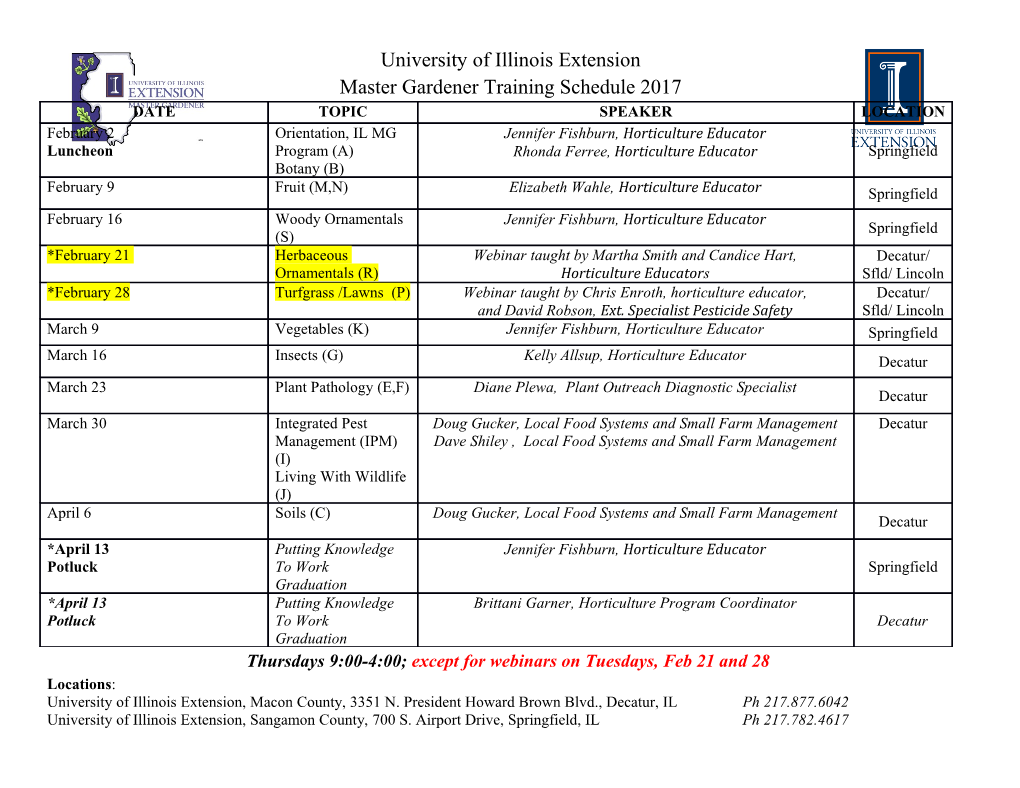
Hot Topics in Clinical Trials Graybill Conference VII Biopharmaceutical Statistics June 11, 2008 Scott Evans, Lingling Li, LJ Wei, Marvin Zelen Program for Quantitative Sciences in Medicine Department of Biostatistics Harvard University Meta Analysis Introduction What is Meta Analysis LJ Wei z What is meant by the word “Meta-analysis” Harvard University ¾ Meta is Greek for “later in time” ¾ Meta is now used to denote something that goes to a higher level or is more comprehensive. ¾ How is an analysis made more comprehensive? z In empirical research, there are often multiple studies addressing the same research question ¾ A standard analysis attempts to reach a conclusion based on a single study without reference to any other studies. ¾ A meta-analysis attempts to reach a conclusion based on a set of studies that address the same hypothesis. Introduction Introduction History of Meta Analysis Why Meta Analysis z In 1805, Legendre developed least squares to combine data on the orbits z There are several reasons for conducting a meta-analysis of of comets from different observatories. the results of previous studies: z In 1930’s, statisticians working in agricultural research developed methods ¾ The increasingly large # of research studies for combining the results of studies. Most notable are Fisher and ¾ 40,000 journals for the sciences 5000 Cochrane. ¾ 1 article every 30 seconds 4000 z In 1960’s, Cohen popularized the notion of effect size for use in sample 3000 size determination in the social and behavioral sciences 2000 1000 ¾ Effect size measures the differences between null hypothesis and the truth 0 ¾ Effect size + sample size determines the power. 1986 1991 1996 2001 z In 1976, Grass published an article “Primary, secondary and meta-analysis ¾ Unsystematic expert reviews of an area of research are often biased or of research”. This is when the term “meta-analysis” was first used. years behind the current research. ¾ Systematic and quantitative reviews are needed to summarize findings in a timely manner without bias. Formulating Hypothesis and Principles of Meta-analysis Effect Measures z Meta-analysis is typically a two stage process z Before conducting a meta analysis, it is important to decide 1) Summary statistic calculated from each study. the hypothesis or aim of the analysis. When formulating a ¾ For controlled trials, these values describe the treatment effects hypothesis for meta-analysis, it is important to determine observed in each trial. 2) Pooled effect is calculated by combining treatment effect ¾ the precise question the meta-analysis aims to address estimates from individual studies. ¾ whether the meta analysis is exploratory or hypothesis testing ¾ Typically a weighted average of individual effects ¾ Hypothesis testing: is the intent of the study to provide a definitive test (usually a test of average effect = 0) z The combination of treatment effect estimates across ¾ Exploratory: are there variations in the treatment or characteristics of the studies may assume studies that lead to better outcomes? Fixed effects: treatment effects the same across studies. ¾ One also needs to select an appropriate effect measure Random effects: treatment effects ~ a distribution across studies. Type of Data and Effect Measures Type of Data and Effect Measures z Dichotomous or binary outcome z Ordinal outcome ¾ Takes values y < y < … < y ¾ when events of interest are rare 1 2 K ¾ Example: “mild”, “moderate” and “severe”. ¾ 1 – p1 # 1 and 1 – p0 # 1 Î RR # OR ¾ When the number of categories is large, such data are often ¾ essential to assess the absolute risk in addition to relative risk analyzed as continuous data. ¾ RR and OR = f if there are no events in the control group ¾ One may transform ordinal data into binary data by combining adjacent categories. ¾ RR and OR not defined if both groups have zero events ¾ Proportional odds ratio under a proportional odds model ¾ Standard procedures either exclude studies with 0/0 events or add 0.5 to empty cells. logit P(Y d yk | Trt) D k ȕTrt ¾ E, the log-odds ratio, summarizes the treatment effect Statistical Methods for Combining Fixed Effects Results Across Studies Vote Count z What is the true effect? Depends on the underlying ¾ Consider # of studies in favor of the conclusion (say, assumption about the study specific effect reach the 0.05 level of significance ) and examine if they are the majority ¾ Fixed Effects Assumption ¾ Assumes that all studies have the same true effect ¾ This approach has been used a lot due to its simplicity, ¾ Variability only within each study but has several drawbacks ¾ Precision depends mainly on study size ¾ Significance depends on study sample size and effect size. ¾ Random Effects Assumption ¾ Even if the null hypothesis is wrong and studies are not small, the percentage of trials reaching significance could still be less than 50% ¾ Studies allowed to have different underlying or true effects Î low power of detecting a treatment effect ¾ Allows variation between studies as well as within studies ¾ Vote counts do not provide an estimate of effect size. z Basic assumption: study results are independent. Fixed Effects Fixed Effects Pooling Using Effect Sizes Pooling Using Effect Sizes ¾ Binary Outcomes ¾ Since Glass’s work in 1976, combining effect sizes has ¾ When the event rates are low or trial sizes are small, the standard become the main form of meta-analysis. error estimates used in the inverse variance method may be poor. ¾ Cochrane-Mantel-Hansel uses a different weighting scheme that ¾ Suppose the estimated effect sizes are depends upon the effect measure (eg RR, OR, RD). { ˆ , i 1,..., S} Ei ¾ Cochrane-Mantel-Hansel pooled OR: ¾ To ascertain the true underlying effect, a common S S MH MH MH MH m0i (n1i m1i ) OR wˆ OR wˆ wˆ ¦ i i ¦ i i approach is to consider a weighted average of the effect i 1 i 1 n1i n0i estimates from individual studies: S S ith Study Event No Event Total ˆ ˆ m (n m ) E wi Ei wi OR 1i 0i 0i ¦ ¦ Intervention m1i n1i -m1i n1i i i 1 i 1 m0i (n1i m1i ) Control m0i n0i -m0i n0i Between Study Heterogeneity Between Study Heterogeneity z Testing for heterogeneity z The key assumption of fixed effects meta analysis S Q wˆ (ȕˆ ȕˆ )2 ~ F 2 under H methods is that all primary studies are estimating ¾ Cochran’s Q-test: ¦ i pooled i S 1 0 i 1 the same underlying true effect ¾ Provides a measure of between study variation. z The underlying effects across studies may be ¾ Other descriptive measures of heterogeneity ¾ H statistic:H Q /(S 1) has mean 1 under H . heterogeneous 0 ˆ ¾ Hoggins and Thompson (2002) suggested: H > 1.5 Îcaution regarding z Each study effect sizeEi is estimating an individual heterogeneity; H < 1.2 Î little heterogeneity population effect Ei. ¾ I2 statistic = (H2 –1)/H2 z ˆ ¾ % of total variability in effect size due to between study variation As study sample size Ni Æ f, Ei o Ei ¾ I2 ~ 0 Î little heterogeneity; I2 ~ 1 Î high heterogeneity z Some of the Ei may be the same, but not all of them. ¾ termed the “inconsistency” of the trials included in meta-analysis and has become a preferred measure of heterogeneity. Meta Regression Meta Regression Special Cases z The pooled effect size estimates the average effect across all z The DerSimonian and Laird (1986) Model: studies Yˆ ˆ z In the presence of heterogeneity i Į ȕi ] i ¾ the validity of such an average measure? ¾ not a single population effect size that applies to all studies z The Begg and Pilote (1991) Model: ¾ random effects pooling addresses heterogeneity to some extent ˆ ˆ z Meta regression Yi XiĮ ȕi ] i ¾ provide an alternative approach that allows exploration of why studies have varied effect sizes. ¾ This model allows the inclusion of single treatment historical controls as well as comparative trials in a treatment effect assessment. ¾ one uses characteristics of the studies to explain the excess variation in effect sizes ¾ Thompson and Higgins (2002) reviewed several meta-regression methods Example Effect of Rosiglitazone on MI or CVD Deaths z Nissen and Wolski (2007) performed a meta analysis to examine whether Rosiglitazone (Avandia, GSK), a drug for treating type 2 diabetes mellitus, significantly increases the risk of MI or CVD related death. Exact Procedure for z Avandia was introduced in 1999 and is widely used as monotherapy or in fixed-dose combinations with either Avandamet or Avandaryl. Combining 2x2 Tables for The original approval of Avandia was based on the ability of the Rare Events drug to reduce blood glucose and glycated hemoglobin levels. z Initial studies were not adequately powered to determine the effects of this agent on microvascular or macrovascular complications of diabetes, including cardiovascular morbidity and mortality. Exact Meta-Analysis Procedure Combining Exact Intervals z Questions: z Could we combine information across studies without z excluding studies with 0 events or z artificial imputation? z Could we make exact inference without relying on possibly inaccurate large sample approximations when z the total number of studies is small, or z the sample sizes of individual studies are small, or z when the event rates are low. • Event Rates from 0% to 2.70% for MI • Event Rates from 0% to 1.75% for CVD Death Exact Meta-Analysis Procedure Exact Meta-Analysis Procedure Combining Exact Intervals Combining Exact Intervals z Thus, we propose to include ' in the 100(1 – D)% level z For a given confidence levelK, Iˆ (K) Study 1 1 confidence interval (a, f) if z one may obtain S study specific one-sided S K-level confidence intervals for the risk Iˆ ( ) Study i i K t(',K) w {y (',K) K} t c difference.
Details
-
File Typepdf
-
Upload Time-
-
Content LanguagesEnglish
-
Upload UserAnonymous/Not logged-in
-
File Pages63 Page
-
File Size-