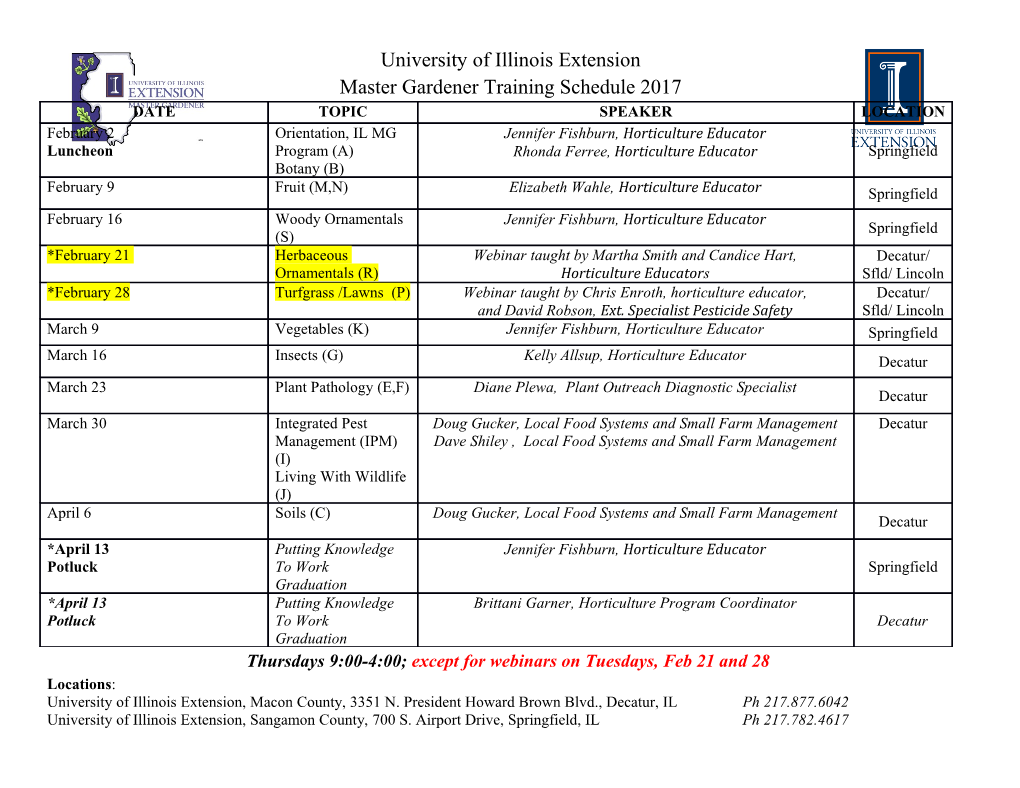
International Journal of Modern Mathematical Sciences, 2013, 8(3): 149-153 International Journal of Modern Mathematical Sciences ISSN:2166-286X Journal homepage:www.ModernScientificPress.com/Journals/ijmms.aspx Florida, USA Article A Class of Modified Ratio Estimator Using Co-efficient of Kurtosis and Quartile Deviation M. Iqbal Jeelani*, S. Maqbool, S. A. Mir, Imran Khan, Nageena Nazir and Fehim Jeelani Division of Agricultural Statistics SKUAST-K, India * Author to whom correspondence should be addressed; E-Mail: [email protected] Article history: Received 29 March 2013, Received in revised form 8 November, Accepted 25 November 2013, Published 3 December 2013. Abstract: In this article, we suggest a new modified ratio estimator of population mean of the study variable using the linear combination of known values of Co-Efficient of Kurtosis and Quartile deviation of the auxiliary variable. Mean square error and bias of the proposed estimator is calculated and compared with Yan & Tian [5] estimator. By this comparison, we demonstrate theoretically and numerically that the proposed estimator is more efficient than the existing one in all conditions. Keywords :Ratio type estimator, Simple random sampling, auxiliary information, Mean square error, Bias, Co-Efficient of Kurtosis, Quartile deviation. Mathematics Subject Classification (2010): 62D05 1. Introduction As Deming [1] has rightly said “Sampling is not mere substitution of a partial coverage for a total coverage. Sampling is the science and art of controlling and measuring the reliability of useful statistical information through the theory of probability”. The simplest and the most common method of sampling is simple random sampling in which a sample is drawn unit by unit, with equal probability of selection for each unit at each draw, where there is no additional information available. In sample surveys, along with study variable 푌, information on auxiliary variable 푋, which is correlated with 푌 is Copyright © 2013 by Modern Scientific Press Company, Florida, USA Int. J. Modern Math. Sci. 2013, 8(3): 149-153 150 also collected. This information on auxiliary variable 푋, may be utilized to obtain a more efficient estimator of the population mean. Ratio method of estimation using auxiliary information is an attempt in this direction. For ratio estimators in sampling theory, population information of the auxiliary variable, such as Co-efficient of variation, Population Co-Efficient of Correlation Co-efficient of Skewness, is often used to increase the efficiency of the estimation for a population mean. However, no attempt is made to use the linear combination of known values of population values of Co-Efficient of Kurtosis and Quartile deviation of the auxiliary variable to improve the ratio estimators. Further, we know that the values of above linear combinations are unaffected by the extremes values or presence of outliers in the population values. These points have motivated the authors the modified ratio estimators using the above linear combinations. The classical ratio estimator for population mean 푌̅ is defined as: 푦̅ 푌̅̂ = 푋̅ = 푅̂푋̅ , (1) 푅 푥̅ 푦̅ 푦 푌̅ 푌 where 푅̂ = = is the estimate of 푅 = = and it is assumed that the population mean 푋̅ of the 푥̅ 푥 푋̅ 푋 auxiliary variate 푋 is known [2]. Hence 푦̅ is the sample mean of the variate of the interest and 푥̅ is the ̂ sample mean of the auxiliary variate. The bias and the mean square error of 푌̅푅 to the first degree of approximation is given below: 1−푓 퐵푖푎푠(푦̅̂ ≅ 푌̅(퐶2 − 2퐶 퐶 휌) (2) 푅) 푛 푥 푥 푦 1−푓 푀푆퐸(푦̅̂ ≅ 푌̅2(퐶2 + 퐶2 − 2퐶 퐶 휌) (3) 푅) 푛 푦 푥 푥 푦 where, 퐶푥, 퐶푦 are the co-efficient of variation and 휌 is the co-efficient of correlation [3]. The ratio estimator given in (1) is more price than the simple random sample mean, when there is positive correlation between 푋 and 푌. The historical developments of ratio method of estimation from 1662 ha been very well defined by Sen [4]. 2. Yan and Tian [5] Estimator Yan and Tian [5] have proposed two modified ratio estimators using the linear combinations of Co-Efficient of skewness and Co-Efficient of kurtosis. ̂ 푋̅훽2+훽1 푌̅1 = 푦̅ [ ] (4) 푥̅훽2+훽1 ̂ 푋̅훽1+훽2 푌̅2 = 푦̅ [ ] (5) 푥̅훽1+훽2 Copyright © 2013 by Modern Scientific Press Company, Florida, USA Int. J. Modern Math. Sci. 2013, 8(3): 149-153 151 푁 ∑푁 (푋 −푋̅)3 where, 훽 = 푖=1 푖 is Co-efficient of Skewness of auxiliary variable and 훽 = 1 (푁−1)(푁−2)푆3 2 푁 ̅ 4 2 푁(푁+1) ∑푖=1(푋푖−푋) 3(푁−1) − is Co-efficient of kurtosis. To the first degree of approximation the bias (푁−1)(푁−2)푆3 (푁−2)(푁−3) and mean square error are given below: (1−푓) 퐵푖푎푠 (푌̅̂ ) = 푌̅(휃2퐶2 − 휃 퐶 퐶 휌) (6) 1 푛 1 푥 1 푥 푦 (1−푓) 푀푆퐸 (푌̅̂ ) = 푌̅2(퐶2 + 휃2퐶2 − 2휃 퐶 퐶 휌) (7) 1 푛 푦 1 푥 1 푥 푦 푋̅훽2 where, 휃1 = 푋̅훽2+훽1 and (1−푓) 퐵푖푎푠 (푌̅̂ ) = 푌̅(휃2퐶2 − 휃 퐶 퐶 휌) (8) 2 푛 2 푥 2 푥 푦 (1−푓) 푀푆퐸 (푌̅̂ ) = 푌̅2(퐶2 + 휃2퐶2 − 2휃 퐶 퐶 휌) (9) 2 푛 푦 2 푥 2 푥 푦 푋̅훽1 where, 휃2 = 푋̅훽1+훽2 In the next section, we will propose a new modified ratio estimator and prove that this proposed estimator is more efficient than the Yan and Tian [5] estimators in all conditions. 3. The Suggested Modified Estimator We propose the modified ratio estimator using the linear combination of Co-Efficient of Kurtosis and Quartile deviation of the auxiliary variable. ̂ 푋̅훽2+ 푄퐷 푌̅푝1 = 푦̅ [ ], (10) 푥̅훽2+ 푄퐷 푁(푁+1) ∑푁 (푋 −푋̅)4 3(푁−1)2 (푄 −푄 ) where, 훽 = 푖=1 푖 − , Co-efficient of kurtosis , 푄. 퐷 = 3 1 ; Quartile 2 (푁−1)(푁−2)푆3 (푁−2)(푁−3) 2 rd st Deviation (where, 푄3 is 3 quartile and 푄1 is 1 quartile of auxiliary variable. ̂ The bias of 푌̅푝1 upto the first degree of approximation is given by; (1−푓) 퐵(푌̅̂ ) = 푌̅(휃2 퐶2 − 휃 퐶 퐶 휌), (11) 푝1 푛 푝1 푥 푝1 푥 푦 MSE of the above estimator can be found using Taylor series method as: Copyright © 2013 by Modern Scientific Press Company, Florida, USA Int. J. Modern Math. Sci. 2013, 8(3): 149-153 152 (1−푓) 푀푆퐸(푌̅̂ ) = 푌̅2(퐶2 + 휃2 퐶2 − 2휃 퐶 퐶 휌) ; (12) 푝1 푛 푦 푝1 푥 푝1 푥 푦 푋̅훽2 where, 휃푝1 = 푋̅훽2+푄퐷 4. Comparison of Efficiency ̂ In this section, we compare the efficiencies for which the estimator given in (10) 푌̅푝1 is more efficient than the existing estimator; ̂ ̂ (휃푝1+휃푖) 퐶푥 푀푆퐸(푌̅푝1) < 푀푆퐸(푌̅푖) if 휌 < ; 1,2 ( Yan and Tian [5] ) (13) 2 퐶푦 5. Numerical Illustration For numerical illustration, we have taken the data from Cochran [2] for the assessment of the performance of the proposed estimator with that of the Yan and Tian [5] estimators. The population parameters are given in Table 1 and Constants, Biasis and MSE’s of estimators are given in Table 2 below: Table 1. Parameters and constants of population 푁 푛 푌̅ 푋̅ 휌 푆푦 퐶푦 푆푥 퐶푥 훽2 훽1 푀푑 푄퐷 49 20 115.153 98.5735 0.5904 98.8285 0.8508 102.97 1.0435 5.9878 2.4224 54 78.5 Table 2. Constants, Biasis and MSE’s of estimators Estimator Constants Bias MSE ̅ 푌̂1Yan and Tian (2010) 0.9959 1.5144 232.7813 ̅ 푌̂2Yan and Tian (2010) 0.9755 1.507 225.2955 ̂ 풀̅풑ퟏ( Proposed Estimator) 0.7527 0.5328 166.708 It is evident from Table 2 that the proposed estimator has smallest bias as well as MSE value as compared to the Yan and Tian [5] estimators. We also calculate the value of the condition given in (13) Copyright © 2013 by Modern Scientific Press Company, Florida, USA Int. J. Modern Math. Sci. 2013, 8(3): 149-153 153 (휃 +휃 ) 퐶 휌 < 푝1 푖 푥 ; 1,2 i.e., 0.5904 < 1.1520. Therefore the above condition is also satisfied for proposed 2 퐶푦 estimator. 5. Conclusions We have developed a new estimator using the linear combination of Co-Efficient of Kurtosis and Quartile deviation of the auxiliary variable. Numerically it has also been proved that our estimator produces smallest bias as well as MSE value as compared to the Yan and Tian [5] estimators. Acknowledgment The first author wishes to record his gratitude and thanks to University Grants Commission, Govt of India, for providing the UGC National Fellowship for perusing the Doctorate prorgramme in Statistics at SKUAST-K. References [1] Deming, W. E., Sampling Techniques. John Wiley & Sons, Inc., New York, 1950. [2] Cochran, W. G., Sampling Techniques, 3rd Edition, John Wiley & Sons, Inc., New York, 1977. [3] Singh, D. and Chaudhary, F. S., Theory and Analysis of Sample Survey Designs, New Age International Publisher, New Delhi, 1986. [4] Sen, A. R., Some early development sin ratio estimation, Biometric Journal, 35(1) (1993): 3- 13. [5] Yan, Z. and Tian, B., Ratio Method to the mean Estimation Using Coefficient of Skewness of Auxiliary variable, ICICA, 2010, Part III, CCIC106, p103-110 Copyright © 2013 by Modern Scientific Press Company, Florida, USA .
Details
-
File Typepdf
-
Upload Time-
-
Content LanguagesEnglish
-
Upload UserAnonymous/Not logged-in
-
File Pages5 Page
-
File Size-