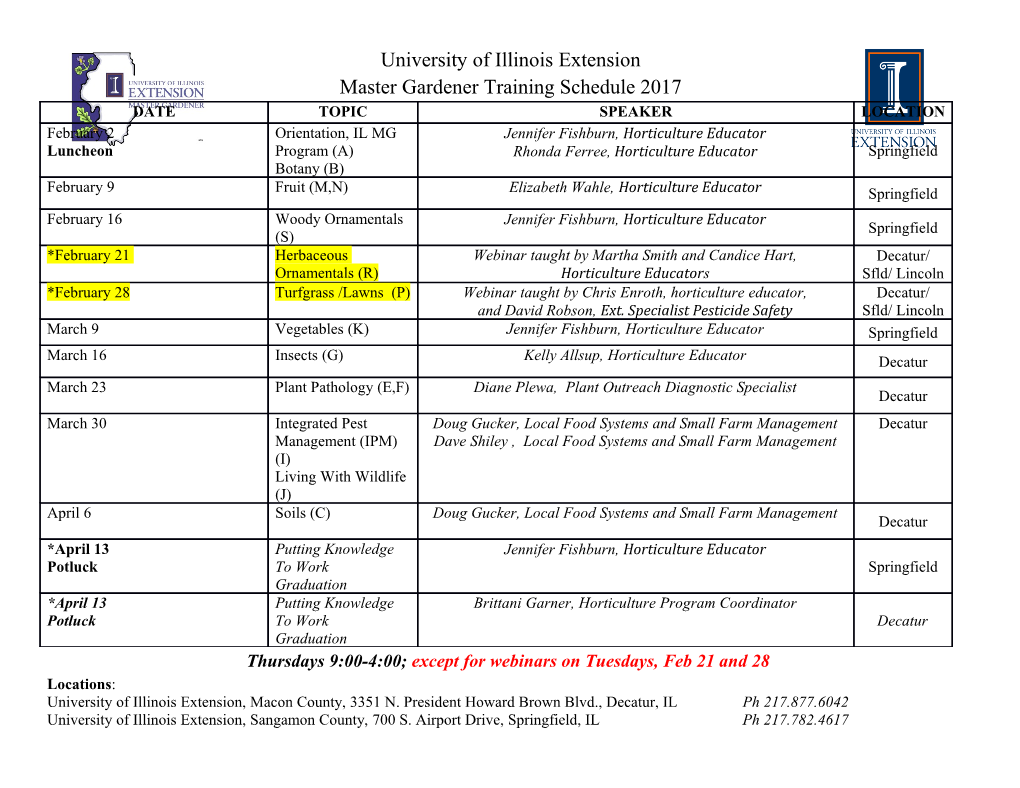
What Makes the Market Tix: A Machine Learning Analysis of the Resale Concert Ticket Market The Harvard community has made this article openly available. Please share how this access benefits you. Your story matters Citation Haglund, George. 2020. What Makes the Market Tix: A Machine Learning Analysis of the Resale Concert Ticket Market. Bachelor's thesis, Harvard College. Citable link https://nrs.harvard.edu/URN-3:HUL.INSTREPOS:37364693 Terms of Use This article was downloaded from Harvard University’s DASH repository, and is made available under the terms and conditions applicable to Other Posted Material, as set forth at http:// nrs.harvard.edu/urn-3:HUL.InstRepos:dash.current.terms-of- use#LAA What Makes The Market Tix A Machine Learning Analysis of The Resale Concert Ticket Market a thesis presented by George Haglund to The Department of Computer Science in partial fulfillment of the requirements for the joint degree of Bachelor of Arts in the subjects of Computer Science and Statistics Harvard University Cambridge, Massachusetts April 2020 © 2020 - George Haglund All rights reserved. Thesis advisor: Kevin Rader George Haglund What Makes The Market Tix Abstract The resale concert ticket market is a largely unexplored domain in terms of rigorous statistical analysis. It is a market in which tickets are sold at multiples of their face value minutes after they are released to the public on the primary market. In this paper, I provide a number of predictive models on data collected from resale ticket websites in order to explain how prices behave in the secondary ticket market. I then introduce parametric models to explain the factors that influence price changes in this market. I am able to use these models to explain how some tickets tend to behave over the course of their life on the secondary market, as well as some factors that influence this behavior. I find that Long Short Term Memory Neural Networks perform extremely well in a predictive capacity for both average and individual ticket prices. The inputs to the parametric models are affected the most by the number of days remaining until a concert andthe population of the city where the concert takes place. These models find that the shape of the trend of ticket prices tends to be similar between concerts, while the starting price of a ticket varies significantly between concerts. The findings ofthis paper shed some light on the confusing secondary market and provide insight for buyers and sellers in both the primary and secondary concert ticket markets. iii Contents 1 Introduction 1 2 Background 4 3 Data 15 3.1 RawData............................. 15 3.1.1 Artist Data . 16 3.1.2 Concert Data . 17 3.1.3 Ticketing Data . 18 3.2 Data Collection . 18 3.2.1 Artist Data Collection . 19 3.2.2 Concert Data Collection . 20 3.2.3 Ticket Data Collection . 21 3.2.4 Avoiding Bans . 22 3.3 Cleaning . 23 3.3.1 Artist Reduction . 23 3.3.2 General Admission . 23 3.3.3 Date Cutoff . 24 3.3.4 Extra Dates . 24 3.3.5 Missing Data . 24 3.3.6 Data Inconsistencies . 24 3.4 Exploratory Data Analysis . 25 iv 3.4.1 Artists . 28 3.4.2 Venues . 29 3.4.3 Tickets . 32 3.4.4 Likelihood Justification . 34 4 Methods 36 4.1 Log Mean Tickets . 36 4.1.1 Linear Regression . 36 4.1.2 Mixed Effects Model . 37 4.1.3 Generalized Additive Model . 38 4.1.4 Random Forest . 38 4.1.5 Auto-Regressive Integrated Moving Average . 39 4.1.6 Long Short-Term Memory Neural Network . 41 4.2 ID Tickets . 43 4.2.1 Likelihood Model . 43 4.3 Model Evaluation . 46 4.3.1 Predictions . 46 4.3.2 Inference . 47 5 Results 48 5.1 Log Mean Tickets . 48 5.1.1 Ticket Level . 49 5.1.2 Venue Level . 52 5.1.3 Artist Level . 53 5.2 ID Tickets . 55 5.2.1 Ticket Level . 55 5.2.2 Venue Level . 57 5.2.3 Artist Level . 60 6 Discussion 65 6.1 Early Trends . 65 6.2 Predictive Power . 66 v 6.3 Variable Contributions . 67 6.4 Affected Parties . 71 6.5 Diagnostics and Decisions . 73 6.6 Next Steps . 75 6.7 Effects of COVID-19 . 76 7 Conclusion 77 References 81 vi Acknowledgments Thank you first and foremost to Kevin Rader, my advisor in this project. He has gently guided me through this year long endeavor, and I am extremely grateful for all the help that he has given me. A special thank you to my family for always supporting me in everything that I pursue and always being there for me. An additional thank you to my sister for her creative help in naming this thesis. Thank you to my friend Ethan for taking me to the Astroworld concert where the idea for this paper had its inception and to my friend Miles for providing his knowledge of the music industry to help turn that idea into a reality. Finally, thank you to my roommates, teammates, and everyone else who encouraged and supported in my journey over the last year. I would also like to apologize to most people mentioned here who had to listen to me talk at length about the small intricacies of how tickets are priced and sold. vii 1 Introduction The music industry is an industry that will likely never go out of style. It has seen incredible growth in recent years due to the popularization of streaming services such as Spotify. There is one area of the music industry, however, that appears to have stagnanted; the quantity of concert tickets sold. According to Pollstar, a magazine that keeps a database of ticket sales and releases a yearly report of its findings, there was a 2.1% drop in total tickets sold between 2017 and 2018, from 23.4 million to 22.9 million [13]. This number does not tell the full story. Pollstar also reports that 2018 was a record setting year for sales, with a ”12% jumpin total gross from last year’s $1.97 billion to a record-setting $2.21 billion” for the top 50 tours worldwide [13]. In its 2018 Media and Entertainment report, Price Waterhouse Cooper found that ”live music ticket sales will increase at a compound annual growth rate (CAGR) of 3.33% from 2018 to 2023, from $21.256bn (projected) in 2018 to $25.036bn in 2023.” [5] The number of tickets 1 sold has dipped, but the total revenue from sales has made a drastic jump. The primary ticket market is raising its prices to keep up with the secondary market. In recent years, the popular music concert ticket market has witnessed a disturbing phenomenon whereby tickets sell out in seconds of release and then reappear on the resale market with astronomical markups. In 2016, the New York Attorney General released a report on the state of ticket scalping in New York. In the report, they ”studied six ticket brokers and found they marked up their tickets an estimated 49% on average, ranging by broker from an average of 15% to 118%.” [18] The reason ticket brokers are able to mark up their prices in this manner is due to the problem of fairness and pricing in the concert ticket industry. Historically, artists have priced their concerts well below what the demand for this good would suggest [10]. These artificially low prices have allowed for rent-seeking behavior, whereby ticket scalpers can buy up tickets at face value and resell the tickets at the market value [10]. The ability of brokers to price tickets at their true value without having to worry about image and reputation has helped the secondary ticket market to grow to between ten and fifteen billion dollar annual volume [4]. As a result of its rapid growth, ”The ticket marketplace has become a fiercely competitive game in which major corporations compete over resale prices with the fan next door, scalpers have a Washington lobbyist and thousands of tickets disappear in a fraction of a second.” [19] Unlike in the primary market, sellers are able to, and frequently do, dynamically alter the prices of their tickets in the time leading up to a concert. Because of this, the secondary market is highly variable. All these factors result in a growing, dynamic marketplace that affects nearly every person who listens to music. Despite the problems in the resale market, there seems to be very little happening to fix it. Some major artists, like Taylor Swift, have tried to institute a dynamic pricing model for their tours [16]. However, the systems that institute these ”dynamic” changes in price tend to be outdated and require significant work on the part of the ticket seller. Previous solutions have been flawed possibly because there is little understanding of how this market functions. In his paper ”Rocknomics”, Alan Kreuger notes that ”because scalping is primarily an 2 underground activity, little systematic empirical analysis has been done on secondary ticket markets” [6]. What analysis has been performed on the concert ticket market is largely made up of economic theory regarding how tickets are priced and sold in the primary market. The goal of this paper is to introduce a machine learning approach to thelittle understood secondary ticket market. This paper proposes to use machine learning methods and statistical inference in order to perform an analysis on the behavior of tickets in the resale market. Specifically, this paper will look at how ticket prices change over time - from when they go on sale to the day of the concert - as well as what factors influence these changes in price.
Details
-
File Typepdf
-
Upload Time-
-
Content LanguagesEnglish
-
Upload UserAnonymous/Not logged-in
-
File Pages91 Page
-
File Size-