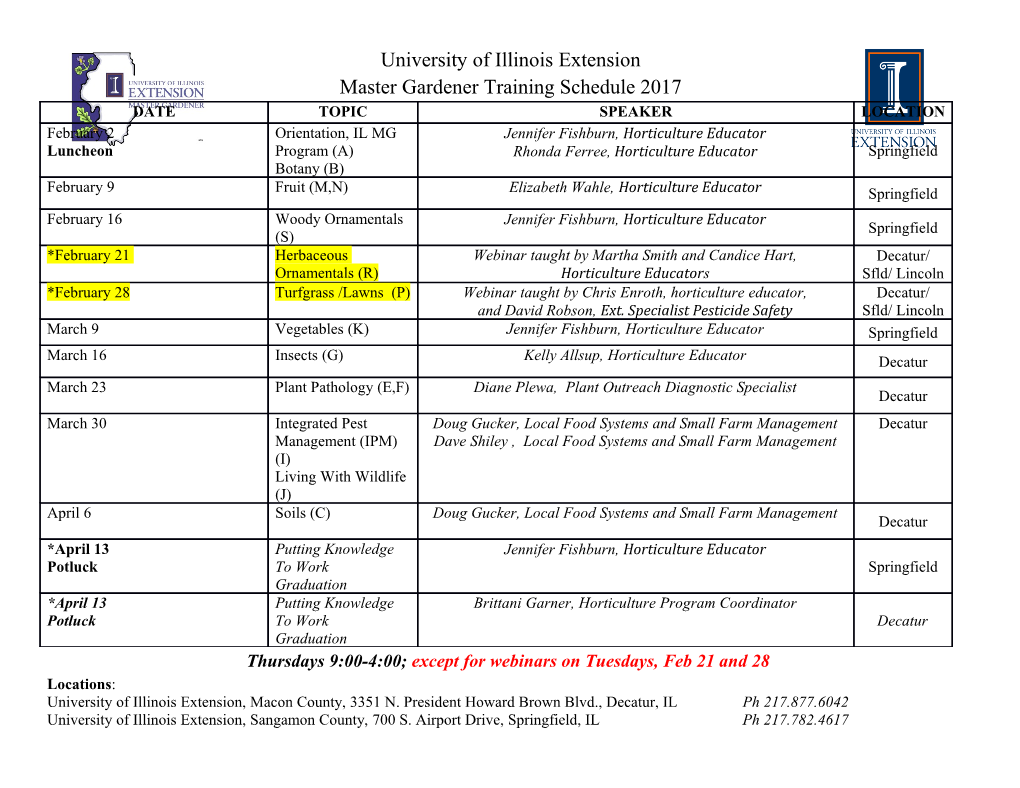
A Whole that may be Greater than the Sum of its Parts: A Balanced Mind from Biased Processes Thesis Presented in Partial Fulfillment of the Requirements for the Degree Master of Arts in the Graduate School of The Ohio State University By Adam Edward Hasinski, B.A. Graduate Program in Psychology The Ohio State University 2010 Thesis Committee: William A. Cunningham, Advisor Gary G. Berntson Russell H. Fazio Copyright by Adam Edward Hasinski 2010 Abstract We propose that evaluative biases, often taken as evidence for the fallibility of human judgment, serve as valuable heuristics, as long as uncertainty is high, by allowing organisms to safely learn about their environment. As uncertainty diminishes via experience, these biases lose their utility and should also wane. Further, we argue that multiple evaluative biases may work in dynamic opposition to produce individuals with balanced and flexible decision-making abilities. These hypotheses were tested using reinforcement learning models to predict subject’s evaluative representations in a newly designed learning task. These computational models indicate that multiple biased decision-making processes influence evaluation under uncertainty. Further our models suggest that these biases act antagonistically towards one another. Finally, our last model indicates that these biases decrease with increasing exposure to the learning environment. The implications of these findings are discussed. ii Dedication Dedicated to my parents, Ed and Linda Hasinski iii Acknowledgements I would like to thank all the former and current members of the Social, Cognitive & Affective Neuroscience Lab—Nathan Arbuckle, Andrew Jahn, Ingrid Johnsen, Tabitha Kirkland, Samantha Mowrer, Dominic Packer, and Jay Van Bavel—for their endless supply of support, patience, and baked goods. I would also like to thank my research assistants—Geetha Athanki, Anna Preston, and Jessica Stum—for their help in collecting data. I am grateful for the insightful comments and words of encouragement from various students and faculty within OSU’s psychology department. I am also grateful to my family for their never-ending support, understanding and confidence in me. Finally, I would like to thank my advisor, Wil Cunningham, for his encouragement, his contagious enthusiasm, and his ability to always see the bright side. iv Vita 2008........................................B.A. Psychology & History, Ohio University 2008-2009...............................Graduate Fellow, The Ohio State University 2009-present...........................Graduate Teaching Associate, The Ohio State University Publications 1. Ratcliff, J. J., Lassiter, G. D., Jager, V. M., Lindberg, M. J., Elek, J. K., Hasinski, A. E. (2010). The Hidden Consequences of Racial Salience in Videotaped Interrogations and Confessions. Psychology, Public Policy & Law, 16, 2, 200-218 Fields of Study Major Field: Psychology v Table of Contents Page Abstract................................................................................................................................ii Dedication...........................................................................................................................iii Acknowledgements.............................................................................................................iv Vita.......................................................................................................................................v Table of Contents................................................................................................................vi List of Tables.....................................................................................................................vii List of Figures...................................................................................................................viii Chapters: 1. Introduction..............................................................................................................1 2. Method...................................................................................................................21 3. Behavioral Analysis...............................................................................................25 4. Model 1: Results & Discussion............................................................................27 5. Model 2: Results & Discussion............................................................................29 6. Model 3: Results & Discussion...........................................................................33 7. General Discussion................................................................................................35 List of References..............................................................................................................46 Appendix A: Tables and Figures.......................................................................................53 vi List of Tables Table Page 1 Reinforcement learning equations.........................................................................54 vii List of Figures Figure Page 1 Conceptual overview of the computational modeling process..............................55 2 Example trial from the value estimation task........................................................56 3 Single subject value output from Model 1…………………................................57 viii Chapter 1: Introduction Attitudes and evaluations help individuals select appropriate actions in a complicated, ever-changing and often dangerous world. Attitudes are stores of information, derived from experience, used for the purpose of making evaluative judgments about a given object (e.g. Ajzen, 1991; Cunningham, Zelazo, Packer & Van Bavel, 2007; Fazio & Olson, 2003). For the development of accurate attitude representations, they must be updatable as new information from the environment becomes available. The utility of evaluations depends on their ability to correctly influence behaviors. Therefore, attitudes and the evaluations they generate form a circuit with behavior and the environment. Attitudes form the basis of the evaluations that guide behavioral interactions with the environment. The consequences of these interactions then update attitudes. In attempting to delineate the boundaries of human cognitive performance, considerable attention has been focused on biases. Broadly speaking, biases refer to the systematic errors in the result of some mental function. The study of bias not only highlights the limitations of human reasoning, but also has the potential to also elucidate reasoning’s component mechanisms. Much like vision researchers use instances of vision failure—via optical illusions—to piece together the visual process, decision researchers can use evaluative biases to better understand the processes underlying 1 human decision-making ability (e.g. Kahneman & Tversky, 1979). Of course, in the real world, determining what information is relevant can be an exceedingly difficult matter, for subject and researcher alike. In response to the murky nature of reality, experimental psychologists have constructed a variety of artificial contexts that clearly, in the eyes of the experimenter, distinguish the relevant from the irrelevant. After decades of research, it’s no longer contentious to claim the human mind contains, or is laden with, bias. Various research camps have made great strides understanding what biases exist, in what contexts they operate, and what function—if any—they serve (e.g. Tversky & Kahneman, 1992). Though research into evaluative biases is far from exhaustive, the general wealth of research findings supporting the existence of biases has, in the minds of some, painted a bleak picture of human evaluative abilities. What are we to make of human cognitive abilities in the face of such evidence? Does the extant research suggest that the human mind at its core is—much to the chagrin of economists—a hopelessly irrational machine? Though various camps have argued against the existence of such irrationality (e.g. Gigerenzer & Goldstein, 1996), the argument often becomes one of semantics, hinging on one’s precise definition of rationality. At best, the evidence from these various researchers tends to further elucidate the conditions under which a given bias will or won’t manifest itself, thereby attenuating the claims of the bias-supporters without repudiating their essence (Gigerenzer 2006, Todd & Gigerenzer, 2000). How then does this body of literature fit in with the well- known truism that humans have survived a hostile and changing world for hundreds of millennia? The answer: biased processes are not necessarily useless or even defective; in 2 contrast, their biased nature may prove to be useful. This has been the often-articulated explanation for the well-documented negativity bias (e.g. Baumeister, et al., 2001). While intuitively appealing, the claim is incredibly difficult to verify or falsify. Furthermore, the claim appears less solid under rigorous logical scrutiny. The existence of biases are often explained by gesturing to evolution and suggesting, seemingly logically, that such a bias would increase the fitness of organisms who possess it. Ironically, this argument could be spun to fit a valence asymmetry in either direction. That is one may argue, in line with the majority of the literature, that “it is better to be safe than sorry;” therefore, negativity biases, serve to guide organisms away from potentially fatal dangers. Conversely one could
Details
-
File Typepdf
-
Upload Time-
-
Content LanguagesEnglish
-
Upload UserAnonymous/Not logged-in
-
File Pages66 Page
-
File Size-