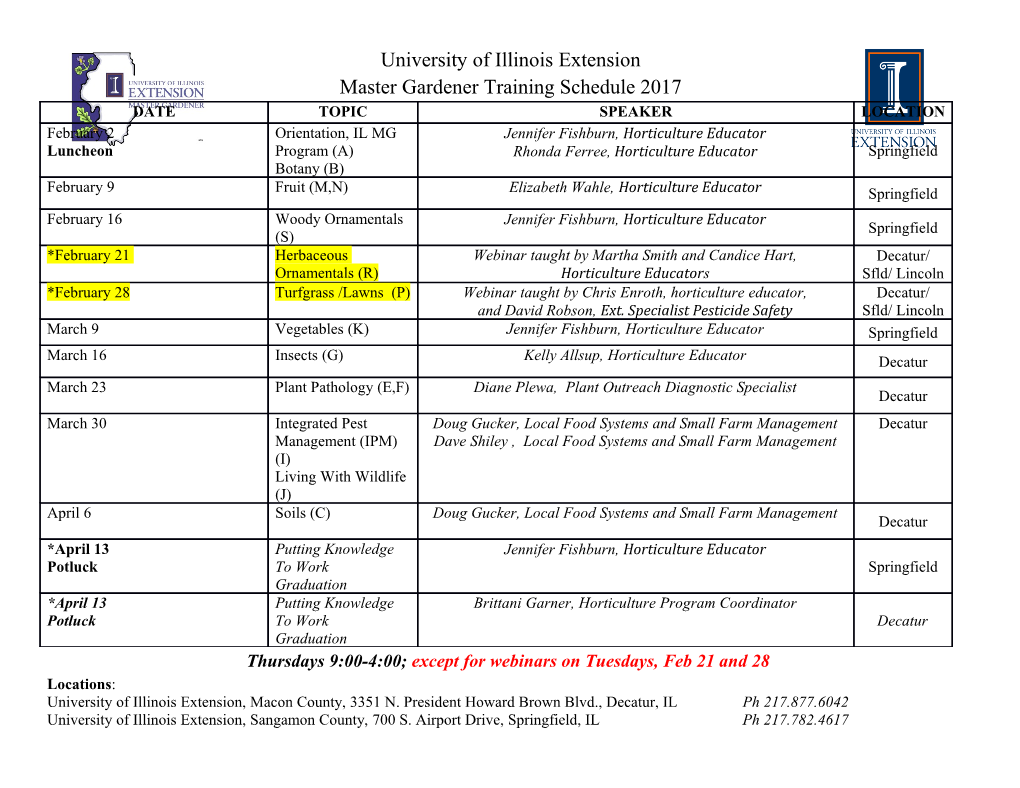
ISSN 1932-6157 (print) ISSN 1941-7330 (online) THE ANNALS of APPLIED STATISTICS AN OFFICIAL JOURNAL OF THE INSTITUTE OF MATHEMATICAL STATISTICS Articles Estimating the rate constant from biosensor data via an adaptive variational Bayesian approach....................YE ZHANG,ZHIGANG YAO,PATRIK FORSSÉN AND TORGNY FORNSTEDT 2011 Estimating abundance from multiple sampling capture-recapture data via a multi-state multi-period stopover model . .... HANNAH WORTHINGTON,RACHEL MCCREA, RUTH KING AND RICHARD GRIFFITHS 2043 Robust elastic net estimators for variable selection and identification of proteomic biomarkers..................GABRIELA V. C OHEN FREUE,DAV I D KEPPLINGER, MATÍAS SALIBIÁN-BARRERA AND EZEQUIEL SMUCLER 2065 Statistical inference for partially observed branching processes with application to cell lineage tracking of in vivo hematopoiesis JASON XU,SAMSON KOELLE,PETER GUTTORP,CHUANFENG WU, CYNTHIA DUNBAR,JANIS L. ABKOWITZ AND VLADIMIR N. MININ 2091 Fire seasonality identification with multimodality tests JOSE AMEIJEIRAS-ALONSO,AKLI BENALI,ROSA M. CRUJEIRAS, ALBERTO RODRÍGUEZ-CASAL AND JOSÉ M. C. PEREIRA 2120 Joint model of accelerated failure time and mechanistic nonlinear model for censored covariates,withapplicationinHIV/AIDS.......HONGBIN ZHANG AND LANG WU 2140 Prediction of small area quantiles for the conservation effects assessment project using a mixed effects quantile regression model . ..... EMILY BERG AND DANHYANG LEE 2158 Microsimulation model calibration using incremental mixture approximate Bayesian computation........CAROLYN M. RUTTER,JONATHAN OZIK,MARIA DEYOREO AND NICHOLSON COLLIER 2189 Principal nested shape space analysis of molecular dynamics data. .....IAN L. DRYDEN, KWANG-RAE KIM,CHARLES A. LAUGHTON AND HUILING LE 2213 Spatial modeling of trends in crime over time in Philadelphia CECILIA BALOCCHI AND SHANE T. JENSEN 2235 Fitting a deeply nested hierarchical model to a large book review dataset using a moment-basedestimator....................NINGSHAN ZHANG,KYLE SCHMAUS AND PATRICK O. PERRY 2260 Objective Bayes model selection of Gaussian interventional essential graphs for the identificationofsignalingpathways.....................FEDERICO CASTELLETTI AND GUIDO CONSONNI 2289 A latent discrete Markov random field approach to identifying and classifying historical forest communities based on spatial multivariate tree species counts STEPHEN BERG,JUN ZHU,MURRAY K. CLAYTON,MONIKA E. SHEA AND DAV I D J. MLADENOFF 2312 A nonparametric spatial test to identify factors that shape a microbiome SUSHEELA P. S INGH,ANA-MARIA STAICU,ROBERT R. DUNN,NOAH FIERER AND BRIAN J. REICH 2341 Vol. 13, No. 4—December 2019 ISSN 1932-6157 (print) ISSN 1941-7330 (online) THE ANNALS of APPLIED STATISTICS AN OFFICIAL JOURNAL OF THE INSTITUTE OF MATHEMATICAL STATISTICS Articles—Continued from front cover Predicting paleoclimate from compositional data using multivariate Gaussian process inverse prediction . JOHN R. TIPTON,MEVIN B. HOOTEN,CONNOR NOLAN, ROBERT K. BOOTH AND JASON MCLACHLAN 2363 Propensity score weighting for causal inference with multiple treatments FAN LI AND FAN LI 2389 Outline analyses of the called strike zone in Major League Baseball DALE L. ZIMMERMAN,JUN TANG AND RUI HUANG 2416 Empirical Bayes analysis of RNA sequencing experiments with auxiliary information KUN LIANG 2452 New formulation of the logistic-Gaussian process to analyze trajectory tracking data........GIANLUCA MASTRANTONIO,CLARA GRAZIAN,SARA MANCINELLI AND ENRICO BIBBONA 2483 A simple, consistent estimator of SNP heritability from genome-wide association studies.........ARMIN SCHWARTZMAN,ANDREW J. SCHORK,RONG ZABLOCKI AND WESLEY K. THOMPSON 2509 A hierarchical curve-based approach to the analysis of manifold data LIBERTY VITTERT,ADRIAN W. BOWMAN AND STANISLAV KATINA 2539 Scalable high-resolution forecasting of sparse spatiotemporal events with kernel methods: A winning solution to the NIJ “Real-Time Crime Forecasting Challenge” SETH FLAXMAN,MICHAEL CHIRICO,PAU PEREIRA AND CHARLES LOEFFLER 2564 On Bayesian new edge prediction and anomaly detection in computer networks SILVIA METELLI AND NICHOLAS HEARD 2586 Bayesian indicator variable selection to incorporate hierarchical overlapping group structure in multi-omics applications . LI ZHU,ZHIGUANG HUO,TIANZHOU MA, STEFFI OESTERREICH AND GEORGE C. TSENG 2611 Hierarchical infinite factor models for improving the prediction of surgical complications forgeriatricpatients....................ELIZABETH LORENZI,RICARDO HENAO AND KATHERINE HELLER 2637 A general theory for preferential sampling in environmental networks JOE WATSON,JAMES V. Z IDEK AND GAV I N SHADDICK 2662 Vol. 13, No. 4—December 2019 THE ANNALS OF APPLIED STATISTICS Vol. 13, No. 4, pp. 2011–2700 December 2019 INSTITUTE OF MATHEMATICAL STATISTICS (Organized September 12, 1935) The purpose of the Institute is to foster the development and dissemination of the theory and applications of statistics and probability. IMS OFFICERS President: Susan Murphy, Department of Statistics, Harvard University, Cambridge, Massachusetts 02138-2901, USA President-Elect: Regina Y. Liu, Department of Statistics, Rutgers University, Piscataway, New Jersey 08854-8019, USA Past President: Xiao-Li Meng, Department of Statistics, Harvard University, Cambridge, Massachusetts 02138-2901, USA Executive Secretary: Edsel Peña, Department of Statistics, University of South Carolina, Columbia, South Carolina 29208-001, USA Treasurer: Zhengjun Zhang, Department of Statistics, University of Wisconsin, Madison, Wisconsin 53706-1510, USA Program Secretary: Ming Yuan, Department of Statistics, Columbia University, New York, NY 10027-5927, USA IMS PUBLICATIONS The Annals of Statistics. Editors: Richard J. Samworth, Statistical Laboratory, Centre for Mathematical Sciences, University of Cambridge, Cambridge, CB3 0WB, UK. Ming Yuan, Department of Statistics, Columbia University, New York, NY 10027, USA The Annals of Applied Statistics. Editor-In-Chief : Karen Kafadar, Department of Statistics, University of Virginia, Heidelberg Institute for Theoretical Studies, Charlottesville, VA 22904-4135, USA The Annals of Probability. Editor: Amir Dembo, Department of Statistics and Department of Mathematics, Stan- ford University, Stanford, California 94305, USA The Annals of Applied Probability. Editors: François Delarue, Laboratoire J. A. Dieudonné, Université de Nice Sophia-Antipolis, France-06108 Nice Cedex 2. Peter Friz, Institut für Mathematik, Technische Universität Berlin, 10623 Berlin, Germany and Weierstrass-Institut für Angewandte Analysis und Stochastik, 10117 Berlin, Germany Statistical Science. Editor: Cun-Hui Zhang, Department of Statistics, Rutgers University, Piscataway, New Jersey 08854, USA The IMS Bulletin. Editor: Vlada Limic, UMR 7501 de l’Université de Strasbourg et du CNRS, 7 rue René Descartes, 67084 Strasbourg Cedex, France The Annals of Applied Statistics [ISSN 1932-6157 (print); ISSN 1941-7330 (online)], Volume 13, Number 4, December 2019. Published quarterly by the Institute of Mathematical Statistics, 3163 Somerset Drive, Cleveland, Ohio 44122, USA. Periodicals postage pending at Cleveland, Ohio, and at additional mailing offices. POSTMASTER: Send address changes to The Annals of Applied Statistics, Institute of Mathematical Statistics, Dues and Subscriptions Office, 9650 Rockville Pike, Suite L 2310, Bethesda, Maryland 20814-3998, USA. Copyright © 2019 by the Institute of Mathematical Statistics Printed in the United States of America The Annals of Applied Statistics 2019, Vol. 13, No. 4, 2011–2042 https://doi.org/10.1214/19-AOAS1263 © Institute of Mathematical Statistics, 2019 ESTIMATING THE RATE CONSTANT FROM BIOSENSOR DATA VIA AN ADAPTIVE VARIATIONAL BAYESIAN APPROACH ∗ BY YE ZHANG ,1,ZHIGANG YAO†,2,4,PATRIK FORSSÉN‡,3 AND TORGNY FORNSTEDT‡,3 Chemnitz University of Technology∗, National University of Singapore† and Karlstad University‡ The means to obtain the rate constants of a chemical reaction is a funda- mental open problem in both science and the industry. Traditional techniques for finding rate constants require either chemical modifications of the reac- tants or indirect measurements. The rate constant map method is a modern technique to study binding equilibrium and kinetics in chemical reactions. Finding a rate constant map from biosensor data is an ill-posed inverse prob- lem that is usually solved by regularization. In this work, rather than finding a deterministic regularized rate constant map that does not provide uncertainty quantification of the solution, we develop an adaptive variational Bayesian approach to estimate the distribution of the rate constant map, from which some intrinsic properties of a chemical reaction can be explored, including information about rate constants. Our new approach is more realistic than the existing approaches used for biosensors and allows us to estimate the dynam- ics of the interactions, which are usually hidden in a deterministic approx- imate solution. We verify the performance of the new proposed method by numerical simulations, and compare it with the Markov chain Monte Carlo algorithm. The results illustrate that the variational method can reliably cap- ture the posterior distribution in a computationally efficient way. Finally, the developed method is also tested on the real biosensor data (parathyroid hor- mone), where we provide two novel analysis tools—the thresholding contour map and the high order moment map—to estimate the number of interactions as well as their rate constants. REFERENCES ALTSCHUH,D.,BJÖKELUND,H.,STRANDGÅRD,J.,CHOULIER,L.,MALMQVIST,M.andAN-
Details
-
File Typepdf
-
Upload Time-
-
Content LanguagesEnglish
-
Upload UserAnonymous/Not logged-in
-
File Pages89 Page
-
File Size-