGNACMOUILLACBIEUJACPEINTURESFARGUESSAINT−GERVAISARBANATSTEMPLESAINT−AVIT−SAINT−NAZAIRESAINT−MEDARD−D'EYRANSSAINT−SULPICE−DE−FALEYRENSTOURNELAVAZANSAINTE−EULALIELANDIRASLATRESNEBOULIACRIONS PODENSAC SAINTE−FOY−LA−GRANDE SAINT−PHILIPPE−DU−SEIGNALSAINT−LAURENT−DU−PLANSAINT−GIRONS−D'AIGUEVIVESSAINT−MAGNE−DE−CASTILLONSAINT−ROMAIN−LA−VIRVEEPRIGNAC−EN−MEDOCTIZAC−DE−CURTONSAINT−MARTIN−DE−SESCASLUGON−ET−L'ILE−DU−CARNAYLIGNAN−DE−BORDEAUXSAINT−SAUVEURPIAN−SUR−GARONNESAINT−AUBIN−DE−BLAYEVILLENAVE−DE−RIONSLALANDE−DE−POMEROLMOMBRIERBELLEBATPORCHERESAUBIE−ET−ESPESSASCESSACCAMPS−SUR−L'ISLEESSEINTESSAINT−MAGNELISTRAC−DE−DUREZEBERSONSOULIGNACGUILLACSAINT−LOUBERTTEUILLACMOURENSLESTIAC−SUR−GARONNECASTELVIELESPIETSAINT−MARTIALOMETSAUMOSLARUSCADEMOULONLABARDEARBISARVEYRESBAURECHSAINT−LOUIS−DE−MONTFERRANDPORTETSEGLISOTTES−ET−CHALAURESBRANNENSHAUXSAUVELANSACSAINT−CAPRAIS−DE−BLAYEAUROSFARGUES−SAINT−HILAIRECASTELNAU−DE−MEDOCRAUZANBIGANOSPUGNACCANEJANTOULENNECREON SAINT−HILAIRE−DE−LA−NOAILLESAINT−AUBIN−DE−BRANNEPETIT−PALAIS−ET−CORNEMPSSAINT−GENES−DE−BLAYETIZAC−DE−LAPOUYADESAINT−EXUPERYPUJOLS−SUR−CIRONMOULIETS−ET−VILLEMARTINCASTILLON−DE−CASTETSCAMIAC−ET−SAINT−DENISSAINT−TROJANCHAMADELLESAINT−PALAISCUSSAC−FORT−MEDOCMERIGNASISLE−SAINT−GEORGESSAINT−MICHEL−DE−FRONSACGENERACMOULIS−EN−MEDOCMARIMBAULTLIGNAN−DE−BAZASCASSEUILSAINTE−TERRESAINT−LEONSAINT−BRICEPUYBARBANSAINT−CIBARDCLEYRACCOUBEYRACASQUESLAGORCEMADIRACSENDETSCOURPIACMONGAUZYCARDANTAURIACLOUCHATSAILLASILLATSBIRACCARSARCINSCAMIRANGUILLOSFLOUDESLADOSLADAUXSAUVIACLAMOTHE−LANDERRONSAINTE−CROIX−DU−MONTBELIN−BELIETCUDOSBARPMACAUCASTETS−EN−DORTHEBILLAUXSAINT−YZAN−DE−SOUDIACSAINT−ANDRE−DE−CUBZACCERONS
Details
-
File Typepdf
-
Upload Time-
-
Content LanguagesEnglish
-
Upload UserAnonymous/Not logged-in
-
File Pages32 Page
-
File Size-