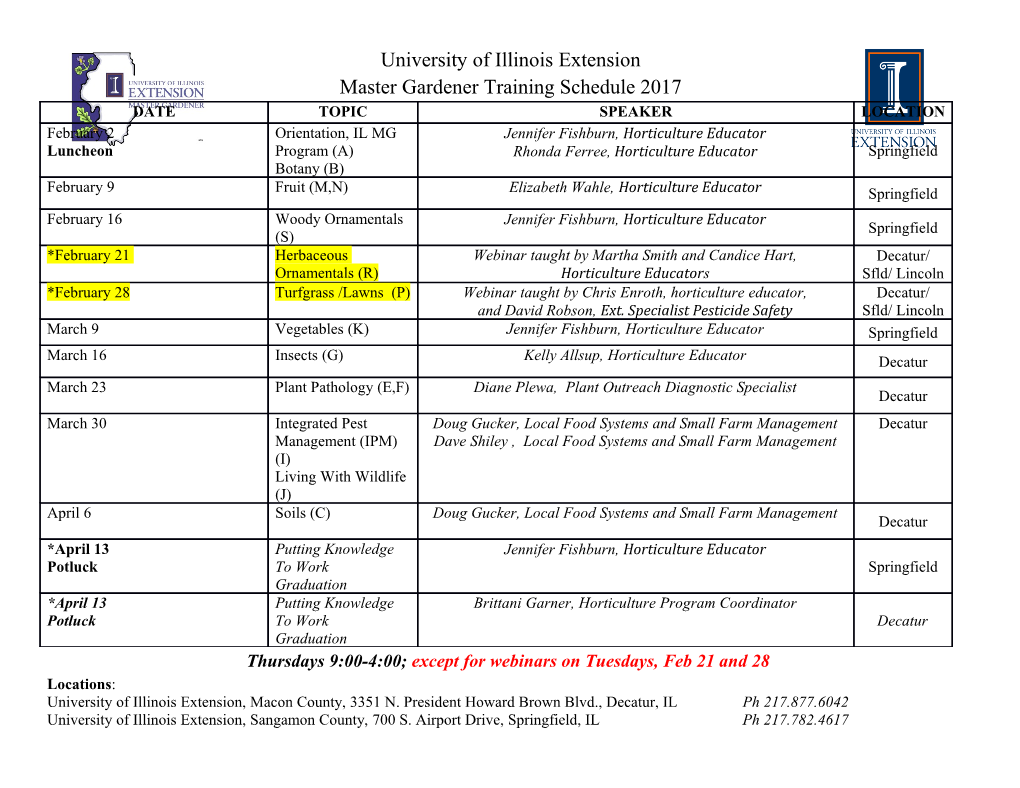
Day 5: Music Recommendation Douglas Eck Research Scientist, Google, Mountain View ([email protected]) 1 Music Music I Like You Like Music I Used To L i ke Get this t-shirt at dieselsweeties.com Thursday, July 7, 2011 Overview • Overview of music recommendation. • Content-based method: autotagging. • Side issues of interest Douglas Eck ([email protected]) CCRMA MIR Workshop Day 5 Thursday, July 7, 2011 Three Approaches to Recommendation • Collaborative filtering (Amazon) “Many people who bought A also bought B. You bought A, you’ll probably like B.” Cannot recommend items no one has bought. Suffers from popularity bias • Social recommendation (Last.FM) Community members tag music. Tag clouds used as basis for similarity measure. Cannot recommend items no one has tagged. Popularity bias (all roads lead to Radiohead) • Expert recommendation (Pandora) Trained experts annotate music based on ~=400 parameters Not scalable (thousands of new songs online daily) Douglas Eck ([email protected]) CCRMA MIR Workshop Day 5 Thursday, July 7, 2011 Music Recommendation Point - Counterpoint: What’s the best way to help users find music they like? Douglas Eck ([email protected]) CCRMA MIR Workshop Day 5 Thursday, July 7, 2011 Point: Use content analysis for music recommendation. • Paul Lamere (EchoNest) • Audio helps us know more about music in the long tail. • Evidence: Examples, observations. Douglas Eck ([email protected]) / Google November 2009 Thursday, July 7, 2011 Help! My iPod thinks I’m emo. SXSW Interactive March 17, 2009 #sxswemo Paul Lamere Anthony Volodkin Photo (CC) by Jason Rogers Thursday, July 7, 2011 Music recommendation is broken A recommendation that no human would make If you like Britney Spears ... You might like the Report on Pre-War Intelligence Thursday, July 7, 2011 Why do we care? Compulsory Long Tail slide Thursday, July 7, 2011 Why do we care? Compulsory Long Tail slide Thursday, July 7, 2011 Why do we care? Compulsory Long Tail slide Thursday, July 7, 2011 Why do we care? Compulsory Long Tail slide Thursday, July 7, 2011 State of music discovery We can’t seem to find the long tail Sales data for 2007 - 4 million unique tracks sold But ... - 1% of tracks account for 80% of sales - 13% of sales are from American Idol or Disney artists State of the Industry 2007 - Nielsen Soundscan Thursday, July 7, 2011 State of music discovery We can’t seem to find the long tail Sales data for 2007 - 4 million unique tracks sold But ... - 1% of tracks account for 80% of sales - 13% of sales are from American Idol or Disney artists State of the Industry 2007 - Nielsen Soundscan Thursday, July 7, 2011 State of music discovery We can’t seem to find the long tail Sales data for 2007 - 4 million unique tracks sold But ... - 1% of tracks account for 80% of sales - 13% of sales are from American Idol or Disney artists State of the Industry 2007 - Nielsen Soundscan Make everything available Thursday, July 7, 2011 State of music discovery We can’t seem to find the long tail Sales data for 2007 - 4 million unique tracks sold But ... - 1% of tracks account for 80% of sales - 13% of sales are from American Idol or Disney artists State of the Industry 2007 - Nielsen Soundscan Make everything available Help me find it Thursday, July 7, 2011 Help! I’m stuck in the head The limited reach of music recommendation Popularity 83 Artists 6,659 Artists 239,798 Artists Sales Rank Study by Dr. Oscar Celma - MTG UPF Thursday, July 7, 2011 Help! I’m stuck in the head The limited reach of music recommendation 48% of recommendations Popularity 83 Artists 6,659 Artists 239,798 Artists Sales Rank Study by Dr. Oscar Celma - MTG UPF Thursday, July 7, 2011 Help! I’m stuck in the head The limited reach of music recommendation 48% of recommendations 52% of recommendations Popularity 83 Artists 6,659 Artists 239,798 Artists Sales Rank Study by Dr. Oscar Celma - MTG UPF Thursday, July 7, 2011 Help! I’m stuck in the head The limited reach of music recommendation 48% of recommendations 0% of recommendations 52% of recommendations Popularity 83 Artists 6,659 Artists 239,798 Artists Sales Rank Study by Dr. Oscar Celma - MTG UPF Thursday, July 7, 2011 Help! My iPod thinks I’m emo Why is music recommendation broken? Thursday, July 7, 2011 The Wisdom of Crowds How does collaborative filtering work? 35% 4% 62% 8% 60% Overlap Data based on listening behavior of 12,000 Last.fm Listeners Thursday, July 7, 2011 The stupidity of solitude The Cold Start problem If you like Blondie, you might like the DeBretts ... But the recommender will never tell you that. Thursday, July 7, 2011 The stupidity of solitude The Cold Start problem If you like Blondie, you might like the DeBretts ... But the recommender will never tell you that. Thursday, July 7, 2011 The stupidity of solitude The Cold Start problem If you like Blondie, you might like the DeBretts ... 0% 0% 0% But the recommender will never tell you that. Thursday, July 7, 2011 The Harry Potter Problem If you like X you might like Harry Potter Thursday, July 7, 2011 The Harry Potter Problem If you like X you might like Harry Potter Thursday, July 7, 2011 Popularity Bias Rich get richer - diversity is the biggest loser Results of popularity bias: - Rich get richer - Loss of diversity - No long tail recommendations Thursday, July 7, 2011 Popularity Bias Rich get richer - diversity is the biggest loser Results of popularity bias: - Rich get richer - Loss of diversity - No long tail recommendations Thursday, July 7, 2011 The Novelty Problem If you like The Beatles you might like ... Thursday, July 7, 2011 The Napoleon Dynamite Problem Some items are not easy to categorize Thursday, July 7, 2011 Help! My iPod thinks I’m emo Fixing music recommendation Thursday, July 7, 2011 Fixing music recommendation Eliminating popularity bias and feedback loops Adam Paul Tim Eric Jim Brian Aaron Peter Chris Liz Yury To d d Bethe Kirk Erik Jason Tristan Sue Jen To d d Cari Scotty Erik Jason Thursday, July 7, 2011 Fixing music recommendation Semantic-based recommendation pop legend dance diva sexy american guilty pleasure 90s teen pop 00s rnb pop rock rock singer-songwriter dance-pop soul emo hot alternative 90s Thursday, July 7, 2011 Fixing music recommendation Where does this information come from? Playlists The web Artist Bios Reviews Blogs Lyrics pop legend dance diva Forums sexy american guilty Events pleasure 90s teen pop 00s rnb pop rock rock singer- songwriter dance-pop soul emo Social sites hot alternative 90s female fronted metal dark rock alternative goth metal metal goth rock emo gothic dark gothic rock heavy metal gothic metal hard rock melodic metal symphonic metal rock metal Crawler pop nu Thursday, July 7, 2011 Fixing music recommendation Content-based recommendation 4 x 10 2 1 0 ! -1 ! -2 0 5 10 15 20 25 sec. BPM 190 143 114 96 72 60 0 5 10 15 20 25 sec. 1 0.8 0.6 0.4 0.2 0 60 72 96 114 143 BPM 190 240 Thursday, July 7, 2011 Content-based recommendation Using machines to listen to music Perceptual features audio: Pattern layer linear ratio - time signature / tempo square ratio - key/ mode Beat layer 1:4 - timbre 1:16 - pitch Segment layer ~1:3.5 - loudness ~1:12.25 - structure ~1:40 Frame layer ~1:1600 1:512 1:262144 Audio layer 4 x 10 2 1 m r o f e 0 v a w ! -1 ! -2 0 0.5 1 1.5 2 2.5 3 sec. 25 m a r 20 g o r t c 15 e p s d 10 n a b - 5 5 2 1 0 0.5 1 1.5 2 2.5 3 sec. 1 0.8 s Thursday, s July0.6 7, 2011 e n d u 0.4 o l 0.2 0 0 0.5 1 1.5 2 2.5 3 sec. 1 n o i t c 0.8 n u f 0.6 n o i t c e 0.4 t e d 0.2 w a r 0 0 0.5 1 1.5 2 2.5 3 sec. n 1 o i t c n 0.8 u f n o 0.6 i t c e t e 0.4 d h t 0.2 o o m s 0 0 0.5 1 1.5 2 2.5 3 sec. Hybrid Recommendation The best of all worlds listener data Hybrid Recommender user history preference editorial 35% 4% 62% 8% 60% 12% 18% 17% 34% 21% recommendations audio data 4% 49% 5% 48% 7% collaborative filterer artist audio analysis track web data content-based random guessing is: adj Term K-L bits np Term K-L bits aggressive 0.0034 reverb 0.0064 softer 0.0030 the noise 0.0051 user a N a synthetic 0.0029 new wave 0.0039 KL = log punk 0.0024 elvis costello 0.0036 N (a + b) (a + c) sleepy 0.0022 the mud 0.0032 b N b funky 0.0020 his guitar 0.0029 + log noisy 0.0020 guitar bass and drums 0.0027 Ncultural(a + b) (b + d) angular 0.0016 instrumentals 0.0021 c N c acoustic 0.0015 melancholy 0.0020 + analysislog N (a + c) (c + d) romantic 0.0014 three chords 0.0019 d N d + log Table 2. Selected top-performing models of adjective and N (b + d) (c + d) noun phrase terms used to predict new reviews of music (3) with their corresemantic-basedsponding bits of information from the K-L distance measure.
Details
-
File Typepdf
-
Upload Time-
-
Content LanguagesEnglish
-
Upload UserAnonymous/Not logged-in
-
File Pages90 Page
-
File Size-