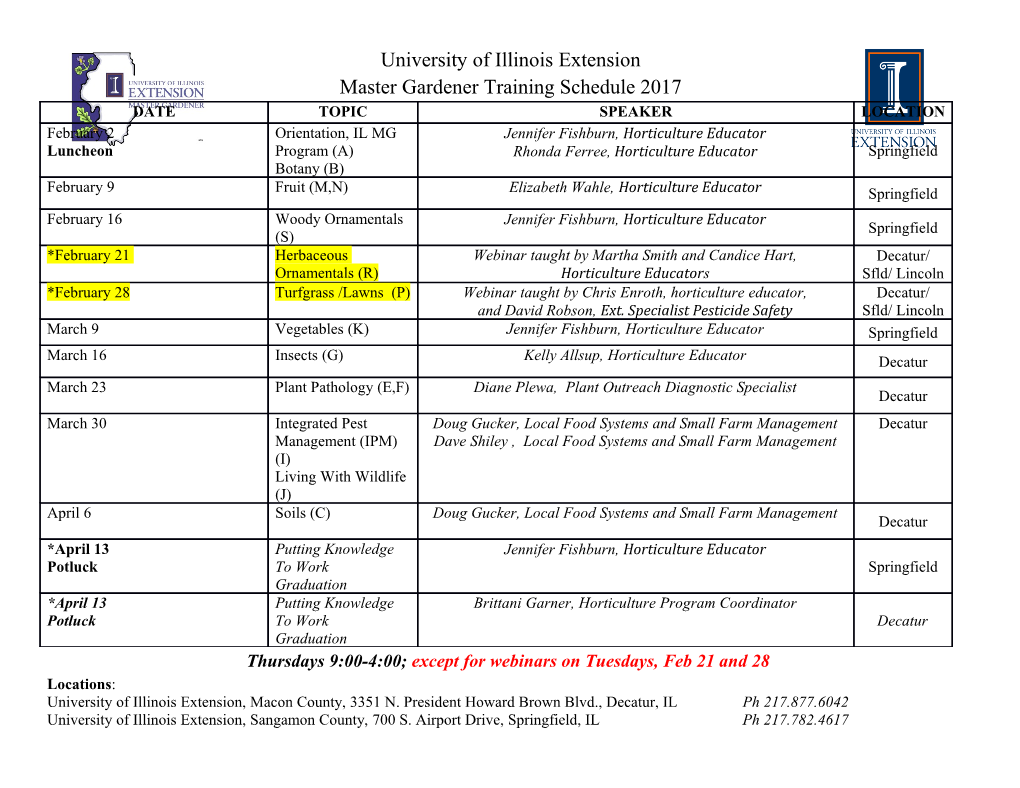
QUARTERLY FORECASTING MODEL FOR INDIA’S ECONOMIC GROWTH: BAYESIAN VECTOR AUTOREGRESSION APPROACH Tara Iyer and Abhijit Sen Gupta NO. 573 ADB ECONOMICS March 2019 WORKING PAPER SERIES ASIAN DEVELOPMENT BANK ADB Economics Working Paper Series Quarterly Forecasting Model for India's Economic Growth: Bayesian Vector Autoregression Approach Tara Iyer and Abhijit Sen Gupta Tara Iyer ([email protected]) is a consultant and Abhijit Sen Gupta ([email protected]) is senior economics officer of the India Resident Mission of the No. 573 | March 2019 Asian Development Bank. The authors would like to gratefully acknowledge the valuable guidance and detailed comments received from Lei Lei Song at various stages. The authors thank Sabyasachi Mitra for very helpful feedback. The authors also appreciate the comments of an anonymous reviewer. All errors remain the authors’ responsibility. ASIAN DEVELOPMENT BANK Creative Commons Attribution 3.0 IGO license (CC BY 3.0 IGO) © 2019 Asian Development Bank 6 ADB Avenue, Mandaluyong City, 1550 Metro Manila, Philippines Tel +63 2 632 4444; Fax +63 2 636 2444 www.adb.org Some rights reserved. Published in 2019. ISSN 2313-6537 (print), 2313-6545 (electronic) Publication Stock No. WPS190056-2 DOI: http://dx.doi.org/10.22617/WPS190056-2 The views expressed in this publication are those of the authors and do not necessarily reflect the views and policies of the Asian Development Bank (ADB) or its Board of Governors or the governments they represent. ADB does not guarantee the accuracy of the data included in this publication and accepts no responsibility for any consequence of their use. The mention of specific companies or products of manufacturers does not imply that they are endorsed or recommended by ADB in preference to others of a similar nature that are not mentioned. By making any designation of or reference to a particular territory or geographic area, or by using the term “country” in this document, ADB does not intend to make any judgments as to the legal or other status of any territory or area. This work is available under the Creative Commons Attribution 3.0 IGO license (CC BY 3.0 IGO) https://creativecommons.org/licenses/by/3.0/igo/. By using the content of this publication, you agree to be bound by the terms of this license. For attribution, translations, adaptations, and permissions, please read the provisions and terms of use at https://www.adb.org/terms-use#openaccess. This CC license does not apply to non-ADB copyright materials in this publication. If the material is attributed to another source, please contact the copyright owner or publisher of that source for permission to reproduce it. ADB cannot be held liable for any claims that arise as a result of your use of the material. Please contact [email protected] if you have questions or comments with respect to content, or if you wish to obtain copyright permission for your intended use that does not fall within these terms, or for permission to use the ADB logo. Corrigenda to ADB publications may be found at http://www.adb.org/publications/corrigenda. Notes: In this publication, “$” refers to United States dollars. ADB recognizes “Russia” as the Russian Federation. The ADB Economics Working Paper Series presents data, information, and/or findings from ongoing research and studies to encourage exchange of ideas and to elicit comment and feedback about development issues in Asia and the Pacific. Since papers in this series are intended for quick and easy dissemination, the content may or may not be fully edited and may later be modified for final publication. CONTENTS TABLES AND FIGURES iv ABSTRACT v%% I. INTRODUCTION 1 II. MACROECONOMIC TRENDS IN INDIA 4 III. FRAMEWORK 11 A. Real and Monetary Variables 12 B. Structural Vector Autoregressions 14 C. Forecast Evaluation 15 IV. DATA 15 V. EMPIRICAL RESULTS 17 A. Univariate Autoregressive Integrated Moving Average Models 17 B. Bayesian Vector Autoregressions: Q1 2004–Q1 2017 Estimation Period 18 C. Bayesian Vector Autoregressions: Q1 2011–Q1 2017 Estimation Period 20 D. Vector Autoregression and Structural Vector Autoregression Forecasts 23 E. Extending the Validation Period 24 F. Inflation Forecasts 24 G. The Changing Dynamics of Gross Domestic Product Growth 25 VI. CONCLUSION 26 APPENDIX 28 REFERENCES 37 TABLES AND FIGURES TABLES 1 Real Sector Groups in the Bayesian Vector Autoregressions 13 2 Monetary Sector Groups in the Bayesian Vector Autoregressions 13 3 Structural Vector Autoregression Models 14 4 Empirical Models 16 5 Top 10 Bayesian Vector Autoregression Forecasting Models of Gross Domestic Product 18 Growth (Q1 2004–Q1 2017 estimation period) 6 Composition of the Top 50 Gross Domestic Product Growth Forecasts 20 (Q1 2004–Q1 2017 estimation period) 7 Composition of the Top 50 Gross Domestic Product Growth Forecasts 21 (Q1 2011–Q1 2017 estimation period) 8 Top 10 Bayesian Vector Autoregression Forecasting Models of Gross Domestic Product 22 Growth (Q1 2011–Q1 2017 estimation period) 9 Dynamic Bayesian Vector Autoregression and Autoregressive Integrated Moving Average 25 Forecasts of Consumer Price Index Inflation (Q1 2004–Q1 2017 estimation period) 10 Composition of the Top 50 Gross Domestic Product Growth Forecasts 26 (Q1 2000–Q4 2006 estimation period) A.1 Data Sources 28 A.2 Top 50 Bayesian Vector Autoregression Forecasting Models of Gross Domestic Product 30 Growth (Q1 2004–Q1 2017 estimation period) A.3 Top 50 Bayesian Vector Autoregression Forecasting Models of Gross Domestic Product 31 Growth (Q1 2011–Q1 2017 estimation period) A.4 Top 10 Vector Autoregression Forecasting Models of Gross Domestic Product Growth 33 (Q1 2004–Q1 2017 estimation period) A.5 Top 10 Vector Autoregression Forecasting Models of Gross Domestic Product Growth 33 (Q1 2011–Q1 2017 estimation period) A.6 Structural Vector Autoregression Forecasting Models of Gross Domestic Product Growth 34 (Q1 2004–Q1 2017 estimation period) A.7 Structural Vector Autoregression Forecasting Models of Gross Domestic Product Growth 34 (Q1 2011–Q1 2017 estimation period) A.8 Dynamic Bayesian Vector Autoregression and Autoregressive Integrated Moving Average 35 Forecasts of Consumer Price Index Inflation (Q1 2011–Q1 2017 estimation period) A.9 Top 50 Bayesian Vector Autoregression Forecasting Models of Gross Domestic Product 35 Growth (Q1 2000–Q4 2006 estimation period) FIGURES 1 Gross Domestic Product Growth 5 2 Decomposition of Gross Domestic Product Growth 5 3 Growth Rate of Key Components of Gross Domestic Product 6 4 Trade Integration 7 5 Export and Import Growth 7 6 Inflation 8 7 Repo and Reverse Repo Rates 9 8 Oil Inflation and Gross Domestic Product Growth 9 9 International Financial Integration 10 10 Portfolio Flows and Foreign Direct Investment 10 11 Effective Exchange Rates 10 12a Dynamic Forecasts of Gross Domestic Product Growth (Q2 2017–Q1 2018) 19 12b Dynamic Forecasts of Gross Domestic Product Growth (Q2 2017–Q1 2018): A Closer Look 19 13a Dynamic Forecasts of Gross Domestic Product Growth (Q2 2017–Q1 2018) 22 13b Dynamic Forecasts of Gross Domestic Product Growth (Q2 2017–Q1 2018): A Closer Look 23 14 5-Quarter Ahead Bayesian Vector Autoregression Forecast 24 15 6-Quarter Ahead Bayesian Vector Autoregression Forecast 24 ABSTRACT This study develops a framework to forecast India’s gross domestic product growth on a quarterly frequency from 2004 to 2018. The models, which are based on real and monetary sector descriptions of the Indian economy, are estimated using Bayesian vector autoregression (BVAR) techniques. The real sector groups of variables include domestic aggregate demand indicators and foreign variables, while the monetary sector groups specify the underlying inflationary process in terms of the consumer price index (CPI) versus the wholesale price index given India’s recent monetary policy regime switch to CPI inflation targeting. The predictive ability of over 3,000 BVAR models is assessed through a set of forecast evaluation statistics and compared with the forecasting accuracy of alternate econometric models including unrestricted and structural VARs. Key findings include that capital flows to India and CPI inflation have high informational content for India’s GDP growth. The results of this study provide suggestive evidence that quarterly BVAR models of Indian growth have high predictive ability. Keywords: Bayesian vector autoregressions, GDP growth, India, time series forecasting JEL codes: C11, C32, C53, F43 I. INTRODUCTION This study seeks to develop an appropriate forecasting model to predict India’s gross domestic product (GDP) growth. It is well known that econometric forecasting has historically been an exercise fraught with uncertainty. The challenge arises in designing a framework that accurately captures the underlying economic structure and the precise variables that provide informational content on the indicators of interest. The forecasting framework should be specific to the country in question, while noting that more complex models with numerous macroeconomic interlinkages often do not perform as well as parsimonious models. Additional econometric challenges arise in developing and emerging market economies (EMEs) since the time series can be more volatile, and sometimes certain variables are unavailable. Yet, precise forecasts are of utmost importance to various stakeholders including policy makers and monetary authorities for whom successful policy decisions depend on accurate predictions. This paper develops an econometric framework that is effective in predicting GDP growth
Details
-
File Typepdf
-
Upload Time-
-
Content LanguagesEnglish
-
Upload UserAnonymous/Not logged-in
-
File Pages47 Page
-
File Size-