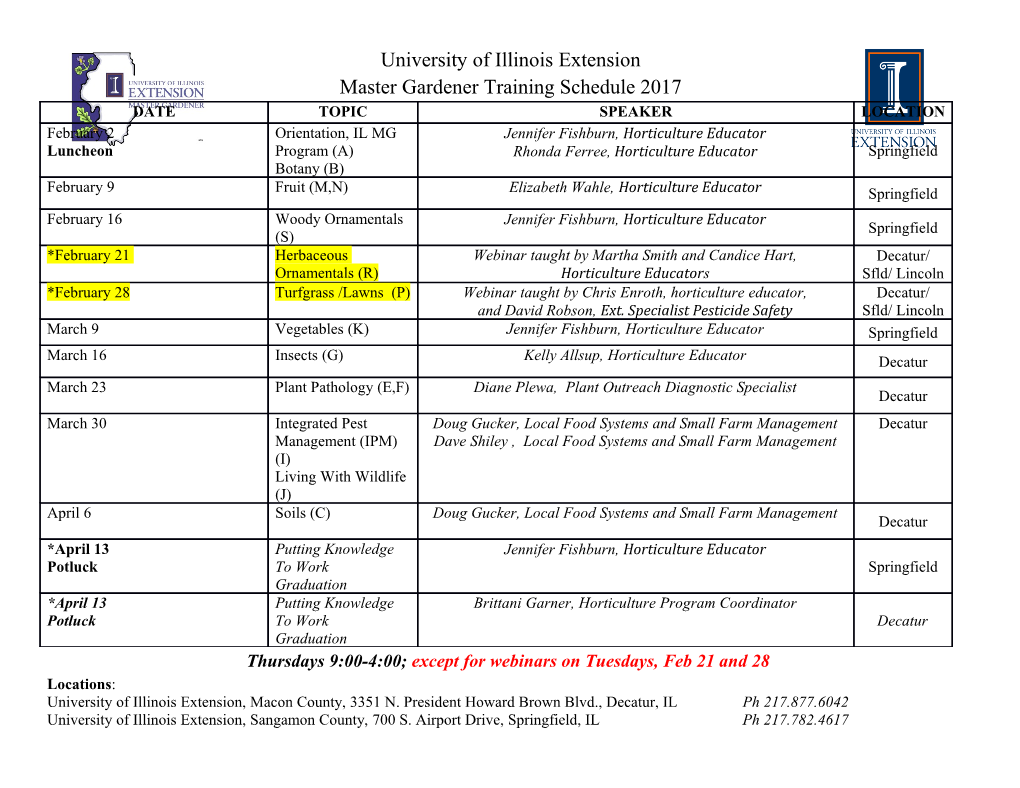
MEASURES OF ASSOCIATION FOR MULTIVARIATE CONTINGENCY TABLES James M. Sakoda, Brown University Need for a Measure of Association Somer's D and Goodman and Kruskal's gamma are too specialized for routine contingency table anal- In analyzing multidimensional contingency ysis, since they apply only to certain tables. tables the goodness of fit of various models is Moreover, gamma and its 2 x 2 table version, generally tested via Pearson or likelihood ratio Yule's have tendencies to be high in compari- chi.square. The acceptance or rejection of a son to other coefficients when marginals are model on the basis of a significance test alone distributed unevenly. runs the risk of allowing the number of cases to determine, at least in part, the number of param- Another advantage of -based measures of eters deemed to be significant. As in other test association is their symmetric nature, requiring situations, judgment of the existence of a rela- only a single measure regardless of the direction tionship should be dependent on the strength of of relationship or prediction. There are a num- the relationship as well as its statistical sig- ber of asymmetric measures of association which nificance. If a sizable relationship is indi- are developed on different bases and which are cated the acceptable significance level might be meaningful in different ways. These are Goodman raised to .10, say, to avoid rejecting a poten- and Kruskal's proportional reduction of errors of tially meaningful source of variation. Converse- prediction measures lambda and tau, Margolin and ly, effects which are extremely small, even Light's analysis of variance measure of though statistically significant, might be elim- proportion of row variation attributable to col- inated from a model. Measures of association are umn variation, and the proportional reduction of also useful in comparing tables with different uncertainty measure based on information theory. numbers of cases. Lambda cannot be recommended for tables with un- even marginals since a zero coefficient results Several measures of association for contin- when the largest frequencies in each column fall gency tables have been developed for two -way in one row and other measures of association show tables, and one of the problems is to select an a relationship. This fault is not shared by tau, appropriate one fort use with higher dimensioEs. even though it is also a measure developed on the We conclude that X divided by the maximum X for principle of proportional reduction in errors of a table serves as a suitable basis for a measure prediction. On the other hand, tau is numeri- of association. A second problem is the applica- cally identical to Margolin and Light's (1974) tion of the chosen measure of association to high- BSS/TSS measure, showing that proportional reduc- er dimensioned tables. In some situations multi- tion in error can be quite similar to proportion dimensional tables can be related to two -way of explained variation. According to Bishop, tables. In the multiple correlation type of situ- Fienberg and Hollan (1975: 391), BSS/PSS and tau ation a dependent variable can be related to a involve a Pearson X -like expression, and when combination of categories of independent vari- tEe row sums are equal, tgey are equal to ables by using a two -way table. In the partial 0 / (I -14and hence to X / N(I -1). N(I -1) is correlation situation, two -way tables can be av- maximum X when I e J. The relative reduction it eraged over a set of control variables. For uncertainty measure utilizes likelihood statis- higher order effects, such as a three-way or four- tics to express uncertainty, which is "variance - way effect or for combinations of effects, reduc- like" (Hays, 1973). These measures, except for tion to two -way tables is not possible. The task their asymmetric nature (some have symmetric is then to find the maximum X for higher order versions), have a great deal in common bop mean- the effects that they can be compared with ob- ingfully and2numerically, with Craér's V , which tained X for a given effect. Goodman (1971) represents X divided by maximum X . The inter- suggested using a proportional reduction in X2 as pretation of these measures not any easier a method of calculating multiple or partial cor- than the interpretation of X -based measures, as relation coefficients. The approach suggested is sometimes claimed. In fact, these measures here differs from his in that higher order effects produce very small coefficients generally in com- are analyzed in terms of the maximum X father parison with measures such as V or the contin- than an arbitrarily- selected empirical X . gency coefficient which resemble the Pearson r rather than r as these measures do. The Choice Among Measures of Association One of the oldest X2 -based measures of For analysis of multidimensional tables the association is Karl Pearson's mean square con- most convegient measures of association are those tingency or contingency coefficient: based on X', since data analysis is performed us- ing X2. It is possible to partition higher order X into their component parts and to relate X C = - for two -way tables to higher order ones. Goodman + , (1971), for examp ;e, suggests using a proportion- 02 where is estimated by X2 / N. "Karl Pearson al reduction in X as a method of calculating showed that, if the itemsare capable of inter- multiple and partial correlation coefficients. pretation as a quantitatively ordered series, if X is suitable with either ordered or unordered the distributions are normal, and if the regres- categories. Measures of association requiring sion is rectilinear, C becomes identical with r ordered categories, such as Kendall's tau, as the number of categories is indefinitely in- 777 creased." (Peters and vanVoorhis, 1940: 392). x 2 In other words, C is an estimate of -the Pearson X2.=N . but can be applied even when categories are the relationships are not linear. unordered and With cancellation of x Its shortcoming is that its maximum value does not reach unity. But maximum ¢2 is the minimum I -1 or J -1 and C can be calculated: of -max N (JJ - 1) X2max x / m.in(I-1,J-1) i+ rin(I,J) By definition xi+ . .Therefore, It is possible to correct C to achieve unity by N (E1-1) = N (I-1) , calculating C/C , although it is not a standard max practice. prow proposed the use of or when J I , X2 = N (J-1) T= - max - 1/(I-1)(J-1) Hence, but maximum of 1 only for square N min(I-1,J-1) achieved a R2max tables. Its use is therefore not recommended. For example, Cramer (1946) suggested norming 02 by divid- ing it by its maximum value: X=2 60(3030302 152 52 102 + 2015 + 2Ó55 1010 1) 2 = 60( 1 + 1 + 1 -1) V min(I-1,J-1) 60(3-1) 120 . Estimating 02 Analogue of Multiple Correlation X2 The analogue to the multiple correlation, in which Variables 2, 3 and 4, for example, are re- min(I- 1,J -1) lated to Variable 1, the dependent variable, can be set up by means of a two -way table. The row The denominator term is maximum X2 for a two -way variable is Variable 1 with I categories and the I x J table so that V can be given a proportion column variable consists of all possible combina- of maximum variation due to interaction interpre- tions of categories of Variables 2, 3 and 4 with tation. V has an acceptable interpretation via J x K x L categories in all. In terms of the V2 and unlike C and T varies between 0 and 1. It loglinear model this table represents an independ- is our choice as a suitable measure for applica- ence model (1 x 234), which tests all effects of tion to higher order tables, with C/C a second 2, 3 and 4 on 1: (12), (13), (14), (123), (124), possibility. (134), (1234). The model can be run on a program like ECTA by fitting 1 and 234. With X2 for the Maximum X2 table available it is a simple matter Tin compute Cramer's V and it can be treated as an analogue According to Cramer (1946: 443), the maximum to the multiple correlation coefficient: V2 of unity is obtained "when and only when each row (when r s) or each column (when r s) con- X2(1x234) tains one single element different from zero." An example of arrangement of cell frequencies !1.234 N min(I-1,JKL-1) for a maximum V is shown in Fig. 1 for a 3 x 4 table with I J. Cramér's condition can be ex- In Fig. 2 is shown a 3 x 2 x 4 table ar- pressed as ranged to give a maximum X2 for Variable 1 xii . against 2 and 3 combined. In fill,jng the cells the distinctions between categories of Variable 2 30 - - - 30 and 3 are ignored. Maximum X2 is equal to - 15 5 - 20 N x (3 -1) or 240. - - - 10 10 10 - - 20 - - 10 - 40 30 15 5 10 60 - 20 - - 10 - - 20 50 - - 10 - - 20 - - 30 Fig. 1. A 3 x 4 Table with Cramér's V = 1.0. 10 20 10 20 10 20 10 20 120 Fig. 2 A3 2 x 4 Table Ariranged for Maximum R2(1x23). We start with the formula for X2: 778 Partial Correlation Fitted Model Parameters X2 df p V C/C Partial correlation is generally defined as - - - -max a measure of association between two variables (1x2134) (134)(234) 27.81 6 .000 .166 .232 holding constant the effects of a third variable.
Details
-
File Typepdf
-
Upload Time-
-
Content LanguagesEnglish
-
Upload UserAnonymous/Not logged-in
-
File Pages4 Page
-
File Size-