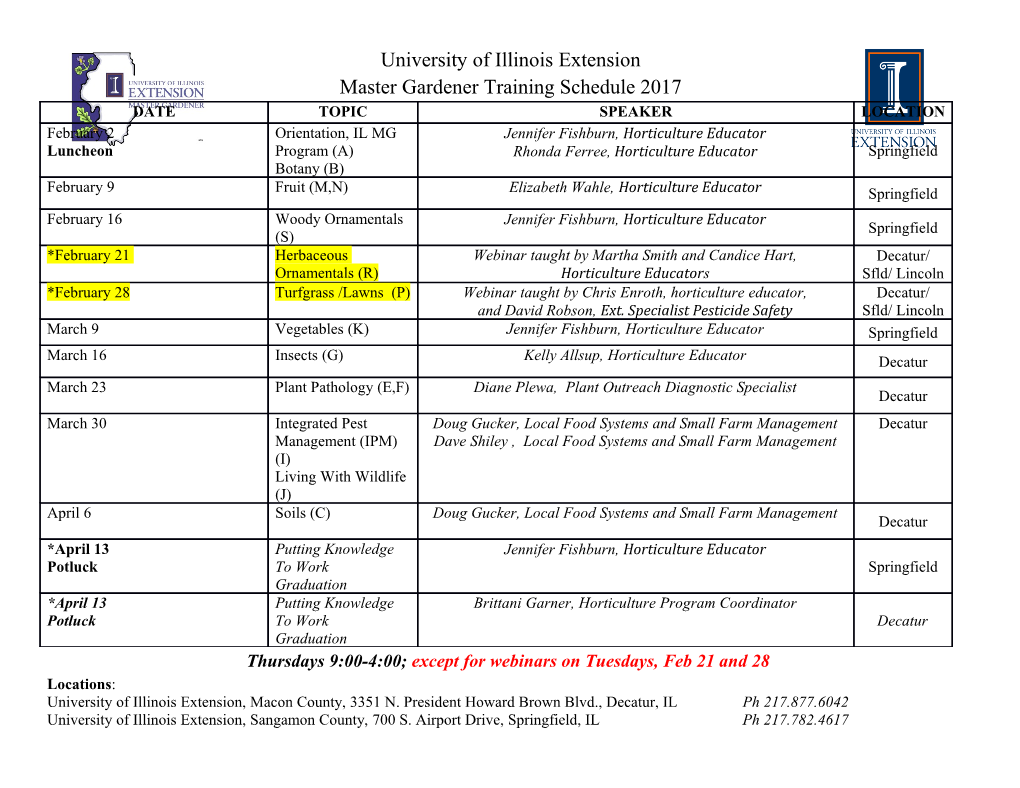
Bregman Divergence and Mirror Descent 1 Bregman Divergence Motivation • Generalize squared Euclidean distance to a class of distances that all share similar properties • Lots of applications in machine learning, clustering, exponential family Definition 1 (Bregman divergence) Let :Ω ! R be a function that is: a) strictly convex, b) continuously differentiable, c) defined on a closed convex set Ω. Then the Bregman divergence is defined as ∆ (x; y) = (x) − (y) − hr (y); x − yi ; 8 x; y 2 Ω: (1) That is, the difference between the value of at x and the first order Taylor expansion of around y evaluated at point x. Examples 1 2 1 2 • Euclidean distance. Let (x) = 2 kxk . Then ∆ (x; y) = 2 kx − yk . n P P P xi • Ω = x 2 : xi = 1 , and (x) = xi log xi. Then ∆ (x; y) = xi log for x; y 2 Ω. R+ i i i yi This is called relative entropy, or Kullback–Leibler divergence between probability distributions x and y. 1 1 1 2 1 2 1 2 • `p norm. Let p ≥ 1 and p + q = 1. (x) = 2 kxkq. Then ∆ (x; y) = 2 kxkq + 2 kykq − D 1 2E 1 2 x; r 2 kykq . Note 2 kykq is not necessarily continuously differentiable, which makes this case not precisely consistent with our definition. Properties of Bregman divergence • Strict convexity in the first argument x. Trivial by the strict convexity of . • Nonnegativity: ∆ (x; y) ≥ 0 for all x; y. ∆ (x; y) = 0 if and only if x = y. Trivial by strict convexity. • Asymmetry: in general, ∆ (x; y) 6= ∆ (y; x). Eg, KL-divergence. Symmetrization not always useful. • Non-convexity in the second argument. Let Ω = [1; 1), (x) = − log x. Then ∆ (x; y) = − log x + x−y 1 2x log y + y . One can check its second order derivative in y is y2 ( y − 1), which is negative when 2x < y. • Linearity in . For any a > 0, ∆ +a'(x; y) = ∆ (x; y) + a∆'(x; y). @ • Gradient in x: @x ∆ (x; y) = r (x) − r (y). Gradient in y is trickier, and not commonly used. • Generalized triangle inequality: ∆ (x; y) + ∆ (y; z) = (x) − (y) − hr (y); x − yi + (y) − (z) − hr (z); y − zi (2) = ∆ (x; z) + hx − y; r (z) − r (y)i : (3) • Special case: is called strongly convex with respect to some norm with modulus σ if σ (x) ≥ (y) + hr (y); x − yi + kx − yk2 : (4) 2 Note the norm here is not necessarily Euclidean norm. When the norm is Euclidean, this condition is σ 2 P equivalent to (x)− 2 kxk being convex. For example, the (x) = i xi log xi used in KL-divergence n P is 1-strongly convex over the simplex Ω = x 2 R+ : i xi = 1 , with respect to the `1 norm. When is σ strong convex, we have σ ∆ (x; y) ≥ kx − yk2 : (5) 2 σ 2 Proof: By definition ∆ (x; y) = (x) − (y) − hr (y); x − yi ≥ 2 kx − yk . • Duality. Suppose is strongly convex. Then ∗ (r )(r (x)) = x; ∆ (x; y) = ∆ ∗ (r (y); r (x)) : (6) Proof: (for the first equality only) Recall ∗(y) = sup fhz; yi − (z)g : (7) z2Ω sup must be attainable because is strongly convex and Ω is closed. x is a maximizer if and only if y = r (x). So ∗(y) + (x) = hx; yi , y = r (x): (8) Since = ∗∗, so ∗(y) + ∗∗(x) = hx; yi, which means y is the maximizer in ∗∗(x) = sup fhx; zi − ∗(z)g : (9) z This means x = r ∗(y). To summarize, (r ∗)(r (x)) = x. • Mean of distribution. Suppose U is a random variable over an open set S with distribution µ. Then min EU∼µ[∆ (U; x)] (10) x2S R is optimized at u¯ := Eµ[U] = u2S uµ(u). Proof: For any x 2 S, we have EU∼µ[∆ (U; x)] − EU∼µ[∆ (U; u¯)] (11) 0 0 = Eµ[ (U) − (x) − (U − x) r (x) − (U) + (¯u) + (U − u¯) r (¯u)] (12) 0 0 0 0 = (¯u) − (x) + x r (x) − u¯ r (¯u) + Eµ[−U r (x) + U r (¯u)] (13) = (¯u) − (x) − (¯u − x)0r (x) (14) = ∆ (¯u; x): (15) This must be nonnegative, and is 0 if and only if x =u ¯. ∗ • Pythagorean Theorem. If x is the projection of x0 onto a convex set C 2 Ω: ∗ x = argmin ∆ (x; x0): (16) x2C Then ∗ ∗ ∆ (y; x0) ≥ ∆ (y; x ) + ∆ (x ; x0): (17) ∗ In Euclidean case, it means the angle \yx x0 is obtuse. More generally Lemma 2 Suppose L is a proper convex function whose domain is an open set containing C. L is not necessarily differentiable. Let x∗ be ∗ ∗ x = argmin fL(x) + ∆ (x ; x0)g : (18) x2C Then for any y 2 C we have ∗ ∗ ∗ L(y) + ∆ (y; x0) ≥ L(x ) + ∆ (x ; x0) + ∆ (y; x ): (19) 2 The projection in (16) is just a special case of L = 0. This property is the key to the analysis of many optimization algorithms using Bregman divergence. ∗ Proof: Denote J(x) = L(x) + ∆ (x; x0). Since x minimizes J over C, there must exist a subgradient d 2 @J(x∗) such that hd; x − x∗i ≥ 0; 8 x 2 C: (20) ∗ ∗ ∗ ∗ Since @J(x ) = fg + rx=x∗ ∆ (x; x0): g 2 @L(x )g = fg + r (x ) − r (x0): g 2 @L(x )g. So there must be a subgradient g 2 L(x∗) such that ∗ ∗ hg + r (x ) − r (x0); x − x i ≥ 0; 8 x 2 C: (21) Therefore using the property of subgradient, we have for all y 2 C that L(y) ≥ L(x∗) + hg; y − x∗i (22) ∗ ∗ ∗ ≥ L(x ) + hr (x0) − r (x ); y − x i (23) ∗ ∗ ∗ = L(x ) − hr (x0); x − x0i + (x ) − (x0) (24) + hr (x0); y − x0i − (y) + (x0) (25) − hr (x∗); y − x∗i + (y) − (x∗) (26) ∗ ∗ ∗ = L(x ) + ∆ (x ; x0) − ∆ (y; x0) + ∆ (y; x ): (27) Rearranging completes the proof. 2 Mirror Descent Why bother? Because the rate of convergence of subgradient descent often depends on the dimension of the problem. Suppose we want to minimize a function f over a set C. Recall the subgradient descent rule x 1 = xk − αkgk; where gk 2 @f(xk) (28) k+ 2 2 1 1 2 xk+1 = argmin x − xk+ 1 = argmin kx − (xk − αkgk)k : (29) x2C 2 2 x2C 2 This can be interpreted as follows. First approximate f around xk by a first-order Taylor expansion f(x) ≈ f(xk) + hgk; x − xki : (30) 1 2 Then penalize the displacement by kx − xkk . So the update rule is to find a regularized minimizer of 2αk the model 1 2 xk+1 = argmin f(xk) + hgk; x − xki + kx − xkk : (31) x2C 2αk It is trivial to see this is exactly equivalent to (29). To generalize the method beyond Euclidean distance, it is straightforward to use the Bregman divergence as a measure of displacement: 1 xk+1 = argmin f(xk) + hgk; x − xki + ∆ (x; xk) (32) x2C αk = argmin fαkf(xk) + αk hgk; x − xki + ∆ (x; xk)g : (33) x2C Mirror descent interpretation. Suppose the constraint set C is the whole space (i.e. no constraint). Then we can take gradient with respect to x and find the optimality condition 1 gk + (r (xk+1) − r (xk)) = 0 (34) αk , r (xk+1) = r (xk) − αkgk (35) −1 ∗ , xk+1 = (r ) (r (xk) − αkgk) = (r )(r (xk) − αkgk): (36) For example, in KL-divergence over simplex, the update rule becomes xk+1(i) = xk(i) exp(−αkgk(i)): (37) Rate of convergence. Recall in unconstrained subgradient descent we followed 4 steps. 3 1. Bound on single update ∗ 2 ∗ 2 kxk+1 − x k2 = kxk − αkgk − x k2 (38) ∗ 2 ∗ 2 2 = kxk − x k2 − 2αk hgk; xk − x i + αk kgkk2 (39) ∗ 2 ∗ 2 2 ≤ kxk − x k2 − 2αk (f(xk) − f(x )) + αk kgkk2 : (40) 2. Telescope T T ∗ 2 ∗ 2 X ∗ X 2 2 kxT +1 − x k2 ≤ kx1 − x k2 − 2 αk (f(xk) − f(x )) + αk kgkk2 : (41) k=1 k=1 ∗ 2 2 2 2 3. Bounding by kx1 − x k2 ≤ R and kgkk2 ≤ G : T T X ∗ 2 2 X 2 2 αk (f(xk) − f(x )) ≤ R + G αk: (42) k=1 k=1 ∗ 4. Denote k = f(xk) − f(x ) and rearrange 2 2 PT 2 R + G k=1 αk min k ≤ : (43) k2f1;:::;T g PT 2 k=1 αk By setting the step size αk judiciously, we can achieve RG min k ≤ p : (44) k2f1;:::;T g T p Suppose C is the simplex.p Then R ≤ 2. If each coordinate of each gradient gi is upper bounded by M, then G can be at most M n, i.e. depends on the dimension. ∗ 2 ∗ Clearly the step 2 to 4 can be easily extended by replacing kxk+1 − x k2 with ∆ (x ; xk+1). So the only challenge left is to extend step 1. This is actually possible via Lemma2. We further assume is σ strongly convex. In (33), consider αk(f(xk) + hgk; x − xki) as the L in Lemma2. Then ∗ ∗ ∗ αk (f(xk) + hgk; x − xki) + ∆ (x ; xk) ≥ αk (f(xk) + hgk; xk+1 − xki) + ∆ (xk+1; xk) + ∆ (x ; xk+1) (45) Canceling some terms can rearranging, we obtain ∗ ∗ ∗ ∆ (x ; xk+1) ≤ ∆ (x ; xk) + αk hgk; x − xk+1i − ∆ (xk+1; xk) (46) ∗ ∗ = ∆ (x ; xk) + αk hgk; x − xki + αk hgk; xk − xk+1i − ∆ (xk+1; xk) (47) σ ≤ ∆ (x∗; x ) − α (f(x ) − f(x∗)) + α hg ; x − x i − kx − x k2 (48) k k k k k k k+1 2 k k+1 σ ≤ ∆ (x∗; x ) − α (f(x ) − f(x∗)) + α kg k kx − x k − kx − x k2 (49) k k k k k ∗ k k+1 2 k k+1 α2 ≤ ∆ (x∗; x ) − α (f(x ) − f(x∗)) + k kg k2 (50) k k k 2σ k ∗ ∗ 2 ∗ Now compare with (40), we have successfully replaced kxk+1 − x k2 with ∆ (x ; xi).
Details
-
File Typepdf
-
Upload Time-
-
Content LanguagesEnglish
-
Upload UserAnonymous/Not logged-in
-
File Pages8 Page
-
File Size-