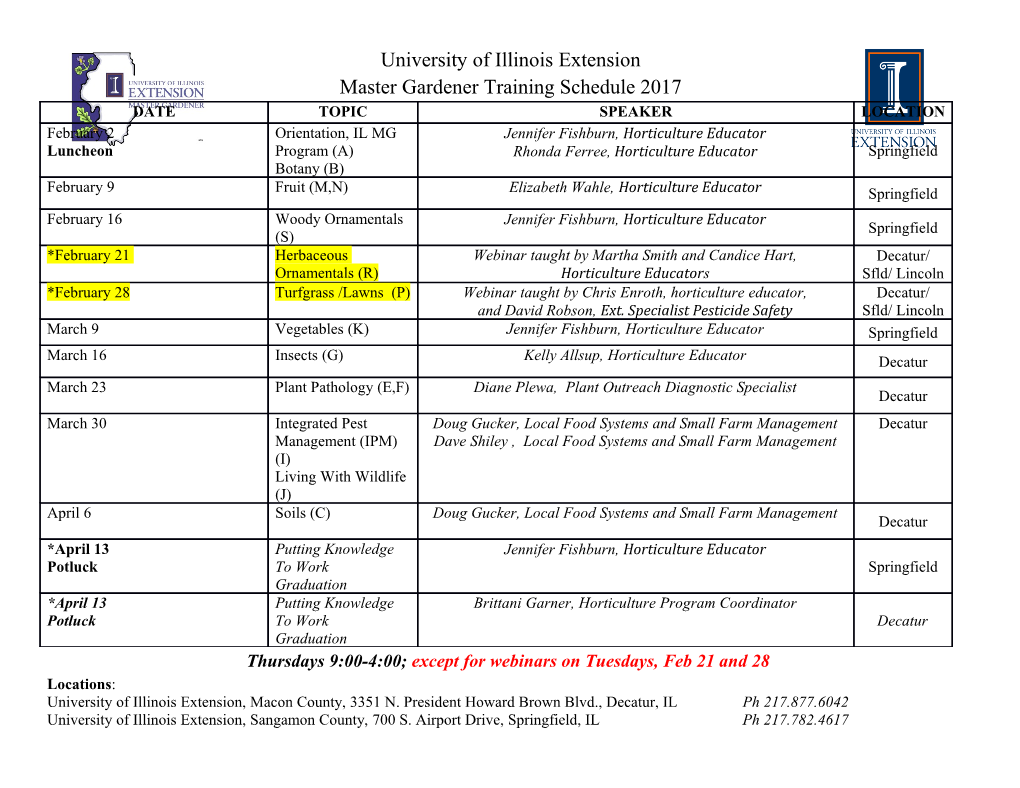
Introduction Method of Moments Procedure Mark and Recapture Monsoon Rains Chapter 7 Point Estimation Method of Moments 1 / 23 Introduction Method of Moments Procedure Mark and Recapture Monsoon Rains Outline Introduction Classical Statistics Method of Moments Procedure Mark and Recapture Monsoon Rains 2 / 23 Introduction Method of Moments Procedure Mark and Recapture Monsoon Rains Parameter Estimation For parameter estimation, we consider X = (X1;:::; Xn), independent random variables chosen according to one of a family of probabilities Pθ where θ is element from the parameter spaceΘ. Based on our analysis, we choose an estimator θ^(X ). If the data x takes on the values x1; x2;:::; xn, then θ^(x1; x2;:::; xn) is called the estimate of θ. Thus we have three closely related objects. 1. θ - the parameter, an element of the parameter space, is a number or a vector. 2. θ^(x1; x2;:::; xn) - the estimate, is a number or a vector obtained by evaluating the estimator on the data x = (x1; x2;:::; xn). 3. θ^(X1;:::; Xn) - the estimator, is a random variable. We will analyze the distribution of this random variable to decide how well it performs in estimating θ. 3 / 23 Introduction Method of Moments Procedure Mark and Recapture Monsoon Rains Classical Statistics In classical statistics, the state of nature is assumed to be fixed, but unknown to us. Thus, one goal of estimation is to determine which of the Pθ is the source of the data. The estimate is a statistic θ^ : data ! Θ: For estimation procedures, the classical approach to statistics is based on two fundamental questions: • How do we determine estimators? • How do we evaluate estimators? • Does this estimator in any way systematically under or over estimate the parameter? • Does it has large or small variance? • How does it compare to a notion of best possible estimator? • How easy is it to determine and to compute? • How does the procedure improve with increased sample size? 4 / 23 Introduction Method of Moments Procedure Mark and Recapture Monsoon Rains Method of Moments Method of moments estimation is based solely on the law of large numbers, Let M1; M2;::: be independent random variables having a common distribution possessing a mean µM . Then the sample means converge to the distributional mean as the number of observations increase. n 1 X M¯ = M ! µ as n ! 1 n n i M i=1 almost surely and in mean. In addition, if the random variables in this sequence fail to have a mean, then the limit will fail to exist. 5 / 23 Introduction Method of Moments Procedure Mark and Recapture Monsoon Rains Procedure • Step 1. If the model is based on a parametric family of densities fX (xjθ) with a d-dimensional parameter space( θ1; θ2 : : : ; θd ), we compute Z m m µm = EX = km(θ) = x fX (xjθ) ν(dx); m = 1;:::; d S the first d moments, µ1 = k1(θ1; θ2 : : : ; θd ); µ2 = k2(θ1; θ2 : : : ; θd ); : : : ; µd = kd (θ1; θ2 : : : ; θd ); obtaining d equations in d unknowns. 6 / 23 Introduction Method of Moments Procedure Mark and Recapture Monsoon Rains Example Let X1; X2;:::; Xn be a simple random sample of Pareto random variables with density β f (xjβ) = ; x > 1; β > 0: X xβ+1 The cumulative distribution function is −β FX (x) = 1 − x ; x > 1: The mean and the variance are, respectively, β β µ = ; σ2 = : β − 1 (β − 1)2(β − 2) In this situation, we have one parameter, namely β. Thus, in step 1, we will only need to determine the first moment β µ = µ = k (β) = 1 1 β − 1 ^ to find the method of moments estimator β for β. 7 / 23 Introduction Method of Moments Procedure Mark and Recapture Monsoon Rains Procedure • Step 2. We then solve for the d parameters as a function of the moments. θ1 = g1(µ1; µ2; ··· ; µd ); θ2 = g2(µ1; µ2; ··· ; µd ); : : : ; θd = gd (µ1; µ2; ··· ; µd ): • Step 3. Now, based on the data x = (x1; x2;:::; xn), we compute the first d sample moments, n n n 1 X 1 X 1 X x = x ; x2 = x2;:::; xd = xd : n i n i n i i=1 i=1 i=1 8 / 23 Introduction Method of Moments Procedure Mark and Recapture Monsoon Rains Example Exercise. If β µ µ = ; show that β = : β − 1 µ − 1 For step 2, we solve for β as a function of the mean µ. µ β = g (µ) = : 1 µ − 1 For step 3, we compute the sample mean n 1 X x = x : n i i=1 9 / 23 Introduction Method of Moments Procedure Mark and Recapture Monsoon Rains Procedure • Step 4. Using the law of large numbers, we have, for each moment, m = 1;:::; d, that m µm ≈ x : For the equations derived in step 2, we replace the distributional moments µm by the sample moments xm to give us formulas for the method of moment estimates (θ^1; θ^2;:::; θ^d ): For the data x, these estimates are 2 d 2 d θ^1(x) = g1(¯x; x ; ··· ; x ); θ^2(x) = g2(¯x; x ; ··· ; x ); 2 d :::; θ^d (x) = gd (¯x; x ; ··· ; x ): 10 / 23 Introduction Method of Moments Procedure Mark and Recapture Monsoon Rains Example Consequently, a method of moments estimate for β is obtained by replacing the distributional mean µ by the sample mean¯x in the equation for g1(µ). Thus, x¯ β^ = : x¯ − 1 A good estimator should have a small variance. To use the delta method to estimate the variance of β^, σ2 σ2 ≈ g 0 (µ)2 : β^ 1 n We compute µ 1 g (µ) = and so g 0 (µ) = − 1 µ − 1 1 (µ − 1)2 giving β 1 (β − 1)2 g 0 = − = − = −(β − 1)2: 1 β − 1 β 2 (β − (β − 1))2 ( β−1 − 1) 11 / 23 Introduction Method of Moments Procedure Mark and Recapture Monsoon Rains Example We find that β^ has mean approximately equal to β and variance σ2 β β(β − 1)2 σ2 ≈ g 0 (µ)2 = (β − 1)4 = : β^ 1 n n(β − 1)2(β − 2) n(β − 2) Let's consider the case with β = 4 and n = 225. Then, p 4 · 32 36 2 2 σ2 ≈ = = ; σ ≈ = 0:283: β^ 225 · 2 450 25 β^ 5 To simulate, we use the probability transform −β −1/β pβ u = FX (x) = 1 − x ; then x = (1 − u) = 1= (1 − u): pβ pβ Note that if Ui are U(0; 1) random variables, then1 = (1 − U1); 1= (1 − U2); ··· have the appropriate Pareto distribution. 12 / 23 Introduction Method of Moments Procedure Mark and Recapture Monsoon Rains Example > paretobar<-rep(0,1000) > for (i in 1:1000){u<-runif(225); Histogram of betahat pareto<-1/(1-u)^(1/4); paretobar[i]<-mean(pareto)} > betahat<-paretobar/(paretobar-1) 0.15 > mean(betahat) [1] 4.03508 0.10 > sd(betahat) Density [1] 0.2833142 0.05 Note that the mean is above4, but the 0.00 standard deviation is very close to the 0 5 10 15 value given by the delta method. betahat Exercise. Reproduce the simulation Figure: 1000 simulations for the method of above and compare. Simulate using a moments estimate for the case β = 4. different value for β. 13 / 23 Introduction Method of Moments Procedure Mark and Recapture Monsoon Rains Mark and Recapture The Lincoln-Peterson method of mark and recapture • The size of an animal population in a habitat of interest is an important question in conservation biology. • In many case, individuals are often too difficult to find and a census is not feasible. • One estimation technique is to capture some of the animals, mark them and release them back into the wild to mix randomly with the population. • Some time later, a second capture from the population is made. 14 / 23 Introduction Method of Moments Procedure Mark and Recapture Monsoon Rains Mark and Recapture Some of the animals were not in the first capture and some, which are tagged, are recaptured. Let • t be the number captured and tagged, • k be the number in the second capture, • r be the number in the second capture that are tagged, and let • N be the total population size. Thus, t and k is under the control of the experimenter. The value of r is random and the populations size N is the parameter to be estimated. 15 / 23 Introduction Method of Moments Procedure Mark and Recapture Monsoon Rains Mark and Recapture We can guess the the estimate of N by considering two proportions. the proportion of the tagged fish ≈ the proportion of tagged fish in the second capture in the population r t ≈ k N This can be solved for N to find kt N ≈ : r The advantage of obtaining this as a method of moments estimator is that we evaluate the precision of this estimator by determining, for example, its variance. 16 / 23 Introduction Method of Moments Procedure Mark and Recapture Monsoon Rains Mark and Recapture To begin, let 1 if the i-th individual in the second capture has a tag. X = i 0 if the i-th individual in the second capture does not have a tag. The Xi are Bernoulli random variables with success probability PfXi = 1g = t=N. The number of tagged individuals is X = X1 + X2 + ··· + Xk and the expected number of tagged individuals is t t t kt µ = EX = EX + EX + ··· + EX = + + ··· + = : 1 2 k N N N N The proportion of tagged individuals, X¯ = (X1 + ··· + Xk )=k, has expected value µ t kt EX¯ = = : Thus, N = : k N µ 17 / 23 Introduction Method of Moments Procedure Mark and Recapture Monsoon Rains Mark and Recapture Now in this case, we are estimating µ, the mean number recaptured with r, the actual number recaptured.
Details
-
File Typepdf
-
Upload Time-
-
Content LanguagesEnglish
-
Upload UserAnonymous/Not logged-in
-
File Pages23 Page
-
File Size-