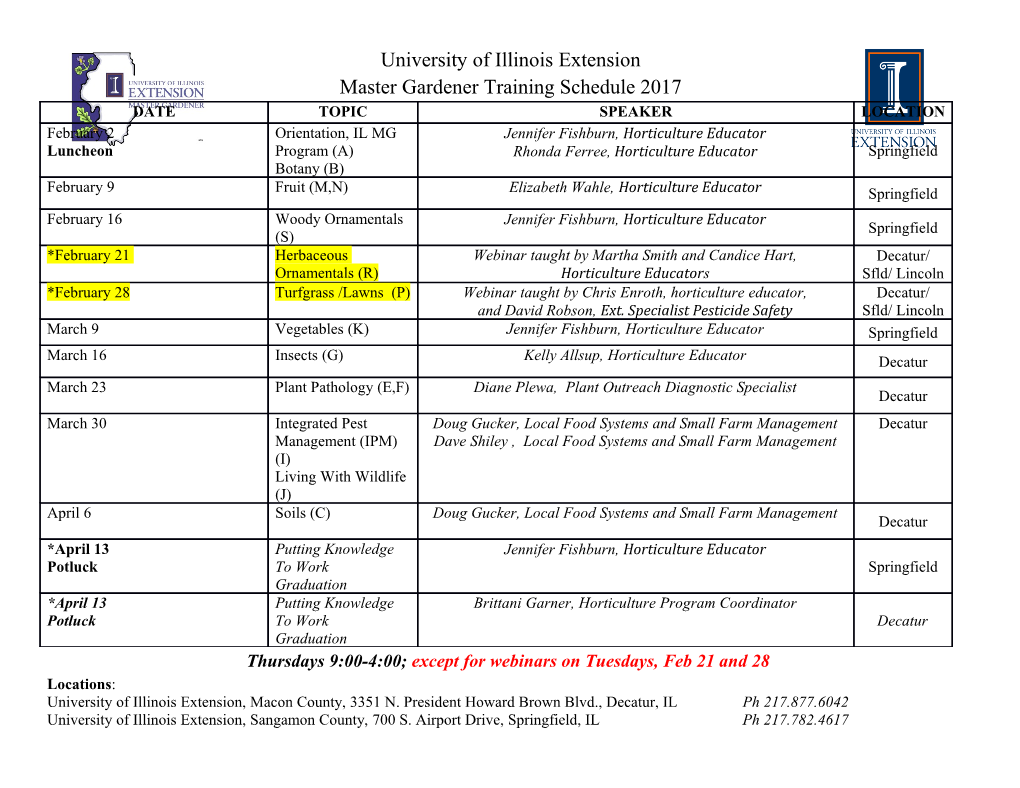
The dimensionality of discourse Isidoros Doxasa,1, Simon Dennisb,2, and William L. Oliverc aCenter for Integrated Plasma Studies, University of Colorado, Boulder, CO 80309; bDepartment of Psychology, Ohio State University, Columbus, OH 43210; and cInstitute of Cognitive Science, University of Colorado, Boulder, CO 80309 Edited* by Richard M. Shiffrin, Indiana University, Bloomington, IN, and approved February 2, 2010 (received for review July 26, 2009) The paragraph spaces of five text corpora, of different genres and accurately estimate passage coherence. In addition, LSA has found intended audiences, in four different languages, all show the same application in many areas, including selecting educational materials two-scale structure, with the dimension at short distances being for individual students, guiding on-line discussion groups, providing lower than at long distances. In all five cases the short-distance feedback to pilots on landing technique, diagnosing mental disor- dimension is approximately eight. Control simulations with ran- ders from prose, matching jobs with candidates, and facilitating domly permuted word instances do not exhibit a low dimensional automated tutors (3). structure. The observed topology places important constraints on By far the most surprising application of LSA is its ability to grade the way in which authors construct prose, which may be universal. student essay scripts. Foltz et al. (9) summarize the remarkable reliability with which it is able to do this, especially when compared correlation dimension | language | latent semantic analysis against the benchmark of expert human graders. In a set of 188 essays written on the functioning of the human heart, the average s we transition from paragraph to paragraph in written dis- correlation between two graders was 0.83, whereas the correlation course, one can think of the path through which one passes as a A of LSA’s scores with the graders was 0.80. A summary of the per- trajectory through a semantic space. Understanding the discourse is, ’ in some sense, a matter of understanding this trajectory. Although it formance of LSA s scoring compared with the grader-to-grader is difficult to predict exactly what will follow from a given discourse performance across a diverse set of 1,205 essays on 12 topics showed context, we can ask a broader question. Does the cognitive system an interrater reliability of 0.7 and a rater-to-LSA reliability of 0.7. impose any fundamental constraints on the way in which the dis- LSA has also been used to grade two questions from the stand- course evolves? ardized Graduate Management Admission Test. The performance To investigate this issue, we constructed semantic spaces for five was compared against two trained graders from Educational Testing corpora, of different genres and intended audiences, in four dif- Services (ETS). For one question, a set of 695 opinion essays, the ferent languages and then calculated the intrinsic dimensionality of correlation between the two graders was 0.86, and LSA’scorrelation the paragraph trajectories through these corpora. Each trajectory with the ETS grades was also 0.86. For the second question, a set of can be embedded in an arbitrary number of dimensions, but it has 668 analyses of argument essays, the correlation between the two an intrinsic dimensionality, independent of the embedding space. graders was 0.87, whereas LSA’s correlation to the ETS grades For instance, in latent semantic analysis (LSA) applications, it is was 0.86. typical to use a 300-dimensional space (1). However, the points that In the research outlined above, LSA was conducted on para- represent the paragraphs in this 300-dimensional space do not fill graphs. However, it is known to degrade rapidly if applied at the the embedding space; rather they lie on a subspace with a dimen- sentence level, where capturing semantics requires one to estab- sion lower than the embedding dimension. The fact that a low- fi dimensional structure can be embedded in a higher dimensional lish the llers of thematic roles and extract other logical rela- space is routinely used in the study of nonlinear dynamic systems, in tionships between constituents. Nevertheless, to achieve the which the embedding theorem (2) relates the dimensionality of the results outlined above, LSA must be capturing an important dataset under study to the dimensionality of the dynamics that component of what we would typically think of as the semantics or describes it. meaning of texts. In this study, we investigate the geometric Historically, the dimensionality of the discourse trajectory has structure of this kind of semantics. been implicitly assumed to be very large, but it has never been calculated. Here we show that the dimensionality of the trajectory The Correlation Dimension is surprisingly low (approximately eight at short distances) and There are many different dimensions that can be defined for a that its structure is probably universal across human languages. given dataset. They include the Hausdorff dimension, the family Although the question of dimensionality has not generally been of fractal dimensions, Dn (capacity, D0; information, D1; corre- considered before, it can be used to guide the development of new lation, D2, etc), the Kaplan-Yoke dimension, etc. (10). A usual models of prose, which are constrained to reproduce the observed choice for small datasets is the correlation dimension (11) dimensional structure. because it is more efficient and less noisy when only a small ≥ Modeling Semantics number of points is available. It can be shown that Dcapacity D ≥ D , but in practice almost all attractors The first step toward being able to calculate the dimensionality of information correlation text is to create a vector representation of the semantics conveyed have values of the various dimensions that are very close to each by each paragraph. Recent years have seen increasing interest in other (10, 12). automated methods for the construction of semantic representa- The correlation dimension is derived by considering the cor- tions of paragraphs [e.g., LSA (3), the topics model (4, 5), non- relation function negative matrix factorization (6), and the constructed semantics model (7)]. These methods were originally developed for use in information retrieval applications (8) but are now widely applied in Author contributions: I.D., S.D., and W.L.O. designed research; I.D., S.D., and W.L.O. performed both pure and applied settings (3). For example, LSA measures research; I.D., S.D., and W.L.O. analyzed data; and I.D., S.D., and W.L.O. wrote the paper. correlate with human judgments of paragraph similarity; correlate The authors declare no conflict of interest. highly with humans’ scores on standard vocabulary and subject *This Direct Submission article had a prearranged editor. matter tests; mimic human word sorting and category judgements; 1Present address: BAE Systems, Washington, DC 20037. simulate word–word and passage–word lexical priming data; and 2To whom correspondence should be addressed. E-mail: [email protected]. 4866–4871 | PNAS | March 16, 2010 | vol. 107 | no. 11 www.pnas.org/cgi/doi/10.1073/pnas.0908315107 Downloaded by guest on September 30, 2021 shows a plot of the correlation function for such a structure. We see 2 N N ! ! ∑ ∑ − − ; [1] that the three scales are clearly distinguishable, with narrow tran- CðlÞ¼ − Hðl j X i X j jÞ NðN 1Þ i¼1 j¼1ðj≠iÞ sition regions around the length scales of the wall thickness and ! diameter, as expected. A similar example in the reverse order is a where X i is an M dimensional vector pointing to the location of large piece of woven cloth. It looks two-dimensional at long scales, the ith point in the dataset in the embedding space, M is the but at short scales it is composed of one-dimensional threads. This number of dimensions within which the data are embedded, N is is the picture that the five language corpora that we have studied the total number of data points, and H is the Heaviside function. present; they look low-dimensional at short scales and higher- The correlation function is therefore the normalized count of the dimensional at long scales. number of distances between points in the dataset that are less than the length l. The correlation dimension, ν, is then given by Description of the Corpora À Á fi ν We have calculated the correlation dimension of ve corpora, in lim C l ∝ l : [2] l→0; N→∞ English, French, modern and Homeric Greek, and German. The English corpus includes text written for children as well as adults, In other words, the correlation dimension is given by the slope of representing the range of texts that a typical US college freshman the ln[C(l)] vs. ln(l) graph. will have encountered. The French corpus includes excerpts from The correlation dimension captures the way that the number of articles in the newspaper Le Monde, as well as excerpts from points within a distance l scales with that distance. For points on a novels written for adults. The modern Greek corpus comprises line, doubling the distance l would double the number of points articles in the political, cultural, economic, and sports pages of the that can be found within that distance. For points on a plane, the newspaper Eleftherotypia, as well as articles from the political number of points within a distance l quadruples as l doubles. In pages of the newspaper Ta Nea. The German corpus includes ν general, the number of points within distance l will scale as l , articles from German textbooks and text extracted from Internet where ν is the correlation dimension (Fig. 1A). sites and is intended to represent the general knowledge of an The correlation dimension, as well as all other dimensions, are adult native speaker of German.
Details
-
File Typepdf
-
Upload Time-
-
Content LanguagesEnglish
-
Upload UserAnonymous/Not logged-in
-
File Pages6 Page
-
File Size-