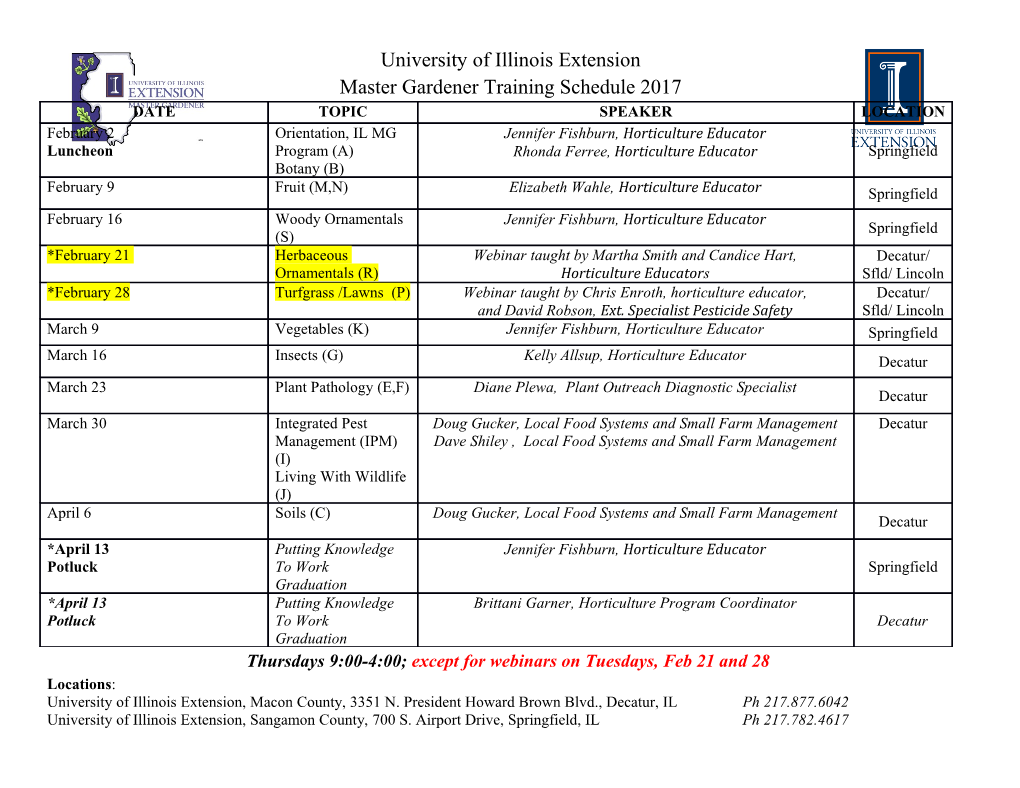
Identification of differential translation in genome wide studies Ola Larssona,1,2, Nahum Sonenberga, and Robert Nadonb,c,2 aDepartment of Biochemistry, McGill University, Montreal, Quebec H3A 1A3, Canada; bDepartment of Human Genetics, McGill University, Montreal, Quebec H3A 1B1, Canada; and cMcGill University and Genome Quebec Innovation Centre, Montreal, Quebec H3A 1A4, Canada Edited by Peter J. Bickel, University of California, Berkeley, CA, and approved October 26, 2010 (received for review May 20, 2010) Regulation of gene expression through translational control is a achieved by dividing actively translated mRNA levels by cytosolic fundamental mechanism implicated in many biological processes mRNA levels obtained in parallel and logging the ratios. We show ranging from memory formation to innate immunity and whose that these log ratios do not actually correct for cytosolic mRNA dysregulation contributes to human diseases. Genome wide ana- levels and that they consequently generate substantial numbers of lyses of translational control strive to identify differential transla- biological false positives and false negatives. Here we propose a tion independent of cytosolic mRNA levels. For this reason, most more sensitive and specific method for analysis of translational studies measure genes’ translation levels as log ratios (translation activity that includes analysis of partial variance (APV) linear levels divided by corresponding cytosolic mRNA levels obtained regression to control for cytosolic mRNA levels and a variance in parallel). Counterintuitively, arising from a mathematical neces- shrinkage method for improving statistical inference. sity, these log ratios tend to be highly correlated with the cytosolic mRNA levels. Accordingly, they do not effectively correct for cyto- Results and Discussion solic mRNA level and generate substantial numbers of biological Common Issues in Genome Wide Analysis of Translational Control. false positives and false negatives. We show that analysis of partial Two types of data are produced from each sample when studying variance, which produces estimates of translational activity that are translational activity: actively translated mRNAs (“translational independent of cytosolic mRNA levels, is a superior alternative. activity data”) and cytosolic mRNAs (“cytosolic mRNA data”) When combined with a variance shrinkage method for estimating obtained in parallel. Typically, log (translational activity data/ error variance, analysis of partial variance has the additional benefit cytosolic mRNA data) ratios are calculated (i.e., logged cytosolic of having greater statistical power and identifying fewer genes as mRNA data is subtracted from its associated logged translational translationally regulated resulting merely from unrealistically low activity data) with the idea of obtaining a cytosolic mRNA data- variance estimates rather than from large changes in translational corrected estimate of translation activity and compared between activity. In contrast to log ratios, this formal analytical approach classes (Fig. 1A). Because log ratios are differences between estimates translation effects in a statistically rigorous manner, logged values, class comparison effects are estimated from differ- eliminates the need for inefficient and error-prone heuristics, and ence scores. Log difference scores can be correlated with cytosolic produces results that agree with biological function. The method mRNA data, however, leading to incorrect biological conclusions. is applicable to datasets obtained from both the commonly used Such is the case when translational activity data and cytosolic polysome microarray method and the sequencing-based ribosome mRNA data are uncorrelated for technical or biological reasons profiling method. (Fig. 1 B and C). For example, false positives can arise from log ratios when mRNAs fail to reach the predetermined threshold for differential expression ∣ RIP-CHIP ∣ random variance model ∣ translatomics the number of ribosomes necessary to join the pool of actively translated mRNAs or when short poorly translated mRNAs, egulation of gene expression is a multistep process that but not their paired cytosolic mRNAs, fail to achieve counts Rincludes transcription, splicing, mRNA-export, -localization, for protected mRNA pieces above the noise level. Log ratios -stability, and -translation. Translational control of gene expres- for such mRNAs tend to produce false positives as they appear sion can be achieved by modulating translation elongation, ter- to be under translational control (Fig. 1B). False negatives can mination, or initiation, although the latter seems to be most be produced by log ratios when translational activity is regulated frequent (1). Thus, differential translation usually involves a independently of the cytosolic mRNA level (Fig. 1C). The phe- change in the number of ribosomes bound to each mRNA, lead- nomenon of a difference score (Y − Z) correlating with each of ing to a change in the amount of synthesized protein per mRNA its terms (Y and Z) was first described by Pearson in 1897 (7) molecule and time unit. This regulation can be accomplished by a who labeled it “spurious correlation” because of the frequent BIOPHYSICS AND specific process, which targets individual or sets of mRNAs for practice of interpreting such correlations as substantive rather regulation, or by a general process, which affects most mRNAs than artifactual. We will also refer to these correlations as spur- COMPUTATIONAL BIOLOGY equally (2). Specific translational control is important in many ious, although our focus will be on the inadequacy of difference biological processes, including cellular senescence (3) and dis- scores to control for cytosolic mRNA data and as a consequence eases (e.g., cancer) (4). fail to correctly estimate class effects (e.g., genotype, treatment, The polysome microarray approach is the most commonly disease). The following equation illustrates the mathematical used method for studying genome wide translational control. In this approach, mRNAs associated with several ribosomes >3 Author contributions: O.L., N.S., and R.N. designed research; O.L. performed research; (usually ) are separated from mRNAs associated with fewer O.L. analyzed data; and O.L., N.S., and R.N. wrote the paper. ribosomes and probed with microarrays. A more recent method, The authors declare no conflict of interest. ’ which circumvents some of the polysome microarray approach s This article is a PNAS Direct Submission. limitations (5), involves isolation and sequencing of RNA pieces 1Present address: Department of Oncology–Pathology, Cancer Center Karolinska, that are physically protected by ribosomes (6). A critical interpre- Karolinska Institute, R8:01, 171 76 Stockholm, Sweden. tative difficulty with both methods, however, is that observed 2To whom correspondence may be addressed. E-mail: [email protected] or robert.nadon@ differential levels of actively translated mRNAs or protected mcgill.ca. mRNA pieces may be due to differential cytosolic mRNA levels. This article contains supporting information online at www.pnas.org/lookup/suppl/ Correction for cytosolic mRNA level has historically been doi:10.1073/pnas.1006821107/-/DCSupplemental. www.pnas.org/cgi/doi/10.1073/pnas.1006821107 PNAS ∣ December 14, 2010 ∣ vol. 107 ∣ no. 50 ∣ 21487–21492 Downloaded by guest on September 24, 2021 AB C D E Fig. 1. (A–D) Common problems when analyzing translational activity F data. Data were simulated to generate two sample classes, each with five measurements of paired translational activity data (indicated as translation on each y-axis) and cytosolic mRNA data (indicated as tran- scription on each x-axis). The examples were generated to illustrate dif- ferent scenarios of translational control analysis. For each example, a two-tailed t-test was performed comparing the sample classes using the translational activity data only or the log-ratio data (p-values as in- dicated). anota was also performed on each example (p-value as indi- cated). Lines represent the regression lines estimated by anota for the two sample categories. (E) Common spurious correlations in published datasets of translational control. Shown are boxplots of the spurious correlations that emerge between the log (translational activity data/cytosolic mRNA data) ratios and log cytosolic mRNA data. (F) Small overlap between the log-ratio approach and anota. The top 5% genes ranked by significance from the log-ratio approach and anota were col- lected. The overlap between the log-ratio approach and anota is visua- lized using Venn diagrams for the three example studies. 21488 ∣ www.pnas.org/cgi/doi/10.1073/pnas.1006821107 Larsson et al. Downloaded by guest on September 24, 2021 necessity underlying the problem (8): surements do not, by definition, show spurious correlations. We have implemented this approach in the anota (analysis of 2 rYZsY sZ − sZ translational activity) R-package. In anota, a common slope for rðY−ZÞZ ¼ qffiffiffiffiffiffiffiffiffiffiffiffiffiffiffiffiffiffiffiffiffiffiffiffiffiffiffiffiffiffiffiffiffiffiffiffiffiffi [1] s s2 þ s2 − 2r s s all sample categories is identified for each gene from the least Z Y Z YZ Y Z squares linear regression of translational activity data on cytosolic mRNA data. Class comparison effects are estimated by calculat- where Y is a vector of translational activity data for a specific ing differences between sample category intercepts; the sum of mRNA, Z is a vector of paired cytosolic mRNA data for the same squares error for these comparisons is reduced by the
Details
-
File Typepdf
-
Upload Time-
-
Content LanguagesEnglish
-
Upload UserAnonymous/Not logged-in
-
File Pages6 Page
-
File Size-