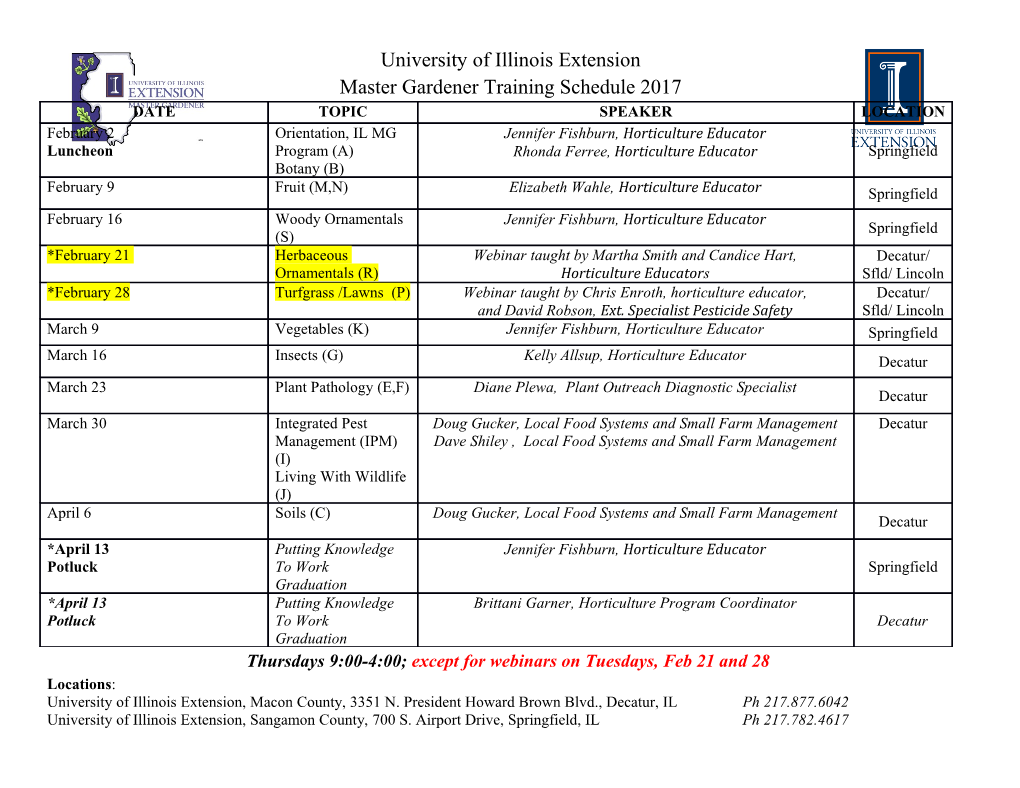
AN ABSTRACT OF THE THESIS OF Jimmy Bell for the degree of Master of Science in Computer Science presented on March 9, 2020. Title: Machine Learning Methods for the Discovery and Analysis of MicroRNAs. Abstract approved: ______________________________________________________ David A. Hendrix MicroRNAs are a highly conserved class of small endogenous RNA, about ~22nt in length, involved in post-transcriptional gene silencing and have prominent roles in disease and development. Though the process of microRNA discovery was once an arduous task, the advent of high throughput sequencing technology has resulted in novel microRNAs being discovered at a rapid rate. Several data-driven pipelines and machine learning-based methods have been devised so that the beginning stages of microRNA discovery can be performed in silico. Despite these efforts, several challenges have persisted in the computational prediction of microRNAs. These challenges include the identification of microRNAs with low expression, proper determination of the precursor span, and the precise labeling of the cleavage sites involved in their biogenesis. This thesis addresses these challenges with two new machine learning-based approaches. MiRWoods improves precursor detection and uses stacked random forests for the sensitive detection of microRNAs. We report that miRWoods has a 10% higher recall of annotated microRNAs when compared with other software. We applied this method to the genomes of human, mouse, Felis catus (cat) and Bos Taurus (cow) and identified hundreds of novel microRNAs in small RNA sequencing datasets. Our novel predictions include a microRNA in an intron of tyrosine kinase 2 (TYK2), that is present in both cat and cow, as well as a family of mirtrons with two instances in the human genome. Our predictions support a more expanded miR-2284 family in the bovine genome, a larger mir-548 family in the human genome, and a larger let-7 family in the feline genome. DeepMirCut is a deep learning approach for identifying cleavage sites within microRNAs. This approach is inspired by site-labeling methods for natural language processing, and can accurately predict how the microRNA processing enzymes Dicer and Drosha cleave the microRNA precursor. ©Copyright by Jimmy Bell March 9, 2020 All Rights Reserved Machine Learning Methods for the Discovery and Analysis of MicroRNAs by Jimmy Bell A THESIS submitted to Oregon State University in partial fulfillment of the requirements for the degree of Master of Science Presented March 9, 2020 Commencement June 2020 Master of Science thesis of Jimmy Bell presented on March 9, 2020 APPROVED: Major Professor, representing Computer Science Head of the School of Electrical Engineering and Computer Science Dean of the Graduate School I understand that my thesis will become part of the permanent collection of Oregon State University libraries. My signature below authorizes release of my thesis to any reader upon request. Jimmy Bell, Author ACKNOWLEDGEMENTS The author expresses sincere appreciation for the countless hours David Hendrix has spent training me, helping me improve my research, and proofreading my writing. I would also like to express my gratitude to Christiane Löhr and Maureen Larson for providing the small RNA sequencing data for cat and performing the PCR experiments on microRNAs found within the Cat genome. I’d also like to thank Massimo Bionaz who provided the small RNA sequencing data for cow and who’s lab performed PCR experiments on microRNAs from the Cow genome. CONTRIBUTION OF AUTHORS Jimmy Bell performed computational analysis presented in this work, including software development, evaluation, and data visualization, and was the primary author of this document. Christiane Löhr and Maureen Larson provided the small RNA sequencing data for cat samples, did a PCR analysis on identified cat microRNAs, and provided the written section explaining the methodology of the PCR experiment. Michelle Kutzler and Massimo Bionaz carried out the tissue collection for cow. Massimo Bionaz provided the small RNA sequencing data for cow samples, did a PCR analysis on identified cow microRNAs and provided the written section explaining the methodology of the PCR experiment performed. David Hendrix directed the research, assisted in developing the methodology, and helped with proofreading and rewriting. TABLE OF CONTENTS Page 1 Introduction ............................................................................................................... 1 1.1 Biological Background .............................................................................. 1 1.2 Early microRNA discovery tools ............................................................... 1 1.3 Machine Learning and Feature-based Prediction ...................................... 2 1.4 Deep learning approaches to microRNA analysis ..................................... 3 1.5 Remaining Challenges to microRNA Discovery ....................................... 4 2 miRWoods: Enhanced precursor detection and stacked random forests for the sensitive detection of microRNAs ................................................................................ 7 2.1 Abstract ...................................................................................................... 7 2.2 Introduction ................................................................................................ 7 2.3 Results ........................................................................................................ 9 2.3.1 Overview of strategy behind miRWoods .................................... 9 2.3.2 Stacked random forest approach ............................................... 10 2.3.3 Accurate mapping of hairpin precursor span ............................ 11 2.3.4 Evaluation of prediction performance ...................................... 12 2.3.5 Novel microRNA predictions in the feline genome ................. 14 2.3.6 Novel microRNA predictions in the bovine genome ................ 16 2.3.7 Novel Predictions in the Bovine miR-2284 Precursor Family . 17 2.3.8 Discovery of novel miR families .............................................. 18 2.4 Discussion ................................................................................................ 18 2.5 Methods.................................................................................................... 20 2.5.1 Ethics Statement ........................................................................ 20 2.5.2 Tissue samples small RNA sequencing .................................... 20 2.5.3 The miRWoods pipeline ........................................................... 21 2.5.4 Mature product random forest .................................................. 22 2.5.5 Hairpin precursor span optimization ......................................... 22 2.5.6 Hairpin precursor random forest ............................................... 23 2.5.7 Training, tuning and model selection ........................................ 25 2.5.8 Comparisons with other tools ................................................... 26 TABLE OF CONTENTS (Continued) Page 2.5.9 Dicer knockdown comparisons ................................................. 28 2.5.10 Validation of bovine and feline microRNA predictions ......... 28 2.5.11 Hierarchical clustering ............................................................ 29 2.5.12 Identification of clusters ......................................................... 29 2.5.13 Identification of novel miR families ....................................... 30 2.6 Tables ....................................................................................................... 30 2.7 Figures...................................................................................................... 35 3 Predicting Dicer and Drosha cleavage sites through deep learning. ....................... 55 3.1 Abstract .................................................................................................... 55 3.2 Introduction .............................................................................................. 55 3.3 Results ...................................................................................................... 57 3.4 Discussion ................................................................................................ 60 3.5 Methods.................................................................................................... 61 3.5.1 Data Preprocessing .................................................................... 61 3.5.2 Hyperparameter Tuning ............................................................ 62 3.5.3 Point Mutation Analysis ........................................................... 62 3.5.4 Identification of Unannotated mature microRNAs ................... 62 3.6 Tables ....................................................................................................... 63 3.7 Figures...................................................................................................... 64 4 Conclusion .............................................................................................................. 73 5 Bibliography ........................................................................................................... 76 LIST OF FIGURES Figure Page Figure 2.1: Outline of miRWoods Pipeline ................................................................ 35 Figure 2.2: Importance of features .............................................................................
Details
-
File Typepdf
-
Upload Time-
-
Content LanguagesEnglish
-
Upload UserAnonymous/Not logged-in
-
File Pages92 Page
-
File Size-