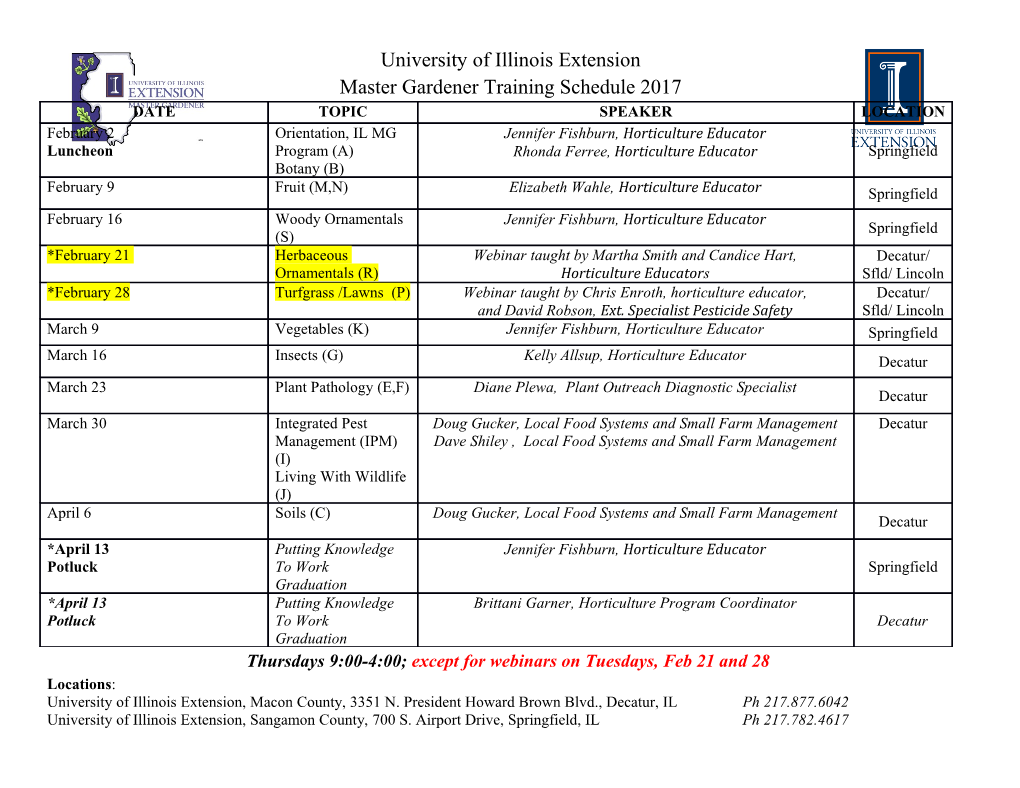
RESEARCH ARTICLE Ensemble PM2.5 Forecasting During the 2018 Camp Fire 10.1029/2020JD032768 Event Using the HYSPLIT Transport Key Points: • The difference between satellite fire and Dispersion Model emission data sets is large, which Y. Li1,2 , D. Q. Tong2,3,4 , F. Ngan4,5 , M. D. Cohen4 , A. F. Stein4 , S. Kondragunta6 , reaches a factor of 10 7 8 9 10 • Ensemble forecast with different X. Zhang , C. Ichoku , E. J. Hyer , and R. A. Kahn emission data sets, meteorology, and 1 2 plume rise schemes can reduce Earth System Science Interdisciplinary Center, University of Maryland, College Park, MD, USA, Department of forecast uncertainty Atmospheric, Oceanic and Earth Sciences, George Mason University, Fairfax, VA, USA, 3Center for Spatial Science and • The new ensemble size reduction Systems, George Mason University, Fairfax, VA, USA, 4NOAA Air Resources Laboratory, College Park, MD, USA, technique can save calculation time 5Cooperative Institute for Satellite Earth System Studies, University of Maryland, College Park, MD, USA, 6NOAA while improving the performance of 7 the ensemble forecast Satellite Meteorology and Climatology Division, College Park, MD, USA, Geospatial Sciences Center of Excellence, Department of Geography and Geospatial Sciences, South Dakota State University, Brookings, SD, USA, 8Department of 9 Supporting Information: Interdisciplinary Studies, College of Arts and Sciences, Howard University, Washington, DC, USA, Marine Meteorology • Supporting Information S1 Division, Naval Research Laboratory, Monterey, CA, USA, 10NASA Goddard Space Flight Center, Greenbelt, MD, USA Correspondence to: Abstract Biomass burning releases a vast amount of aerosols into the atmosphere, often leading to Y. Li and D. Q. Tong, severe air quality and health problems. Prediction of the air quality effects from biomass burning [email protected]; emissions is challenging due to uncertainties in fire emission, plume rise calculation, and other model [email protected] inputs/processes. Ensemble forecasting is increasingly used to represent model uncertainties. In this paper, an ensemble forecast was conducted to predict surface PM2.5 during the 2018 California Camp Fire event Citation: using the National Oceanic and Atmospheric Administration (NOAA) HYSPLIT dispersion model at 0.1° Li, Y., Tong, D. Q., Ngan, F., Cohen, ‐ fi M. D., Stein, A. F., Kondragunta, S., horizontal resolution. Different combinations of four satellite based re emission data sets (FEER, et al. (2020). Ensemble PM2.5 FLAMBE, GBBEPx, and GFAS), two plume rise schemes (Briggs and Sofiev), various meteorology inputs, forecasting during the 2018 Camp Fire and model setup options were used to create the forecast ensemble, for a total of 112 experiments. The event using the HYSPLIT transport and ‐ dispersion model. Journal of performance of each ensemble member and the ensemble mean were evaluated using ground based Geophysical Research: Atmospheres, observations, with four statistical metrics and an overall rank. The ensemble spread of the 112 members 125, e2020JD032768. https://doi.org/ reached 1,000 μg/m3, highlighting the large uncertainty in wildfire forecast. The ensemble mean displayed 10.1029/2020JD032768 the best performance. Each fire emission product contributed to one or more members among the top 10 fi Received 18 MAR 2020 performers, revealing the forecasting dependence on both the quality of re emissions data and model Accepted 20 JUN 2020 representation of emission, transport, and removal processes. In addition, an ensemble size reduction Accepted article online 29 JUN 2020 technique was introduced. With the help of this technique, the ensemble size was reduced from 112 to 28 members and still produced an ensemble mean that yielded comparable or even better performance to that of the full ensemble. 1. Introduction Biomass burning (BB) releases a large quantity of aerosols and trace gases into the atmosphere, often leading to hazardous air quality and health problems (Koning et al., 1985). However, global and regional models do a poor job of predicting the dispersion of aerosol from BB. This is due to uncertainties in fuel type and quality, combustion condition, emission locations and rates, estimation of plume rise, meteorological fields, and atmospheric fate/transport modeling. In addition, the large pollution concentration gradients between affected and unaffected regions contribute to forecast uncertainty. The simulation of the smoke injection height (Hp) plays a vital role in downwind pollutant dispersion, as ©2020. The Authors. wind speed and direction generally change with height. Also, a higher injection height will typically reduce This is an open access article under the near‐field downwind concentrations and can influence removal processes and atmospheric lifetime. terms of the Creative Commons Attribution‐NonCommercial‐NoDerivs Briggs (1969) introduced a set of semiempirical formulas to estimate Hp for stack emissions from stationary License, which permits use and distri- power plant point sources in different atmospheric stability states, using buoyancy flux, horizontal wind bution in any medium, provided the speed, static stability, and atmospheric turbulence conditions. That scheme was improved in the original work is properly cited, the use ‐ is non‐commercial and no modifica- mid 1980s with a set of more sophisticated equations (Briggs, 1984; Weil, 1988). The use of the Briggs tions or adaptations are made. approach for forest fires, an application that it was not designed for, introduces additional uncertainties LI ET AL. 1of19 Journal of Geophysical Research: Atmospheres 10.1029/2020JD032768 that will be discussed in more detail below. Freitas et al. (2007) developed a 1‐D plume rise and transport parameterization for low resolution atmospheric chemistry models. The subgrid plume rise model is based on Latham (1994), which was built upon governing equations for the first law of thermodynamics, vertical motion, and continuity for the water phases. Rio et al. (2010) introduced a pyrothermal plume model by modifying a mass‐flux‐based thermal plume model. In their parameterization, the vertical distribution of the wildfire emission was determined from the burning area, heat released, and the environmental condi- tions. Sofiev et al. (2012) developed a new plume rise scheme, which utilizes fire radiative power (FRP), pla- netary boundary layer (PBL) height, and the Brunt‐Vaisala frequency in the free troposphere to estimate the Hp from wild‐land fires. The parameters of the new scheme were fitted using the plume height observations collected within the Multi‐angle Imaging SpectroRadiometer (MISR) Plume Height Project in North America (Val Martin et al., 2010) and Siberia. Stein et al. (2009) simulated four forest fire cases using the National Oceanic and Atmospheric Administration (NOAA) Air Resources Laboratory's (ARL) HYSPLIT model and found that the ground PM2.5 (particulate matter less than 2.5 μm in diameter) concentration is very sensitive to changes in the smoke release height, especially when the Hp is close to PBL height. Vernon et al. (2018) studied the impact of Hp on downwind dispersion using the HYSPLIT model. Three wildfire cases using the Hp derived from MISR and the Hp estimated by the Briggs (1969) scheme were com- pared. Results showed that when the differences in the injection heights were large, or when the nominal model plume rise and the MISR observations disagree about whether injection was within the PBL, differ- ences in the downwind dispersion would increase. An accurate near‐real‐time BB emission data set with high resolution is also of great importance to BB aero- sol forecasts. With the help of products based on satellite remote sensing active fires and burned areas, var- ious BB emission data sets have been developed. These data sets can be divided into two groups based on the approaches used to generate the data. The first group uses the burned area approach, such as the Fire Locating and Monitoring of Burning Emissions (FLAMBE; Reid et al., 2009), the Fire INventory from NCAR (FINN; Wiedinmyer et al., 2011), and the Global Fire Emissions Database (GFED; van der Werf et al., 2006, 2010, 2017). The other group uses the FRP approach, including the Global Fire Assimilation System (GFAS; Kaiser et al., 2012), the Fire Energetics and Emissions Research algorithm (FEER; Ichoku & Ellison, 2014), the Quick Fire Emissions Dataset (QFED; Darmenov & da Silva, 2013), and the blended Global Biomass Burning Emissions Product from Moderate Resolution Imaging Spectroradiometer (MODIS), Visible Infrared Imaging Radiometer Suite (VIIRS), and geostationary satellites (GBBEPx; Zhang et al., 2019, 2012, 2014). Pan et al. (2020) compared six BB emission data sets for the year 2018, includ- ing GFED Version 3.1, GFED Version 4 with small fires, FINN Version 1.5, GFAS Version 1.2, FEER Version 1.0, and QFED Version 2.4. They found that the BB emissions from these six data sets have similar spatial distributions, but their global total emission amounts differed by a factor of 3–4, which shows great uncer- tainty in BB emissions. The HYSPLIT model is a comprehensive dispersion and transport modeling system for computing trajec- tories, complex transport, dispersion, chemical transformation, and deposition simulations (Draxler & Hess, 1998), which has evolved over more than 30 years. Although an Eulerian mode is available, its Lagrangian framework is most widely used, in which advection and dispersion calculations are based on a plume‐following coordinate
Details
-
File Typepdf
-
Upload Time-
-
Content LanguagesEnglish
-
Upload UserAnonymous/Not logged-in
-
File Pages19 Page
-
File Size-