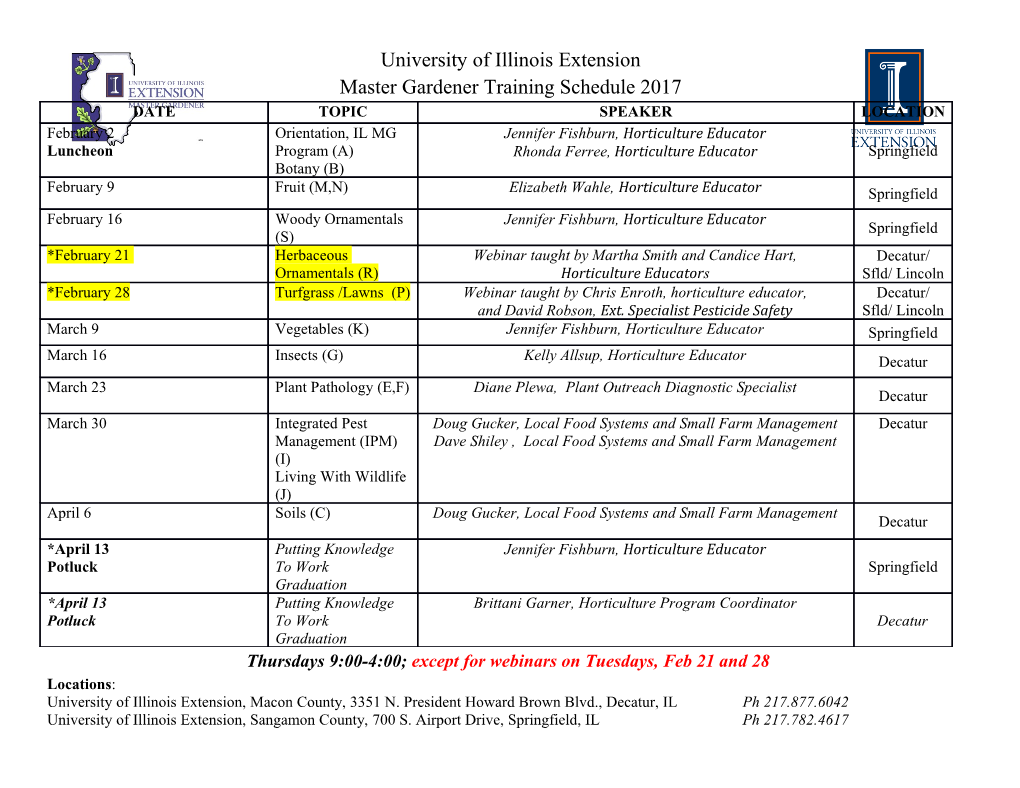
Division of Applied Mathematics Colloquium Thursday, October 10, 2019, 12:00 noon - 1:00pm 170 Hope Street, Room 108 Speaker: Constantinos Daskalakis, MIT Title: Statistical Inference from Dependent Data Abstract: Statistical Learning Theory has largely focused on learning and generalization from independent and identically distributed observations. This assumption is, however, too strong. In many applications, observations are collected on nodes of a network, or some spatial or temporal domain, and are dependent. Examples abound in financial and meteorological applications, and dependencies naturally arise in social networks through peer effects. We study the basic statistical tasks of linear and logistic regression on networked data, where the responses on the nodes of the network are not independent conditioning on the nodes’ vectors of covariates. Given a single observation (across the whole network) from a networked linear or Professor of Computer Science at MIT and a member of CS-AI logistic regression model and under necessary weak dependency Lab. He works on computation theory and its interface with game theory, economics, probability theory, statistics and assumptions, we prove strong consistency results for estimating the model machine learning. He holds a PhD in Computer Science from parameters, recovering the rates achievable in the standard setting with UC-Berkeley. He is the recipient of the Nevanlinna Prize from independent data. We generalize these results beyond linear and logistic the International Mathematical Union, the Kalai Prize from the regression, assuming that the observations satisfy Dobrushin’s condition, Game Theory Society, the ACM Grace Murray Hopper Award, showing how to use Gaussian complexity and VC dimension to control the Simons investigator award, the Bodossaki Foundation Distinguished Young Scientists Award, the SIAM outstanding generalization error. paper prize, the ACM Doctoral Dissertation award.
Details
-
File Typepdf
-
Upload Time-
-
Content LanguagesEnglish
-
Upload UserAnonymous/Not logged-in
-
File Pages1 Page
-
File Size-