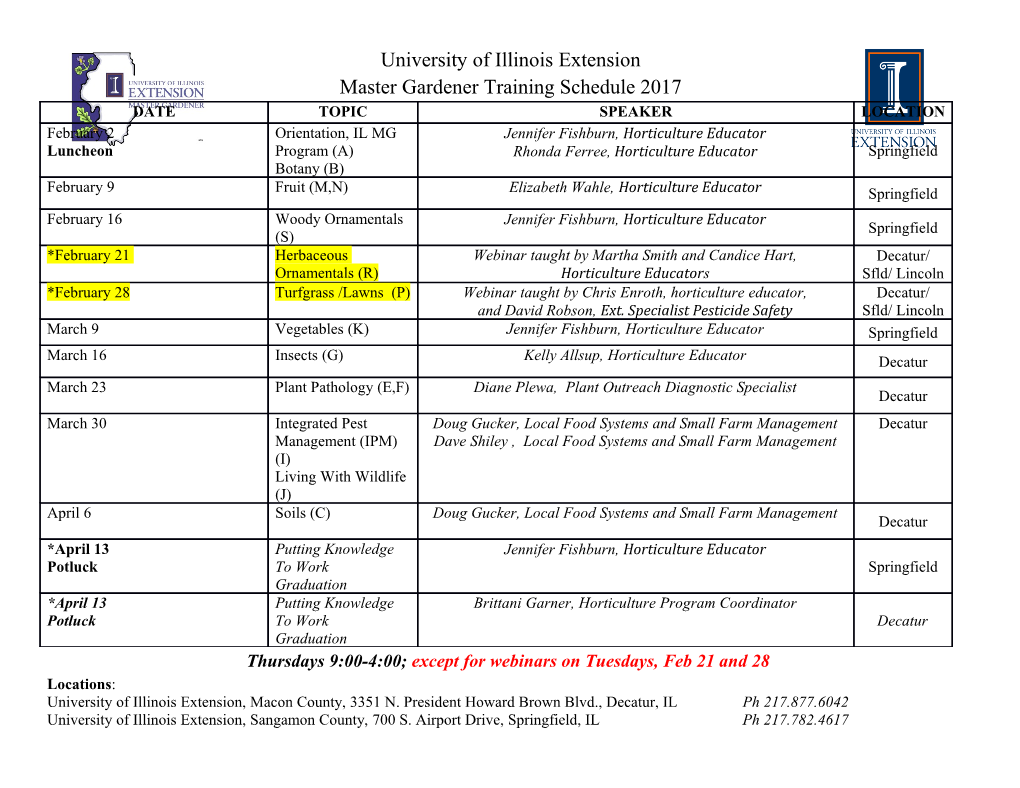
The Springer Series on Demographic Methods and Population Analysis 40 Anatoliy I. Yashin · Eric Stallard Kenneth C. Land Biodemography of Aging Determinants of Healthy Life Span and Longevity With contributions by Igor Akushevich · Liubov S. Arbeeva Konstantin G. Arbeev · Irina Culminskaya Mikhail Kovtun · Julia Kravchenko Alexander M. Kulminski · Frank A. Sloan Svetlana V. Ukraintseva · Deqing Wu The Springer Series on Demographic Methods and Population Analysis Volume 40 Series Editors Kenneth C. Land Biodemography of Aging Research Unit, Center for Population Health and Aging, Duke Population Research Institute & Social Science Research Institute at Duke University, Durham, NC, USA Department of Sociology, Duke University, Durham, NC, USA In recent decades, there has been a rapid development of demographic models and methods and an explosive growth in the range of applications of population analysis. This series seeks to provide a publication outlet both for high-quality textual and expository books on modern techniques of demographic analysis and for works that present exemplary applications of such techniques to various aspects of population analysis. Topics appropriate for the series include: • General demographic methods • Techniques of standardization • Life table models and methods • Multistate and multiregional life tables, analyses, and projections • Demographic aspects of biostatistics and epidemiology • Stable population theory and its extensions • Methods of indirect estimation • Stochastic population models • Event history analysis, duration analysis, and hazard regression models • Demographic projection methods and population forecasts • Techniques of applied demographic analysis, regional and local population estimates and projections • Methods of estimation and projection for business and health care applications • Methods and estimates for unique populations such as schools and students Volumes in the series are of interest to researchers, professionals, and students in demography, sociology, economics, statistics, geography and regional science, public health and health care management, epidemiology, biostatistics, actuarial science, business, and related fields More information about this series at http://www.springer.com/series/6449 Anatoliy I. Yashin • Eric Stallard Kenneth C. Land Biodemography of Aging Determinants of Healthy Life Span and Longevity With contributions by: Igor Akushevich Liubov S. Arbeeva Konstantin G. Arbeev Irina Culminskaya Mikhail Kovtun Julia Kravchenko Alexander M. Kulminski Frank A. Sloan Svetlana V. Ukraintseva Deqing Wu Anatoliy I. Yashin Eric Stallard Biodemography of Aging Research Unit, Biodemography of Aging Research Unit, Center for Population Health and Aging Center for Population Health and Aging Duke Population Research Institute Duke Population Research Institute & Social Science Research Institute & Social Science Research Institute at Duke University at Duke University Durham, NC, USA Durham, NC, USA Kenneth C. Land Biodemography of Aging Research Unit, Center for Population Health and Aging Duke Population Research Institute & Social Science Research Institute at Duke University Durham, NC, USA Department of Sociology Duke University Durham, NC, USA ISSN 1389-6784 ISSN 2215-1990 (electronic) The Springer Series on Demographic Methods and Population Analysis ISBN 978-94-017-7585-4 ISBN 978-94-017-7587-8 (eBook) DOI 10.1007/978-94-017-7587-8 Library of Congress Control Number: 2016934343 © Springer Science+Business Media B.V. 2016 This work is subject to copyright. All rights are reserved by the Publisher, whether the whole or part of the material is concerned, specifically the rights of translation, reprinting, reuse of illustrations, recitation, broadcasting, reproduction on microfilms or in any other physical way, and transmission or information storage and retrieval, electronic adaptation, computer software, or by similar or dissimilar methodology now known or hereafter developed. The use of general descriptive names, registered names, trademarks, service marks, etc. in this publication does not imply, even in the absence of a specific statement, that such names are exempt from the relevant protective laws and regulations and therefore free for general use. The publisher, the authors and the editors are safe to assume that the advice and information in this book are believed to be true and accurate at the date of publication. Neither the publisher nor the authors or the editors give a warranty, express or implied, with respect to the material contained herein or for any errors or omissions that may have been made. Printed on acid-free paper This Springer imprint is published by Springer Nature The registered company is Springer Science+Business Media B.V. Dordrecht Preface Some people live to age 100 years or more, others become sick and die prematurely. What factors determine human lifespan? Which biological mechanisms are involved in the regulation of health span and longevity? Which forces shape the age pattern and affect the time trend of human mortality? These questions constitute an emerging high priority research area because of the ever-increasing proportions of elderly individuals in most countries and the imperative to improve the health of these populations. Such improvements will require substantially better understand- ing of the regularities of individual aging-related changes in biological variables (biomarkers) that take place during the life course and their connections with aging- related declines in health and survival. Better understanding can be reached by proper integration of relevant informa- tion accumulated in the field with newly collected data. The data for such studies are readily available today. Many informative datasets have been collected in longitudinal studies of aging, health, and longevity. The dimensions and scope of such information will continually increase over time—resulting in a supermassive “Big Data” problem. Dealing with such data requires efficient approaches that allow the analysis not only of subsets of available data (which practically all traditional methods do) but also the analysis of the entire dataset within the scope of a single comprehensive framework. The goal of this monograph is to show how questions about the connections between and among aging, health, and longevity can be addressed using the wealth of available accumulated knowledge in the field, the large volumes of genetic and non-genetic data collected in longitudinal studies, and advanced biodemographic models and analytic methods. The distinguishing features of the aging-related declines in health and survival are the development of comorbidity and multimorbidity involving chronic diseases, medical conditions, frailties, and physical and cognitive impairments that are mutually dependent. The dynamic connections among trajectories of aging changes in biomarkers, risks of diseases, and mortality risks are important for evaluating long-term consequences of exposures to environmental factors, medical interven- tions, and other disturbances. This monograph visualizes aging-related changes in v vi Preface physiological variables and survival probabilities, describes methods, and summa- rizes the results of analyses of longitudinal data on aging, health, and longevity in humans performed by the group of researchers in the Biodemography of Aging Research Unit (BARU) at Duke University during the past decade. The process of individual aging involves dynamic relationships among biolog- ical variables and their connections to health and survival outcomes. These relation- ships are captured in longitudinal data. Therefore, the focus of this monograph is studying dynamic relationships between aging, health, and longevity characteristics using longitudinal datasets. A substantial part of this research is based on the idea of using a specific stochastic process model (SPM) of longitudinal data. This model was developed in the 1970s by Max A. Woodbury who worked at the Center for Demographic Studies, Duke University, together with Kenneth G. Manton and Eric Stallard. This model was further developed by the current research team during the past decade to include responses of physiological variables and other biomarkers to persistent external disturbances, aging-related decline in resistance to stresses, adaptive capacity, connections between health and mortality, etc. Another part of this research uses the Grade of Membership (GoM) model also developed in the 1970s at Duke University by Max A. Woodbury. To our knowl- edge this was one of the first attempts to address the “Big Data” problem. This model was also further developed by the current research team during the past two decades for application to longitudinal data on health and disability among the general elderly population, as well as for characterization of complex disease progression among Alzheimer’s disease patients. Recent development has focused on the relaxation of the GoM model assumptions and a broadening of scope of application under the Linear Latent Structure (LLS) paradigm. The research questions and related methods described above belong to the field of biodemography of aging—or, more narrowly, biomedical demography— because they link detailed biological and physiological information about aging- related changes in humans with population health and mortality characteristics. Demographers have always wanted to have a mortality model whose parameters could characterize the process of individual
Details
-
File Typepdf
-
Upload Time-
-
Content LanguagesEnglish
-
Upload UserAnonymous/Not logged-in
-
File Pages470 Page
-
File Size-