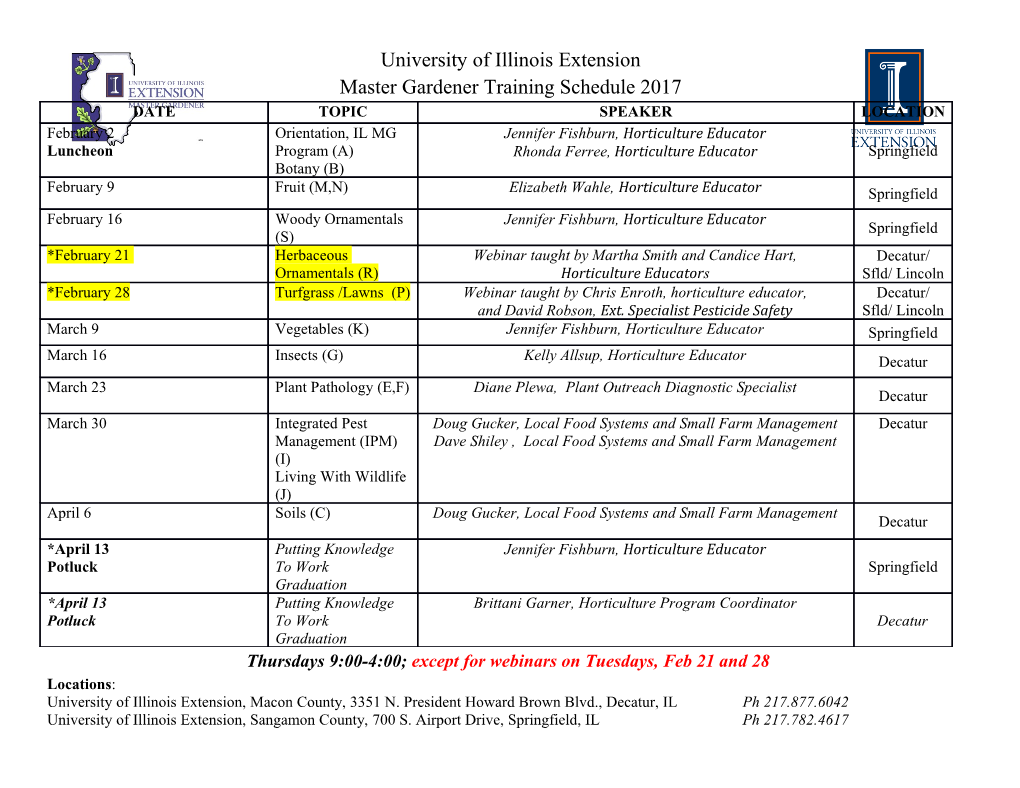
UCAM-CL-TR-848 Technical Report ISSN 1476-2986 Number 848 Computer Laboratory Automatically generating reading lists James G. Jardine February 2014 15 JJ Thomson Avenue Cambridge CB3 0FD United Kingdom phone +44 1223 763500 http://www.cl.cam.ac.uk/ c 2014 James G. Jardine This technical report is based on a dissertation submitted August 2013 by the author for the degree of Doctor of Philosophy to the University of Cambridge, Robinson College. Technical reports published by the University of Cambridge Computer Laboratory are freely available via the Internet: http://www.cl.cam.ac.uk/techreports/ ISSN 1476-2986 Abstract | 3 Abstract This thesis addresses the task of automatically generating reading lists for novices in a scientific field. Reading lists help novices to get up to speed in a new field by providing an expert-directed list of papers to read. Without reading lists, novices must resort to ad- hoc exploratory scientific search, which is an inefficient use of time and poses a danger that they might use biased or incorrect material as the foundation for their early learning. The contributions of this thesis are fourfold. The first contribution is the ThemedPageRank (TPR) algorithm for automatically generating reading lists. It combines Latent Topic Models with Personalised PageRank and Age Adjustment in a novel way to generate reading lists that are of better quality than those generated by state- of-the-art search engines. TPR is also used in this thesis to reconstruct the bibliography for scientific papers. Although not designed specifically for this task, TPR significantly outperforms a state-of-the-art system purpose-built for the task. The second contribution is a gold-standard collection of reading lists against which TPR is evaluated, and against which future algorithms can be evaluated. The eight reading lists in the gold-standard were produced by experts recruited from two universities in the United Kingdom. The third contribution is the Citation Substitution Coefficient (CSC), an evaluation metric for evaluating the quality of reading lists. CSC is better suited to this task than standard IR metrics such as precision, recall, F-score and mean average precision because it gives partial credit to recommended papers that are close to gold-standard papers in the citation graph. This partial credit results in scores that have more granularity than those of the standard IR metrics, allowing the subtle differences in the performance of recommendation algorithms to be detected. The final contribution is a light-weight algorithm for Automatic Term Recognition (ATR). As will be seen, technical terms play an important role in the TPR algorithm. This light-weight algorithm extracts technical terms from the titles of documents without the need for the complex apparatus required by most state-of-the-art ATR algorithms. It is also capable of extracting very long technical terms, unlike many other ATR algorithms. Four experiments are presented in this thesis. The first experiment evaluates TPR against state-of-the-art search engines in the task of automatically generating reading lists that are comparable to expert-generated gold-standards. The second experiment compares the performance of TPR against a purpose-built state-of-the-art system in the task of automatically reconstructing the reference lists of scientific papers. The third experiment involves a user study to explore the ability of novices to build their own reading lists using two fundamental components of TPR: automatic technical term recognition and topic modelling. A system exposing only these components is compared against a state- of-the-art scientific search engine. The final experiment is a user study that evaluates the technical terms discovered by the ATR algorithm and the latent topics generated by TPR. The study enlists thousands of users of Qiqqa, research management software independently written by the author of this thesis. Abstract | 4 Acknowledgements | 5 Acknowledgements I would like to thank my supervisor, Dr. Simone Teufel, for allowing me the room to develop my ideas independently from germination to conclusion, and for dedicating so much time to guiding me through the writing-up process. I thank her for the many interesting and thought-provoking discussions we had throughout my graduate studies, both in Cambridge and in Edinburgh. I am grateful to the Computer Laboratory at the University of Cambridge for their generous Premium Research Studentship Scholarship. Many thanks are due to Stephen Clark and Ted Briscoe for their continued and inspiring work at the Computer Laboratory. I am also grateful to Nicholas Smit, my accomplice back in London, and the hard-working committee members of Cambridge University Entrepreneurs and the Cambridge University Technology and Enterprise Club for their inspiration and support in turning Qiqqa into world-class research software. I will never forget my fellow Robinsonians who made the journey back to university so memorable, especially James Phillips, Ross Tokola, Andre Schwagmann, Ji-yoon An, Viktoria Moltz, Michael Freeman and Marcin Geniusz. Reaching further afield of College, University would not have been the same without the amazing presences of Stuart Barton, Anthony Knobel, Spike Jackson, Stuart Moulder and Wenduan Xu. I am eternally grateful to Maïa Renchon for her loving companionship and support through some remarkably awesome and trying times, to my mother, Marilyn Jardine, for inspiring me to study forever, and to my father, Frank Jardine, for introducing me to my first “thinking machine”. Acknowledgements | 6 Table of Contents | 7 Table of Contents Abstract............................................................................................................................. 3 Acknowledgements .......................................................................................................... 5 Table of Contents ............................................................................................................. 7 Table of Figures .............................................................................................................. 11 Table of Tables ............................................................................................................... 13 Chapter 1. Introduction .............................................................................................. 15 Chapter 2. Related work ............................................................................................. 21 2.1 Information Retrieval ....................................................................................... 21 2.2 Latent Topic Models ........................................................................................ 24 2.2.1 Latent Semantic Analysis ......................................................................... 27 2.2.2 Latent Dirichlet Allocation ....................................................................... 28 2.2.3 Non-Negative Matrix Factorisation (NMF) ............................................. 30 2.2.4 Advanced Topic Modelling ...................................................................... 30 2.3 Models of Authority......................................................................................... 32 2.3.1 Citation Indexes ........................................................................................ 32 2.3.2 Bibliometrics: Impact Factor, Citation Count and H-Index ..................... 34 2.3.3 PageRank .................................................................................................. 34 2.3.4 Personalised PageRank ............................................................................. 36 2.3.5 HITS ......................................................................................................... 41 2.3.6 Combining Topics and Authority ............................................................. 42 2.3.7 Expertise Retrieval ................................................................................... 45 2.4 Generating Reading Lists................................................................................. 45 2.4.1 Ad-hoc Retrieval ...................................................................................... 45 2.4.2 Example-based Retrieval .......................................................................... 46 2.4.3 Identifying Core Papers and Automatically Generating Reviews ............ 46 2.4.4 History of Ideas and Complementary Literature ...................................... 47 2.4.5 Collaborative Filtering.............................................................................. 48 2.4.6 Playlist Generation ................................................................................... 49 2.4.7 Reference List Reintroduction .................................................................. 49 Table of Contents | 8 2.5 Evaluation Metrics for Evaluating Lists of Papers .......................................... 51 2.5.1 Precision, Recall and F-score ................................................................... 51 2.5.2 Mean Average Precision (MAP) .............................................................. 52 2.5.3 Relative co-cited probability (RCP) ......................................................... 53 2.5.4 Diversity ................................................................................................... 54 Chapter 3. Contributions of this Thesis.....................................................................
Details
-
File Typepdf
-
Upload Time-
-
Content LanguagesEnglish
-
Upload UserAnonymous/Not logged-in
-
File Pages164 Page
-
File Size-