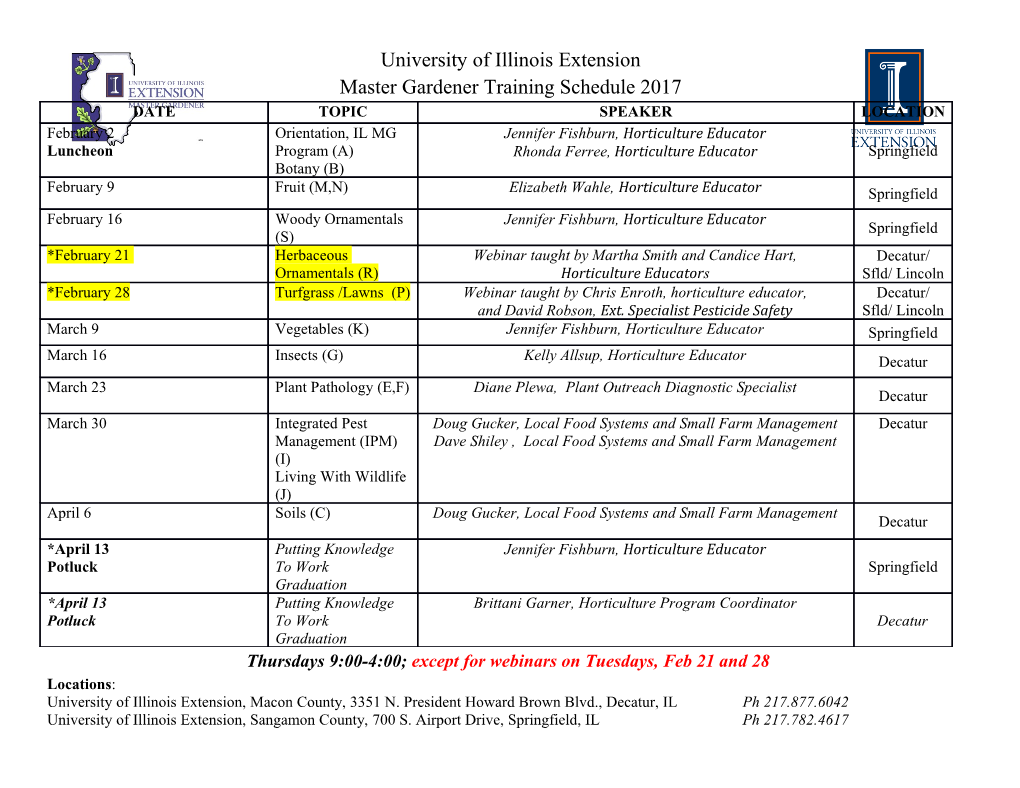
Using More Reasoning to Improve #SAT Solving Jessica Davies and Fahiem Bacchus Department of Computer Science University of Toronto Toronto, Canada [jdavies|fbacchus]@cs.toronto.edu Abstract called component caching DPLL, can achieve a run time that is at least as good as traditional Bayesian network algorithms Many real-world problems, including inference in Bayes (e.g., variable elimination or jointrees), and can on some Nets, can be reduced to #SAT, the problem of counting the instances be super-polynomial faster (Bacchus, Dalmao, & number of models of a propositional theory. This has mo- tivated the need for efficient #SAT solvers. Currently, such Pitassi 2003). In practice, it has been demonstrated that solvers utilize a modified version of DPLL that employs de- component caching DPLL is very effective on many types of composition and caching, techniques that significantly in- Bayes nets including those arising from linkage analysis and crease the time it takes to process each node in the search relational probabilistic models (Chavira & Darwiche 2005; space. In addition, the search space is significantly larger Chavira, Darwiche, & Jaeger 2004). than when solving SAT since we must continue searching Solving #SAT poses some different challenges from ordi- even after the first solution has been found. It has previously nary SAT solving. In particular, in SAT we need only search been demonstrated that the size of a DPLL search tree can be in the space of non-solutions: once a solution is found we significantly reduced by doing more reasoning at each node. can stop. In this non-solution space every leaf of the search However, for SAT the reductions gained are often not worth the extra time required. In this paper we verify the hypoth- tree is a conflict where some clause is falsified. Hence, the esis that for #SAT this balance changes. In particular, we technique of clause learning can be very effective in exploit- show that additional reasoning can reduce the size of a #SAT ing these conflicts to minimize the time spent traversing the solver’s search space, that this reduction cannot always be non-solution space. #SAT solvers, however, must traverse achieved by the already utilized technique of clause learning, the solution space as well as the non-solution space. Con- and that this additional reasoning can be cost effective. flicts are not encountered in the solution space so clause learning is not effective in speeding up search in this part Introduction of the space. Another key factor is that component caching involves a The goal of model counting, #SAT, is to determine the significant overhead at each node of the search tree: the al- number of models of a propositional theory. Like SAT, gorithm must examine the theory for components, compute the canonical NP-complete problem, #SAT is a fundamen- cache keys and perform cache lookups at each node. These tal problem that is complete for the complexity class #P operations are significantly more expensive than the simple (Valiant 1979). This means that many real-world prob- operation of unit propagation performed by SAT solvers. lems can be reduced to #SAT, motivating the need for ef- Finally, #SAT solvers are often used for knowledge com- ficient #SAT solvers. Currently, inference in Bayesian net- pilation (Huang & Darwiche 2005). This involves saving works is the dominant application area of #SAT solving (Li, the search tree explored while solving the #SAT instance (a van Beek, & Poupart 2006; Chavira & Darwiche 2006; trace of DPLL’s execution). A number of different queries Sang, Beame, & Kautz 2005b), but #SAT has also been suc- can then be answered in time linear in the size of the saved cessfully applied to combinatorial problems (Gomes, Sab- search tree. Hence for this important application it can be harwal, & Selman 2006), probabilistic planning (Domshlak beneficial to reduce the size of the explored search tree even & Hoffmann 2006) and diagnosis (Kumar 2002). if more time is consumed. This is analogous to the bene- State-of-the-art #SAT solvers utilize modified versions of fits of using higher levels of optimization in a compiler even DPLL, the classical backtracking search algorithm for solv- when compilation takes more time. ing SAT. The key modification that makes DPLL efficient for In SAT it has been shown that performing more extensive #SAT is the dynamic decomposition of the residual theory inference at each node of the search tree, i.e., going beyond into components, solving those components independently, unit propagation, can significantly decrease the size of the and then caching their solutions so that a component need explored search tree even when clause learning is not em- not be solved repeatedly. It can be shown that this method, ployed (Bacchus 2002). For SAT however, since nodes can Copyright c 2007, Association for the Advancement of Artificial be processed so quickly, the time more extensive inference Intelligence (www.aaai.org). All rights reserved. requires to reduce the number of nodes searched is often not worthwhile. Our hypothesis is that this balance shifts Algorithm 1: Component Caching DPLL for #SAT where processing each node is already much more 1 #DPLL (F ) costly and we might want to minimize the size of the search 2 begin tree in the context of compilation. 3 choose (a variable v ∈ F ) In this paper we verify this hypothesis by applying the 4 foreach ∈{v, ¬v} do techniques of hyper-binary resolution and equality reduction 5 F | = simplify(F ,) originally used for SAT in (Bacchus 2002), and show that 6 if F | contains an empty clause then this usually reduces the size of the explored search tree and 7 count[]=0% clause learning can be done here. can can often reduce the time taken when solving a #SAT 8 else instance. We observe that more reasoning at each node re- 9 count[]=1 duces the size of the residual theory making both compo- 10 C = findComponents(F |) nent detection and caching more efficient. Furthermore, by 11 foreach Ci ∈Cwhile count[] = 0 do (C ) uncovering more forced literals than unit propagation, the 12 cN = getCachedValue i 13 if cN == NOT FOUND then technique can also encourage the partitioning of the theory 14 cN = #DPLL (Ci) into disjoint components which can significantly reduce the × size of the search tree. These two factors help to improve 15 count[ ] = count[ ] cN efficiency in both the solution and non-solution parts of the 16 addCachedValue(F , count[v] + count[¬v]) search space. 17 return (count[v] + count[¬v]) The paper is organized as follows. In the next section we 18 end present some necessary background. Then we present some formal results that allow us to efficiently integrate hyper- binary resolution and equality reduction with component caching. We have constructed a new #SAT solver called we can solve each component independently (lines 11–15). #2clseq by building on the 2clseq solver of (Bacchus 2002), For each component we first examine the cache to see if this and the next section describes some important features of component has been encountered and solved earlier in the the implementation. Experimental results demonstrating the search (line 12). If the component value is not in the cache effectiveness of our approach are presented next followed by we solve it with a recursive call, keeping a running prod- some final conclusions. uct of the solved values (line 15). If any component Ci has #(Ci)=0we can stop: #F | must also be equal to zero. #(F )=#(F | )+#(F | ) Background Finally, v ¬v , so we return that value at line 17 after first inserting it into the cache. In this section we present DPLL with component caching for solving the #SAT problem, and discuss the precise form Hyper-Binary Resolution of extra reasoning that we propose to employ at each node of the search tree. Typically the simplification employed at line 5 is unit prop- agation (UP), which involves assigning all literals appearing Model Counting Using DPLL in clauses of length one the value true, simplifying the re- sulting theory, and continuing until the theory contains no We assume our input is a propositional logic formula F in more unit clauses. In this paper we suggest employing a Conjunctive Normal Form (CNF). That is, it is a conjunction more powerful form of simplification based on the approach of clauses, each of which is a disjunction of literals, each of utilized in (Bacchus 2002). which is a propositional variable or its negation. A truth Definition 1 The Hyper-Binary Resolution (HBR) rule of assignment ρ to the variables of F is a solution to F if ρ inference takes as input a k +1-ary clause (l1,l2,...,lk,x) satisfies F (i.e., makes at least one literal in every clause and k binary clauses each of the form (¬li,y) (1 ≤ i ≤ k) true). The #SAT problem is to determine the number of truth and deduces the clause (x, y). assignments that satisfy F , #(F ). The component caching DPLL algorithm shown in Algorithm 1 computes #(F ). It can be noted that HBR is simply a sequence of resolution This algorithm successively chooses an unassigned vari- steps compressed into a single rule of inference. Its main able v to branch on (line 3), and then counts the number advantage is that instead of generating lengthy clauses it x = y of solutions for F |v and F |¬v, where F | is the simplifi- only generates binary or unary clauses (unary when ). k =1 cation of F by setting to be true (line 14). The sum of When HBR is simply the ordinary resolution of bi- these counts is #(F ) (line 17). Each subproblem, #(F |v) nary clauses.
Details
-
File Typepdf
-
Upload Time-
-
Content LanguagesEnglish
-
Upload UserAnonymous/Not logged-in
-
File Pages6 Page
-
File Size-