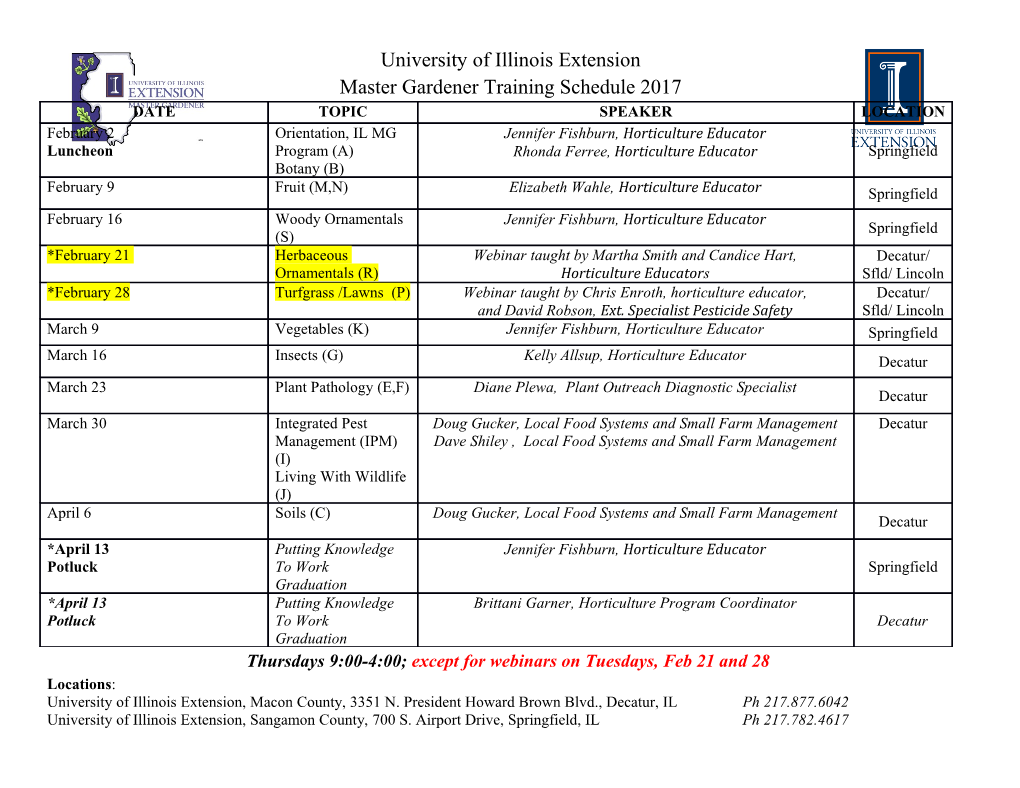
Introduction to Matlab MATLAB Tutorial Amir massoud Farahmand http://www.cs.ualberta.ca/~amir Ela Pek! alska, Marjolein van der Glas [CMPUTPattern Recognit651] PrionobabilisticGroup, Faculty Graof Appliephicald Scienc Modelses Russ DelftGreinerUniversit andy of T Mattechnology Brown VersionJan 0.6:uary September2002 24, 2008 Send comments to [email protected] The MATLAB logo is a trademark of MathWorks, Inc. What is MATLAB? • A scripting/programming language for numerical calculations • Pros • Fast prototyping for your [numerical] algorithms • Drawing graphs easily • Fast matrix computation • Cons • Slow for many other things (e.g. for loops are terribly slow) • Not a general-purpose programming language • Not so cheap How to Run MATLAB @ U of A? login to bonanza, pipestone, eureka, etc. or matlab -nodesktop You need to type here! I Will Talk About ... • vectors and matrices in MATLAB • several useful predefined functions • graphics • writing your own functions • tricks for writing efficient codes • ... Vectors >> a = 2 a = 2 >> b = [1 2 3] b = 1 2 3 >> c = [-1 1.1 2]' c = -1.0000 1.1000 2.0000 Vectors >> a = 2 >> a*b a = ans = 2 2 4 6 >> b = [1 2 3] >> c*b b = ans = 1 2 3 -1.0000 -2.0000 -3.0000 1.1000 2.2000 3.3000 >> c = [-1 1.1 2]' 2.0000 4.0000 6.0000 c = >> b*c -1.0000 ans = 1.1000 2.0000 7.2000 Vectors >> sin(b) >> a = 2 >> a*b ans = a = ans = 0.8415 0.9093 0.1411 2 2 4 6 >> exp(c) >> b = [1 2 3] >> c*b ans = b = ans = 0.3679 3.0042 1 2 3 -1.0000 -2.0000 -3.0000 7.3891 1.1000 2.2000 3.3000 >> c = [-1 1.1 2]' 2.0000 4.0000 6.0000 >> b + c ??? Error using ==> plus c = >> b*c Matrix dimensions must agree. -1.0000 ans = >> b + c' 1.1000 2.0000 7.2000 ans = 0 3.1000 5.0000 Another way to generate vectors >> x = -2*pi:0.01:2*pi; >> y = sin(x); >> y2 = sin(x) + cos(2*x) + 0.1*sin(10*x); >> plot(x,y,'b') >> hold Current plot held >> plot(x,y2,'k') >> x = -2*pi:0.01:2*pi; >> y = sin(x); >> y2 = sin(x) + cos(2*x) + 0.1*sin(10*x); >> plot(x,y,'b') >> hold Current plot held >> plot(x,y2,'k') xlabel('Time'); ylabel('Amplitude'); title('My sinusoid wave') >> A = [1 2 3;4 5 6] Matrices A = 1 2 3 4 5 6 >> B = ones(3,5) B = 1 1 1 1 1 1 1 1 1 1 1 1 1 1 1 >> C = zeros(3) C = 0 0 0 0 0 0 0 0 0 >> A = [1 2 3;4 5 6] Matrices A = 1 2 3 4 5 6 >> B = ones(3,5) size(D) >> D = A*B B = ans = D = 1 1 1 1 1 1 1 1 1 1 2 5 6 6 6 6 6 1 1 1 1 1 15 15 15 15 15 >> C = zeros(3) C = 0 0 0 0 0 0 0 0 0 Accessing Elements of Vectors and Matrices a = [1 2 3 4 5 6 7 8 9 10] A = [1 2 3 4;5 6 7 8; 9 10 11 12;13 14 15 16] a = A = 1 2 3 4 5 6 7 8 9 10 1 2 3 4 5 6 7 8 a(3) 9 10 11 12 13 14 15 16 ans = >> B = A(2:3,2:4) 3 B = 6 7 8 10 11 12 Random Number Generators rand(5,1) Uniform ans = 0.0193 0.7683 0.4579 0.0436 0.0996 >> randn(5,1) Normal (Gaussian) ans = -0.6223 -0.8081 -0.8724 0.1395 0.6093 Random Numbers and Histograms X1 = randn(2000,1); >> X2 = rand(2000,1); >> hist(X1,20) sums, means, var X = randn(1000,1); >> Y = rand(1000,1); >> sum(X), mean(X), var(X) min(X), max(X) ans = -14.6247 ans = ans = -3.5851 -0.0146 ans = ans = 3.7476 1.0325 >> min(Y), max(Y) >> sum(Y), mean(Y), var(Y) ans = ans = 0.0010 491.2878 ans = ans = 0.9973 0.4913 ans = 0.0863 Matrices Again! A = [1 2 3; 4 5 6; 7 8 9] A*B A = ans = 1 2 3 3 2 4 4 5 6 9 2 10 7 8 9 15 2 16 >> B = [1 -1 1;1 0 0;0 1 1] B = >> A.*B ans = 1 -1 1 1 0 0 1 -2 3 0 1 1 4 0 0 >> rank(A) 0 8 9 ans = B./A 2 ans = >> eig(A) ans = 1.0000 -0.5000 0.3333 0.2500 0 0 16.1168 0 0.1250 0.1111 -1.1168 -0.0000 How to Write a Program? • Script • Anything that you do in command line except in a single file • Functions • Get inputs, return outputs MATLAB for Machine Learning: Regression Assume that y = f(x) + ! where E ! = 0. The goal is estimating the { } regressor, f(.), using samples (Xi, Yi) , i = 1, , n. There are books written on thi{ s topic,}but for·n· o· w, we consider a simple (but efficient) method called Kernel regression estimator. Let h > 0 be a positive number called the bandwidth. The Nadaraya-Watson kernel estimator is defined by n 1 x xi fˆ(x) = K − Yi n x xj h K − i=1 j=1 h $ % & ! " # Kernels can have different forms. An example of them is Gaussian kernel: 1 K(x) = exp( x2/2). √2π − See L. Wasserman, All of Nonparametric Statistics (Section 5.4) for more information. Let’s implement it! What Do We Need? • Some function f(x) • Noisy samples from f(x) • Kernel regressor • kernel function (e.g. Gaussian) A Script File as the Skeleton of the Program edit KernelRegressionTest First Attempt KernelRegressionTest.m KernelRegressor.m % Number of samples function yhat = KernelRegressor(xQuery,XTrain,YTrain,h) n = 1000; % Noise variance sigma = 1; SizeOfTrainingSet = size(XTrain,2); % Bandwidth yhat = 0; h = 0.05; Normalizer = 0; for m=1:SizeOfTrainingSet Let h > 0 be a positive number called the bandwid Kxi = exp( -(xQueryth. -The XTrain(:,m))^2/hNad );araya-Watson xSamples = (rand(1,n)-0.5)*8; yhat = yhat + Kxi*YTrain(m); xGridkernel = linspace(-4,4,2000)estimator; is defined by Normalizer = Normalizer + Kxi; end ySamples = 2*sin(2*xSamples) + 0.5*cos(5*xSamples) + sigma^2 * randn(1,n); yTrue = 2*sin(2*xGrid) + 0.5*cos(5*xGrid); n yhat = yhat/Normalizer; 1 x x plot(xSamples,ySamples,'.') ˆ i hold on; f(x) = K − Yi n x x plot(xGrid,yTrue,'r','Linewidth',3); − j h j=1 K h i=1 % & $ % Evaluating the regressor on a set of test points " # ! for m=1:length(xGrid) yTest(m) = KernelRegressor(xGrid(:,m),xSamples,ySamples,h); end plot(xGrid,yTest,'g','Linewidth',2); First Attempt tic; KernelRegressionTest; toc @ Command line Elapsed time is 31.262343 seconds. profile on KernelRegressionTest profile report Source of slowness: for loop. Vectorization Avoid loops; vectorize! FOR loop solution: tic; i = 1; for x=0:0.001:8*pi; y(i) = sin(x); i = i+1; end; toc Elapsed time is 9.641997 seconds. Sin #2: Incremental growing of vectors Sin #1: for loop Vectorized solution: >> tic; x = 0:0.001:8*pi; y = sin(x); toc A = [1 2 3;4 5 6] Elapsed time is 0.013867 seconds. A = 1 2 3 4 5 6 >> repmat(A,3,2) Related trick: ans = 1 2 3 1 2 3 4 5 6 4 5 6 1 2 3 1 2 3 4 5 6 4 5 6 1 2 3 1 2 3 4 5 6 4 5 6 Vectorization What can be done for the previous code? for m=1:SizeOfTrainingSet Kxi = exp( -((xQuery - XTrain(:,m))^2)/h ); yhat = yhat + Kxi*YTrain(m); Normalizer = Normalizer + Kxi; end scalar XTrain is originally a vector, but we de-vecotorize it to a scalar here. Let’s vectorize xQuery. xQueryRepeated = repmat(xQuery,1,SizeOfTrainingSet); and do all calculations at once. KX = exp( -((xQueryRepeated - XTrain).^2) / h); yhat = sum(KX.*YTrain)/sum(KX); Second Attempt function yhat = KernelRegressor(xQuery,XTrain,YTrain,h) tic; KernelRegressionTest; toc Elapsed time is 0.514618 seconds. SizeOfTrainingSet = size(XTrain,2); % First solution (slow) if 1>2 yhat = 0; Normalizer = 0; for m=1:SizeOfTrainingSet Kxi = exp( -((xQuery - XTrain(:,m))^2)/h ); yhat = yhat + Kxi*YTrain(m); Normalizer = Normalizer + Kxi; end % Second solution (faster) else xQueryRepeated = repmat(xQuery,1,SizeOfTrainingSet); KX = exp( -((xQueryRepeated - XTrain).^2) / h); yhat = sum(KX.*YTrain)/sum(KX); end Remarks on Kernel Regressor • Easily extendable to multi-dimensions • Selecting the bandwidth is important • Model selection • Theoretical results Other Useful Functions, Commands, ... • inv(A), pinv(A), det(A), cond(A), svd(A) • & (logical AND), | (logical OR), ~ (logical NOT) • clear, whos, load, save • help • surf, mesh, plot3, comet, ... • Lots of other functions (ODE, optimization, control toolbox, etc.) Resources • MATLAB’s help (command line’s help or the manual) • E. Pekalska and M. van der Glas, Introduction to MATLAB, 2002. (http://www.cs.ualberta.ca/ ~dale/cmput466/w06/matlab_manual.pdf) • MATLAB 7.6 demos (http:// www.mathworks.com/products/matlab/ demos.html) • Many others.
Details
-
File Typepdf
-
Upload Time-
-
Content LanguagesEnglish
-
Upload UserAnonymous/Not logged-in
-
File Pages31 Page
-
File Size-