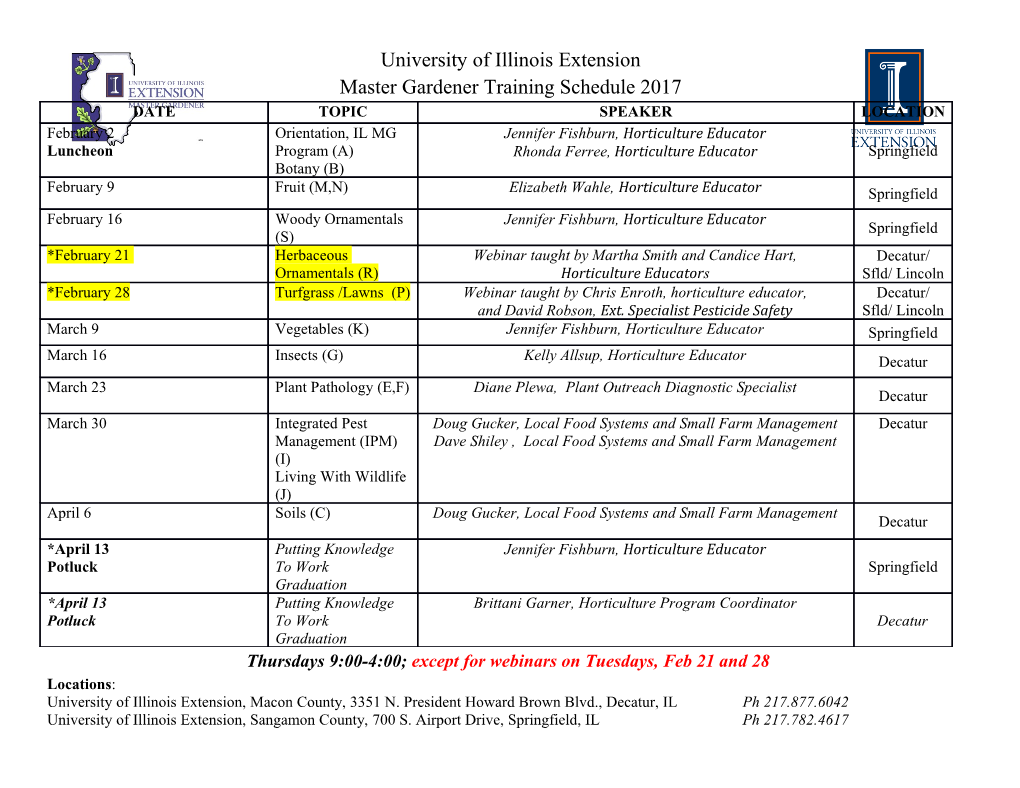
Natural Intersection Cuts for Mixed-Integer Linear Programs Trivikram Dokka∗ Adam N. Letchford∗ Draft, April 2014 Abstract Intersection cuts are a family of cutting planes for pure and mixed- integer linear programs, developed in the 1970s. Most papers on them consider only cuts that come from so-called maximal lattice-point-free polyhedra. We define a completely different family of intersection cuts, called \natural". Their key property is that they can be generated very quickly and easily from a simplex tableau. In many cases, one can also easily strengthen them, using an idea of Balas and Jeroslow. We show that the strengthened cuts are a generalisation of Gomory mixed-integer cuts, and then show how to tailor the cuts to problems with special structure (such as knapsack, packing, covering and par- titioning problems, or problems with complementarity constraints or fixed charges). Our computational results are encouraging. Keywords: Integer programming, cutting planes, branch-and-cut, set packing, set partitioning, set covering. 1 Introduction Cutting planes, or more briefly, cuts, are a key ingredient of many modern integer programming algorithms (see, e.g., [14, 15, 27]). Broadly speaking, cutting planes fall into two classes. First, there are \specialised" cutting planes, that come from a study of a particular family of mixed-integer linear programs (MILPs). Such cuts are usually shown to define facets of the integral hull, or at least faces of high dimension (see, e.g., [15, 27]). Second, there are \general-purpose" cutting planes, that can be defined for any MILP (see, e.g., [14, 17, 27]). Examples of such cuts are Gomory mixed-integer (GMI) cuts [25], intersection cuts [2], disjunctive cuts [4], split cuts [16] and mixed-integer rounding (MIR) cuts [28]. This paper focuses on intersection cuts. After their introduction in 1971 by Balas [2], there was a brief flurry of activity on them (e.g., [3, 5, 12, 21, ∗Department of Management Science, Lancaster University, Lancaster LA1 4YX, United Kingdom. E-mail: fT.Dokka,[email protected] 1 22, 23, 24]). After that, research on them died down for a while. Recently, however, they have received a resurgence of interest (e.g., [1, 10, 11, 18, 19]. This is for two main reasons: • In theory, every GMI, split or MIR cut can be deduced from a single row of a simplex tableau, given a suitable (possibly primal and/or dual infeasible) basis [9]. This is not true for intersection cuts [1]. So, potentially, intersection cuts can capture more \global" aspects of the problem. • Although intersection cuts can be viewed as a subclass of the disjunc- tive cuts [4, 9, 10], they can usually be generated fairly quickly and easily from a simplex tableau (e.g., [2, 5, 23]). By contrast, to generate disjunctive cuts, one must solve large LPs [4, 6], or at least perform a sequence of simplex pivots [9]. To generate strong intersection cuts, one needs first to generate \large" polyhedra that have no feasible solutions in their interior. Most papers on intersection cuts focus on so-called maximal lattice-point-free polyhedra, which are maximal polyhedra that do not contain any integral points in their interior [26]. The only exception of which we are aware is Balas [3], which uses so-called outer polars. In this paper, we focus on a completely different family of \large" polyhedra, which we call \natural". Like outer polars, these polyhedra are permitted to contain lattice points in their interior, but only infeasible ones. The difference is that each facet of a natural polyhedron is parallel to a facet of the feasible region of the continuous relaxation of the problem. As we will show, this makes the corresponding intersection cuts, also called \natural", particularly easy to generate. The paper is structured as follows. In Section 2, we review the relevant literature. In Section 3, we define natural polyhedra and the associated cuts. We also define \strengthened" natural intersection cuts, and show that they include all GMI cuts. In Section 4, we show to how to construct useful natural polyhedra, both for general MILPs and for MILPs with binary variables (including knapsack, packing, covering and partitioning problems, and problems with complementarity constraints and fixed charges). We also show how to generate and strengthen the resulting intersection cuts. In Section 5, we describe a rudimentary cutting-plane algorithm based on our cuts, and give extensive computational results, on all of the problem classes mentioned. Finally, concluding remarks are made in Section 6. 1.1 Notation and terminology Throughout the paper, we use the following notation and terminology. We assume that we are given a MILP with n variables and m constraints, of the form: T min c x : Ax ≤ b; x ≥ 0; xi 2 Z (i 2 I) ; 2 m×n m n where A 2 Z , b 2 Z , c 2 Q and I ⊆ f1; : : : ; ng. The variables in I are called integer-constrained. The feasible region of the continuous relaxation of the MILP is the poly- n hedron P = fx 2 R+ : Ax ≤ bg. The convex hull of the feasible MILP solutions is the polyhedron PI = conv fx 2 P : xi 2 Z (i 2 I)g : A cutting plane is an inequality that is valid for PI , but not for P . The modified MILP that results when one adds non-negative slack vari- ables xn+1; : : : ; xn+m and converts the inequalities Ax ≤ b into the equations (AjI)x = b is called the augmented MILP. To avoid confusion, we use x for the variables in the original MILP andx ~ for the variables in the augmented MILP. We use I~ to denote the union of I and the set of all slack variables that have to take integral values (because the associated inequalities have zero coefficients for all continuous variables). The polyhedra associated with the augmented MILP will be denoted by P~ and P~I , respectively. Note that P~ and P~I are affine images of P and PI , respectively. A cutting plane for the augmented MILP is an inequality that is valid for P~I , but not for P~. It becomes a cutting plane for the original MILP when slack variables are eliminated. ∗ n+m Letx ~ 2 Q+ be a basic feasible solution to the LP relaxation of the augmented MILP, and note thatx ~∗ is an extreme point of P~. We partition the index set f1; : : : ; n + mg into a set B of basic variables and a set N of non-basic variables (with jBj = m and jNj = n). The simplex tableau then provides us with a system of m simultaneous linear equations, which we write in the form X ∗ x~k + αikx~i =x ~k (k 2 B): (1) i2N We also sometimes write this system in the compact form ~ ∗ x~B + Ax~N =x ~B: (2) Finally, a set M of vectors is called a monoid if the zero vector is in M, and t1; t2 2 M implies t1 + t2 2 M. 2 Literature Review Now, we review the relevant literature. We recall GMI cuts in Subsection 2.1, intersection cuts in Subsection 2.2, and disjunctive and split cuts in Subsection 2.3. Subsection 2.4 reviews the relationship between intersection cuts and disjunctive cuts. 3 2.1 Gomory mixed-integer cuts ∗ n+m ∗ Letx ~ 2 Q+ be defined as above, and suppose thatx ~k 2= Z for some k 2 B \ I~. For i 2 N, let fi denote αik − bαikc, the so-called fractional part ∗ ∗ of αik. Also let f0 denotex ~k − bx~kc. Then, Gomory [25] showed that the following cutting plane is violated byx ~∗: X αik −αik X fi 1 − fi max ; x~i + min ; x~i ≥ 1: (3) f0 1 − f0 f0 1 − f0 i2NnI~ i2N\I~ This is the Gomory mixed-integer (GMI) cut. In [7], very good computational results were obtained by generating ~ ∗ rounds of GMI cuts, i.e., one cut for every k 2 B \ I such thatx ~k 2= Z, within a branch-and-cut framework. For more recent computational results with GMI cuts, see, e.g., [20]. 2.2 Intersection cuts Intersection cuts, sometimes called convexity cuts, are defined as follows [2, 3, 21]. Let x∗ be an extreme point of P that is not feasible for the MILP. In the absence of degeneracy, x∗ will lie on n edges of P . Each edge defines a direction in which one can move, to go from x∗ to an adjacent extreme n point of P . Now, let S ⊂ R be any closed, bounded and full-dimensional convex set such that: • x∗ lies in the interior of S, and • no feasible MILP solution lies in the interior of S. If we start at x∗ and move in any of the n directions mentioned above, we will eventually reach the boundary of S. The point where this occurs is called an intersection point. Then, the hyperplane that passes through the n intersection points defines a cutting plane that is both valid for PI and violated by x∗. This is the intersection cut. (If there is degeneracy, then one can temporarily drop all but n of the constraints that are tight at x∗, to obtain the desired n directions. The resulting cut remains valid.) If S is a polyhedron, then there is a closed-form expression for the in- tersection cuts, in the space of the augmented MILP. Suppose that S is defined by s linear inequalities. Use the linear system (2) to write those s inequalities in the form: X γirx~i ≥ 1 (r = 1; : : : ; s): i2N Then the intersection cut can be written as: X max fγirg x~i ≥ 1: (4) 1≤r≤s i2N 4 One can define intersection cuts also when S is not bounded.
Details
-
File Typepdf
-
Upload Time-
-
Content LanguagesEnglish
-
Upload UserAnonymous/Not logged-in
-
File Pages18 Page
-
File Size-