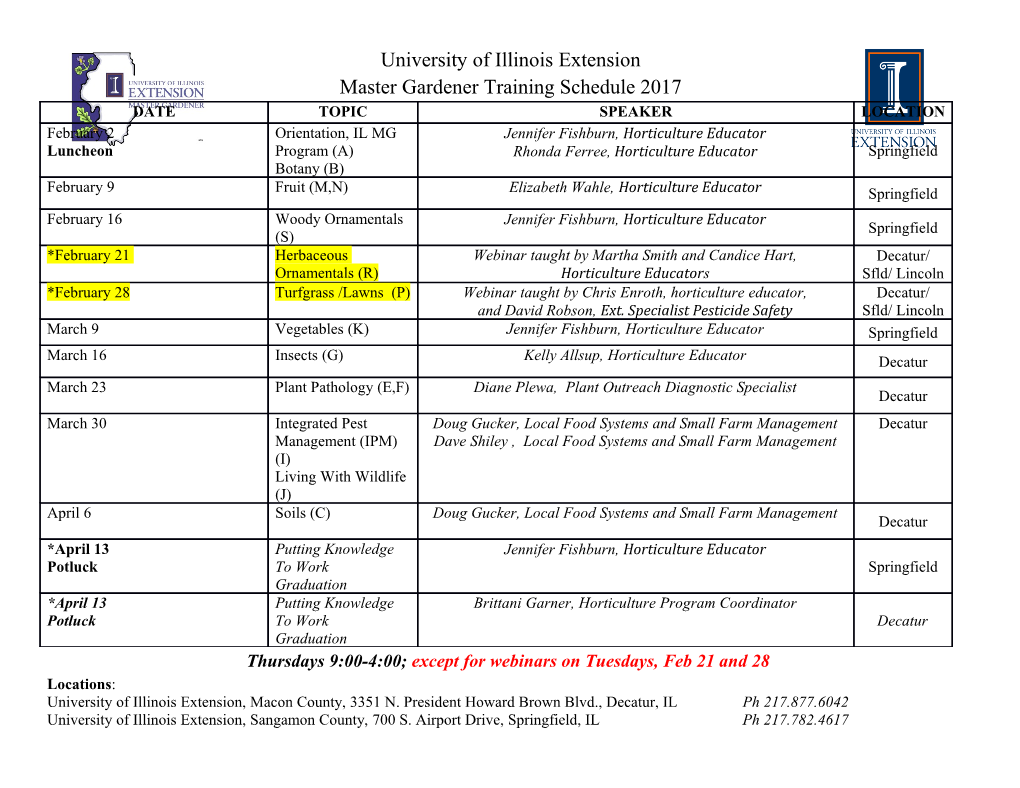
Volume 21, Number 1, February/March 2015 Adjusting the Sample Size during the Experiment Aniko Szabo, PhD, Division of Biostatistics, MCW Selecting the sample size for a study is an essential part of research. The basic statistical tenet of sample size selection is that it needs to be selected in advance, and modifying it based on the collected data can lead to invalid results. The IRB and the IACUC require sample size justification before approval of human and animal studies, respectively, so correct practices are typically followed. However in contexts not governed by these agencies in detail, such basic science laboratory studies or secondary data analyses of existing records, practices vary widely. In this article we will illustrate some of the dangers of incorrect practices, and discuss group- sequential designs as a possible solution. Sampling to significance A common experimental plan that researchers apply – consciously or not – is to collect some data, stop if the results are statistically significant, or collect more data to increase the power if not. Statisticians refer to this practice as “sampling to significance.” In fact, if such a procedure would be carried on indefinitely long, all experiments would give a statistically significant result sooner or later! Figure 1 shows simulated results for 10 hypothetical series of experiments in which there is no actual difference between two study groups. The p-value is calculated after each set of 5 additional samples from both groups, up to 50 samples per group. In all the 10 © 2015 by Division of Biostatistics, Medical College of Wisconsin Volume 21, Number 1, February/March 2015 experiments the p-value varies substantially between repeated tests, and in three experiments dips below the significance level of 0.05 denoted by the blue horizontal dashed line. The red points show where the experimenter would have stopped the study, the future p-values shown by gray lines would never be observed. In two of the cases the p-values would have “rebounded” if the experiment were continued, and only one of the runs would have been significant at the final sample size. These simulations demonstrate the increased rate of false positives when the researcher “looks” at the p-value multiple times. While false positives are unavoidable, a significance level of 0.05 should limit their occurrence to 5% of experiments. In the situation described above, the probability of a significant result at one of the 10 multiple possible looks is almost 20%! While this example is somewhat extreme – most researchers would probably add new samples only once or twice – the general phenomenon of increasing rate of false positives is still present. Even just one additional look after 25 samples per group almost doubles the false positive rate. Sequential testing The sample size required for any given study depends on a number of factors, such as the expected variability of the measurements or the expected difference between the groups. Unfortunately, these are often unknown at the initiation of a study. One of the reasons for the researchers’ reluctance to commit to a fixed sample size a priori might be the uncertainty about © 2015 by Division of Biostatistics, Medical College of Wisconsin Volume 21, Number 1, February/March 2015 these inputs. What if the variability is larger than expected? The preplanned sample size might be too low. What if the difference is much larger than expected? Perhaps money and effort could be saved by stopping earlier. The repeated looks seem to offer a solution to these problems, by allowing the sample size to adjust to the experiment, but as we saw above, this approach is not statistically valid. Fortunately, several statistical methods allowing such multiple looks have been developed. So called group-sequential designs are most commonly used in clinical trials, but they are applicable in any situation where sample size adjustment is desired. A group-sequential design requires specifying the maximum sample size, and the number and timing of the intermediate evaluations. A statistical program then can calculate the significance cutoff for each look. Figure 2 shows two such possible adjusted cutoffs for the previous simulation study. The vertical axis is restricted to the 0 to 0.06 range to show the details, so only the three simulation runs with p- values under 0.05 are shown. The green dotted line shows the so-called Pocock boundary, which uses the same adjusted significance level, here 0.0116, for each comparison. Simulation 4 crosses below this boundary at 45 samples per group, simulation 5 at 15, but simulation 9 never reaches it. So in our small example 2 out of the 10 simulations crossed even the adjusted boundary, but it can be proven that in the long run only 5% will do so. In this example we planned for up to 10 equal-spaced looks at the p-value; Table 1 shows the p-value cutoffs for different number of evaluations. Number of 2 3 4 5 6 7 8 9 10 looks Significance 0.0304 0.0232 0.0193 0.0169 0.0152 0.0140 0.0130 0.0122 0.0116 cutoff One drawback of the constant Pocock boundary is that if the study goes to the planned maximal size, the significance level that has to be used is much lower than the typical 0.05 level that would have been used without the interim looks. As an alternative, a different boundary with significance cutoffs that increase with sample size can be used. The red solid line shows © 2015 by Division of Biostatistics, Medical College of Wisconsin Volume 21, Number 1, February/March 2015 such a boundary; initially a very low p-value is needed to stop the trial, so simulation 5 is not stopped after 15 samples (though it gets really close to the cutoff). The benefit is that by the end of the study the significance cutoff is much closer to 0.05, it is 0.0412 in this example. As with the Pocock boundary, even though 1 out of the 10 simulations crossed this boundary, in the long run only 5% will do so. 4 5 9 0.06 0.05 0.04 P-value 0.03 0.02 0.01 0.00 10 20 30 40 50 10 20 30 40 50 10 20 30 40 50 Sample size Significance boundary Adjusted-varying Adjusted-constant Unadjusted Conclusion Adjusting the sample size of the study based on accumulating data destroys the statistical validity of p-value testing. However more complicated study designs, such as group-sequential studies or internal pilot studies (not discussed here) can accommodate situations with large uncertainty in estimates required for sample size calculations. Any such designs need to be planned in advance, and adjustments need to be made even if the study is not stopping early. © 2015 by Division of Biostatistics, Medical College of Wisconsin .
Details
-
File Typepdf
-
Upload Time-
-
Content LanguagesEnglish
-
Upload UserAnonymous/Not logged-in
-
File Pages4 Page
-
File Size-