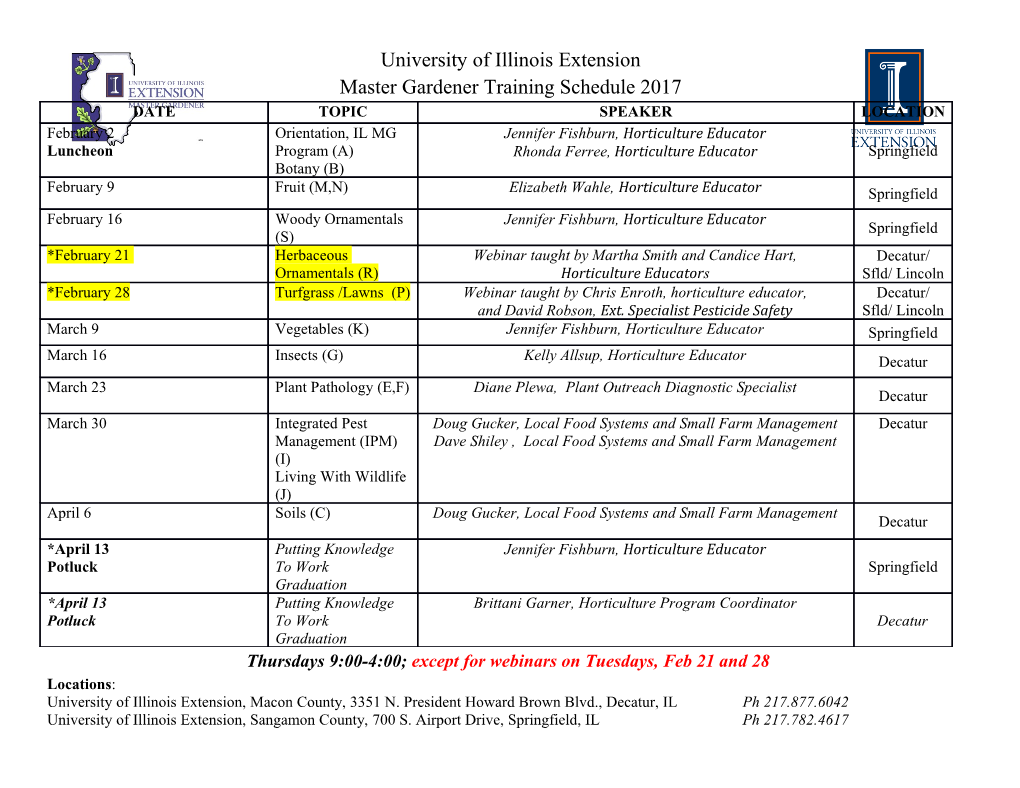
THE GEOMETRY OF SLOPPINESS EMILIE DUFRESNE, HEATHER A HARRINGTON, AND DHRUVA V RAMAN Abstract. The use of mathematical models in the sciences often involves the estimation of unknown parameter values from data. Sloppiness provides information about the uncertainty of this task. In this paper, we develop a pre- cise mathematical foundation for sloppiness as initially introduced and define rigorously key concepts, such as `model manifold', in relation to concepts of structural identifiability. We redefine sloppiness conceptually as a comparison between the premetric on parameter space induced by measurement noise and a reference metric. This opens up the possibility of alternative quantification of sloppiness, beyond the standard use of the Fisher Information Matrix, which assumes that parameter space is equipped with the usual Euclidean metric and the measurement error is infinitesimal. Applications include parametric statistical models, explicit time dependent models, and ordinary differential equation models. 1. Introduction Mathematical models describing physical, biological, and other real-life phe- nomena contain parameters whose values must be estimated from data. Over the past decade, a powerful framework called \sloppiness" has been developed that relies on Information Geometry [1] to study the uncertainty in this proce- dure [10, 17, 56, 57, 58, 55]. Although the idea of using the Fisher Information to quantify uncertainty is not new (see for example [20, 45]), the study of sloppi- ness gives rise to a particular observation about the uncertainty of the procedure and has potential implications beyond parameter estimation. Specifically, sloppi- ness has enabled advances in the field of systems biology, drawing connections to sensitivity [25, 19, 24], experimental design [4, 37, 25], identifiability [47, 55, 13], robustness [17], and reverse engineering [19, 14]. Sethna, Transtrum and co-authors identified sloppiness as a universal property of highly parameterised mathematical models [61, 56, 54, 25]. More recently a non-local version of sloppiness has emerged, called predictive sloppiness [33]. However, the precise interpretation of sloppiness remains a matter of active discussion in the literature [4, 26, 29]. This paper's main contribution is to serve as a first step towards a unified math- ematical framework for sloppiness rooted in algebra and geometry. While our work does not synthesizes the entirety of the field, we provides some of the mathematical elements needed to formalize sloppiness as it was initially introduced. We extend the concept beyond time dependent models, in particular, to statistical models. We rigorously define the concepts and building blocks for the theory of sloppiness. Our approach requires techniques from many fields including algebra, geometry, and statistics. We illustrate each new concept with a simple concrete example. The new mathematical foundation we provide for sloppiness is not limited by current computational tools and opens up the way to further work. Our general setup is a mathematical model M that describes the behavior of a variable x Rm depending on a parameter p P Rr. Our first step is to explain how each precise2 choice of perfect data z induces2 an⊆ equivalence relation on the ∼M;z Date: 3rd Aug, 2018. 1 2 DUFRESNE, HARRINGTON, AND RAMAN parameter space: two parameters are equivalent if they produce the same perfect data. We then characterize the various concepts of structural identifiability in terms 2 DUFRESNE,HARRINGTON,ANDRAMAN of the equivalence relation M;z. Roughly speaking, structural identifiability asks to what extent perfect data∼ determines the value of the parameters. See section 2. theory. We illustrate each new concept with a simple concrete example. The Assume that the perfect data z is a point of N for some N. The second crucial new mathematical foundations we provide forR sloppiness is not limited by current step needed in order to define sloppiness is a map φ from parameter space P to computational tools and opens up the way to further work. data space N giving the perfect data as a function φ(p) of the parameters known Our generalR set up is of a mathematical model M which describes the behavior as a \model manifold" in the literature [56, 57, 58, 55], which we rename as a of a variable x m depending on a parameter p P r.Ourfirststepisto model prediction map.R A model prediction map thus inducesR an injective function explain how each2 precise choice of perfect data z induces2 ✓ an equivalence relation on the set of equivalence classes (the set-theoretic quotient P= ), that is, the M,z) on the parameter space: two parameters are equivalentM;z if they produce equivalence( classes can be separated by N functions P . See∼ Section 3. the⇠ same perfect data. We then characterize the variousR concepts of structural The next step is to assume that the mathematical model! describes the phenome- identifiability in terms of the equivalence relation (M,z). non we are studying perfectly, but that the \real data"⇠ is corruptedN by measurement errorAssume and the we use can of finite identify sample the perfct size. Thatdata z is,with we assume a point that of R noisyfor some data arisesN.The second crucial step needed in order to define sloppiness is a map φ from parameter from a random process whoseN probability distribution then induces a premetric d on thespace parameterP to data space, space via theR Kullback-Leiblergiving the perfect divergence data as a ( function see start of of the Section paramaters 4 ). Thisknown premetric as a “modeld quantifies manifold” the proximity [48, 49, 50, between 47], which the two we parametersredefine as a in model parameter predic- tion map. A model prediction map φ: P RN induces an injective function on space via the discrepancy between the probability! distributions of the noisy data associatedthe set of to equivalence the two parameters. classes, that is, the equivalence classes can be separated by N functions P R. See Section 3. The aforementioned! premetric d has a tractable approximation in the limit of decreasingThe sloppiness measurement of a noise model using manifests the Fisher itself Information when dealing Matrix with (FIM).noisy data. In the We standardassume definition, that the mathematical a model is \sloppy" model when describes the condition the phenomenon number we of the are FIM studying is large,perfectly, that is, but there that are the several “real data” orders is of corrupted magnitude by between measurement its largest error and and/or smallest the use eigenvalues.of finite sample Multiscale size. Therefore sloppiness noisy (see [44]) data extends arises from this a concept random to process regimes whose of non- prob- infinitesimalability distribution noise. induces a premetric on the parameter space, via the Kullback- LeiblerWe conceptually divergence. extend This premetric the notion quantifies of sloppiness the proximity to a comparison between between parameters the in premetricparameterd and space a reference via the discrepancy metric on parameter between the space. probability We demonstrate distributions that of us- the noisy data associated to distinct parameters. ing the condition number of the FIM to measure sloppiness at a parameter p0, as is doneThe in aforementioned most of the sloppiness premetric literature has a tractable [10, 17, 56,approximation 57, 58, 55], in corresponds the limit of decreasing noise using the Fisher Information Matrix (FIM). In the standard defi- to comparing an approximation of d in an infinitesimal neighborhood of p0 to the nition, a model is “sloppy” when the condition number of the FIM is large, that is, standard Euclidean metric on Rr P . Note that considering the entire spectrum of thethere FIM, are as severalis done ordersnewer work of magnitude in⊃ the sloppiness between literature its largest (eg, and [61]) smallest corresponds eigenvalues. to performingWe conceptually a more refined extend comparisonthe notion of between sloppiness an approximationto a comparison of betweend in an thisinfini- pre- metric and a reference metric on parameter space. We demonstrater that using the tesimal neighborhood of p0 to the standard Euclidean metric on R P . Multiscale sloppiness,condition which number we of extend the FIM here to beyond measure its sloppiness original definition at a parameter [44]⊃ forp0 Euclidean, as is done parameterin mostspace of the and sloppiness Gaussian literature, measurement corresponds noise, avoids to comparing approximating an approximationd, and so of d in an infinitesimal neighborhood of p0 to the standard Euclidean metric on betterr reflects the sloppiness of models beyond| the infinitesimal scale. Finally, we describeR sup theP . intimateWe alsoput relationship into context between and generalise sloppiness the and quantification practical identifiability, of sloppiness thatintroduced is, whether in [36] generic which noisy avoids data approximating allows for boundedd and confidenceso better reflects regions the when slop- performingpiness of maximum models beyond likelihood the infinitesimal estimation. Seescale. Section Finally, 4. we describe the intimate relationshipThe following between diagram sloppiness illustrates and the practical main objects identifiability. discussed See in this Section paper: 4. The following diagram illustrates the main objects discussed in this paper: M a mathematical model P/ M,z x variable, belongs to X Rm ⇠ ✓ inj y observable, belongs to Y Rn ✓ φ model prediction map r φ N r R P Z R p parameter, belongs to P R ⊃ ⇢ ✓ z data, belongs to Z RN ✓ g g function giving y in terms of x Rm X Y Rn ⊃ ⇢ 2. Arelationonparameterspace A mathematical model M describes the behavior of a variable x X Rm 2 ✓ depending on a parameter p P Rr, with measurable output y = g(x) Y Rn. We further specify a choice2 of perfect⇢ data z produced for the parameter2 2 value p. We think of the perfect data z as belonging to the wider data space Z which THE GEOMETRY OF SLOPPINESS 3 2. An equivalence relation on parameter space A mathematical model M describes the behavior of a variable x X Rm 2 ⊆ depending on a parameter p P Rr, with measurable output y = g(x) Y Rn.
Details
-
File Typepdf
-
Upload Time-
-
Content LanguagesEnglish
-
Upload UserAnonymous/Not logged-in
-
File Pages32 Page
-
File Size-