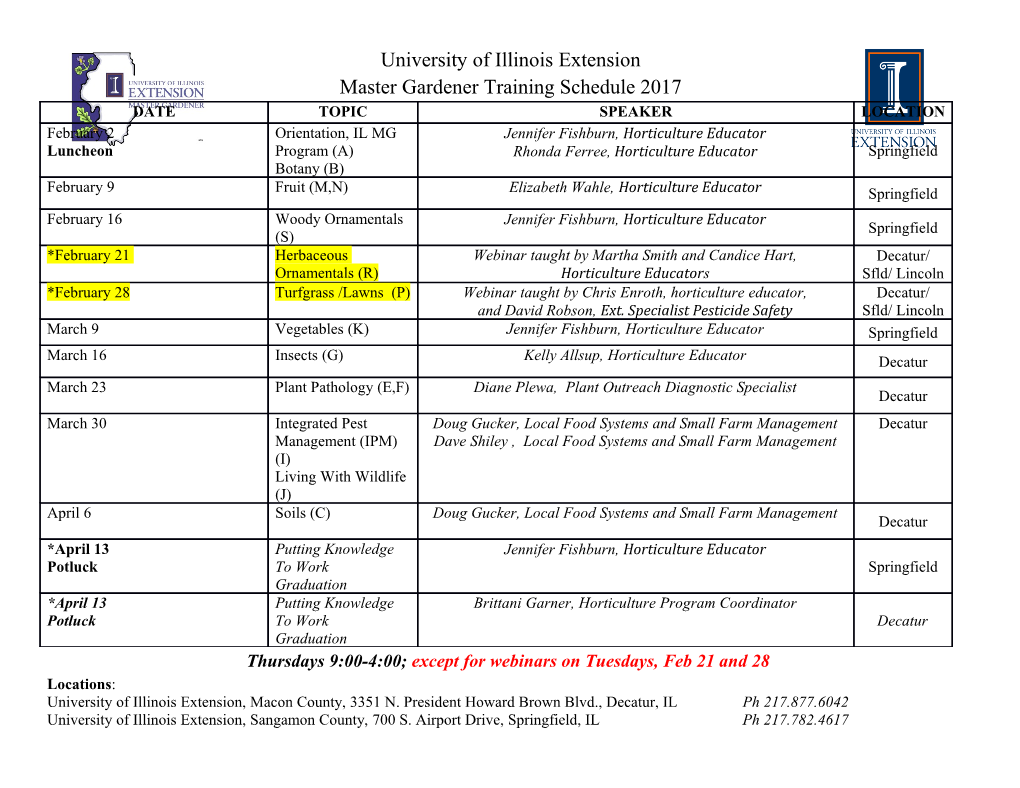
This article has been accepted and published on J-STAGE in advance of copyediting. Content is final as presented. IEICE Electronics Express, Vol.VV, No.NN, 1–6 LETTER A Proposal for Enhancing Training Speed in Deep Learning Models Based on Memory Activity Survey Dang Tuan Kiet1a), Binh Kieu-Do-Nguyen1b), Trong-Thuc Hoang1c), Khai-Duy Nguyen1d), Xuan-Tu Tran2e), and Cong-Kha Pham1f) Abstract forms such as mobile devices or embedded systems [1]. Deep Learning (DL) training process involves intensive computations that However, it is not the case for the training process. The require a large number of memory accesses. There are many surveys on training process is still a data-intensive challenge for many memory behaviors with the DL training. They use well-known profiling tools or improving the existing tools to monitor the training processes. computing systems. It is characterized by millions of para- This paper presents a new approach to profile using a co-operate solution meters with billions of data transactions to compute. There- from software and hardware. The idea is to use Field-Programmable-Gate- fore, besides improving the training algorithms or models, Array memory as the main memory for the DL training processes on a memory access enhancement is another approach to speed computer. Then, the memory behaviors from both software and hardware up such a process. point-of-views can be monitored and evaluated. The most common DL models are selected for the tests, including ResNet, VGG, AlexNet, and DL training is a data-intensive process that requires millions GoogLeNet. The CIFAR-10 dataset is chosen for the training database. The to billions of read/write transactions. For example, the confi- experimental results show that the ratio between read and write transactions gurable parameters during the training processes of VGG-19 is roughly about 3 to 1. The requested allocations are varied from 2-Byte to 64-MB, with the most requested sizes are approximately 16-KB to 64-KB. and ResNet-152 are already 144 and 57 million parameters Based on the statistic, a suggestion was made to improve the training speed [2], respectively. To interact with those multi-million para- using an L4 cache for the Double-Data-Rate (DDR) memory. It can be meters, the number of read/write transactions on the main demonstrated that our recommended L4 cache configuration can improve memory could quickly go up to the scale of multi-billion. the DDR performance by about 15% to 18%. Such an enormous number puts high pressure on the main key words: Deep Learning, Memory, Survey, Training Speed. Classification: Integrated circuits (logic) memory, which tends to be the system’s bottleneck. Many profiling tools were proposed to evaluate the data usage of 1. Introduction DL training processes, such as Nvprof [3], Tegrastats [4], Deep Learning (DL) is widely applied to reform multiple and TensorFlow profiler [5]. Although the collected statis- aspects in today’s world (e.g., image recognition, cognitive tics from these tools can help in many cases [2, 6, 7, 8], assistance, speech recognition, gene analysis, street navi- they are hard to or even cannot provide a complete view gation, etc.). DL applications development generally con- at the physical level, which represents the actual read/write sists of two steps, i.e., training and inference. The central transactions of the Random-Access-Memory (RAM). Fur- issue of inference is now less severe because of recent de- thermore, modern Central-Processing-Units (CPUs) nowa- velopments of Field-Programmable-Gate-Arrays (FPGAs), days only communicate to the main memory via a complex Graphics-Processing-Units (GPUs), and Tensor-Processing- bus system with many layers of caches. Thus, the memory’s Units (TPUs) have made them more potent in small plat- actual behaviors are often hidden, sometimes even inten- tionally due to security reasons, from the software point of 1University of Electro-Communications (UEC), view. 1-5-1 Chofugaoka, Chofu-shi, Tokyo 182-8585, Japan To overcome the issue, a co-operate software-hardware so- 2The Information Technology Institute (VNU-ITI), lution is derived from this paper. The idea is to develop the 144 Xuan Thuy road, Cau Giay dist., Hanoi, Vietnam training processes for several common DL models that run a) [email protected] on a computer but not using the computer’s main RAM. b) [email protected] Instead, an FPGA board having a Double-Data-Rate (DDR) c) [email protected] memory and a Peripheral-Component-Interconnect-express d) [email protected] e) [email protected] (PCIe) connection is used to emulate the computer’s main f) [email protected] RAM. Furthermore, in the FPGA, a monitor module is de- DOI: 10.1587/elex.XX.XXXXXXXX signed to collect the valuable data of the memory at the DOI:Received 10.1587/elex.18.20210252 October 10, 2019 physical level. To be specific, three steps are performed in ReceivedAccepted June October 08, 10,2021 2019 this paper to provide an effective and appropriate suggestion Accepted July 01, 2021 PublicizedPublished July December 09, 2021 31, 2019 on improving memory access. First of all, we determine the 1 CopyrightCopyright ©© 2021 2019 The The Institute Institute of of Electronics, Electronics, Information Information and and Communication Communication Engineers Engineers IEICE Electronics Express, Vol.VV, No.NN, 1–6 most critical aspects that need to be evaluated in memory tioned above, only TensorFlow, PyTorch, and OpenNN are activities. They are the peek allocated memory for a training using a C/C++ core library. However, TensorFlow is heavily loop, the smallest and the biggest requested size in a single python-oriented, and the OpenNN is using 3rd-party APIs allocation, the most and the second most frequent requested for the main computations. As a result, the PyTorch is the sizes of the allocations, the number of read/write transac- most suitable one for the implementation in this paper. More- tions, and the total length of read/write data. Secondly, a over, its C/C++ core library can achieve high-performance co-operate software-hardware system is designed to collect processing [22]. the trivial data at both abstracts (software) and physical (hardware) levels. On the software side, the DL applications 3. Proposed Memory Activity Survey Method are modified to control the hardware and collect a part of the 3.1 Hardware Implementation statistical data. On the hardware side, a monitor module is Fig. 1 shows the overview of the designed monitoring sys- developed to monitor and collect the DDR behaviors at the tem. In the proposed system, the hardware implementation physical level. Furthermore, the monitor module is designed on FPGA is used to emulate the host computer’s main me- in a way to not interfere with the DDR bandwidth. In other mory. The chosen FPGA board needs to have high-speed words, we make sure that the bottleneck of the whole system DDR RAM and a PCIe connection. The FPGA board is is not due to the monitor module itself. Finally, based on the connected to the host computer via PCIe, and the PCIe driver collected data, we perform an analysis and suggest practical is used for the software on the host computer to recognize the solutions to improve memory access performance. FPGA as a RAM. Therefore, the DL programs on the host The remainder of this paper is organized as follows. Section computer can run while using the FPGA’s DDR memory. 2 briefly reviews the most common Convolutional Neural In the FPGA, a monitor module is developed to record the Networks (CNNs) and datasets used in the DL applications. memory usage behaviors at the physical level. Furthermore, Section 3 explains the memory activity survey method. Sec- the software on the host computer is also modified to con- tion 4 shows the experimental results. Section 5 suggests trol the hardware and get the statistics data from the FPGA some remedies to enhance the DDR usage performance. afterward. And finally, section 6 concludes the work. Fig. 2 gives the design idea of the monitor module. As shown in the figure, there are four main components, including 2. Common Deep Learning Models and Datasets probe, controller, collectors, and storage. The probe is also One of the most common tasks encompassed by object shown in Fig. 1; it records the signal activities of the RAM recognition is image classification which involves predic- controller. Then, the controller part in the monitor module ting the type of an object in images. So far, CNN, a class analyses the received data and pushes it to the corresponding of DL neural networks, is the best learning algorithm for collector. The collectors keep track of the RAM’s behaviors, understanding image content. It has shown exemplary per- such as the number of read/write cycles, the number of formances in various image processing applications such as bytes (or requested size), and the total number of read/write segmentation, classification, detection, and retrieval-related transactions. Finally, all the recorded data are mapped to a tasks [9]. A typical CNN is often constructed by multiple storage that can be read back by the host computer. layers like Convolutional layer (Conv), Rectified Linear Unit (ReLU), pooling layer, Batch Normalization (BN), dropout function, and Fully-Connected layer (FC). Some of these primary layers can be grouped to form a block. Generally, a DL model consists of distinct layers and building blocks stacked in many orders to form various architectures for various purposes [10]. The DL models that used for the survey in this paper are among the most common ones such as ResNet-18, ResNet- 34, ResNet-50, ResNet-101, ResNet-152 [11], AlexNet [12], VGG-16, VGG-19 [13], and GoogLeNet [14].
Details
-
File Typepdf
-
Upload Time-
-
Content LanguagesEnglish
-
Upload UserAnonymous/Not logged-in
-
File Pages6 Page
-
File Size-