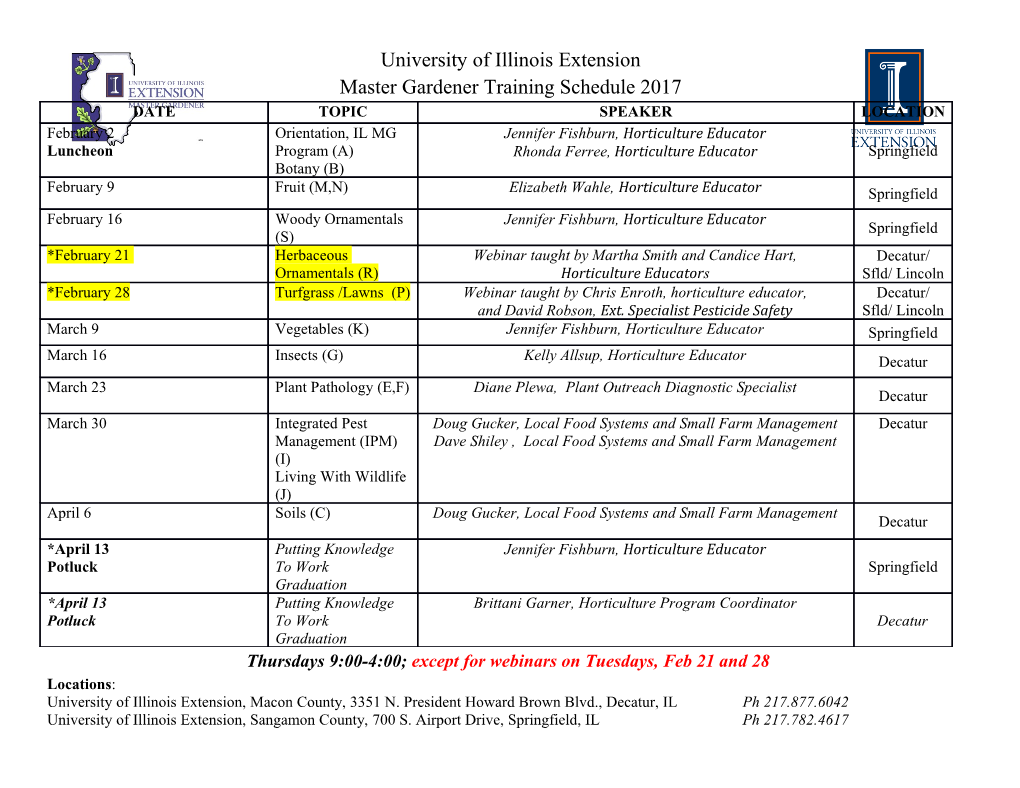
Math 1180, 2009 Notes 19 1 Some general facts about eigenvalues All of the matrices in this set of notes are square matrices. De nition 1 The \trace" of an n n matrix A = (aij) is the sum of the diagonal elements: n tr (A) = aii i=1 X Theorem 2 If A and B are similar, then det A = det B and tr(A) = tr(B) Proof. From the de nition of similarity, there is a nonsingular matrix M such 1 that M AM = B. We saw already that 1 1 det B = det M AM = det M det A det M: But 1 1 det M det M = det M M = det I = 1; so det B = det A: For the trace, we need a lemma. Lemma 3 For any n n matrices A and B, tr (AB) = tr (BA) : Proof. We have n (AB)ii = aijbji; j=1 X so n n n n n tr (AB) = (AB)ii = aijbji = aijbji i=1 i=1 j=1 ! j=1 i=1 ! X X X X X n n n = bjiaij = (BA)jj j=1 i=1 ! j=1 X X X 1 Continuing with the proof of the Theorem, we then have 1 1 1 tr (B) = tr M AM = tr M (AM) = tr (AM) M = tr (A) We apply this theorem to get some facts about eigenvalues of a matrix. To do this, we consider once again the characteristic polynomial. Theorem 4 For any n n matrix A, with eigenvalues 1; 2; ::::; n; tr (A) = 1 + 2 + + n det A = 12 n: In other words, the trace of A is the sum of the eigenvalues, and the determinant of A is the product of the eigenvalues. Proof. We will only prove this theorem for diagonalizable matrices. We know that if A is similar to the diagonal matrix D, then the eigenvalues of A and D are the same. From the previous theorem, A and D have the same trace and same determinant. But it is obvious that for D the sum of the eigenvalues is the trace, and the product of the eigenvalues is the determinant, because the eigenvalues are the diagonal elements. This proves the theorem for a diagonalizable matrix. In the homework you are asked to prove the theorem for any 2 2 matrix, whether or not it is diagonalizable. Application: Find the eigenvalues of 1 1 : 2 2 Solution: By the theorem, 1 + 2 = 3; 12 = 0: Hence, 1 = 0; 2 = 3: "Spectral Theorem" This is a result about diagonalizing symmetric matrices. It turns out that sym- metric matrices can always be diagonalized. Furthermore, they can be diagonalized using special kinds of matrices. (That is, the matrix M used in the diagonalization can be special. This is indicated by using a di erent letter from M .) Some authors consider this the most important theorem in the course. 2 Theorem 5 If A is a n n symmetric matrix (AT = A), then (i) All eigenvalues of Aare real (ii) Eigenvalues corresponding to di erent eigenvalues are orthogonal (iii) there is an orthonormal basis for Rn consisting of eigenvectors of A. Corollary 6 If A is an n n symmetric matrix, then A is diagonalizable, and the matrix of eigenvectors used for the diagonalization can be chosen to be an orthogonal matrix. (QT Q = I). We shall not have time to prove this. It is stated and proved in the text, pages 580-582. But I will give a examples, and assign some homework Example 6 2 A = : (1) 2 9 We nd the eigenvalues: (6 ) (9 ) 4 = 0 2 15 + 50 = 0 ( 5) ( 10) = 0 So 1 = 5; 2 = 10: We nd the eigenvectors: 1 2 A 5I = : 2 4 2 From this we see that an eigenvector is : 1 4 2 A 10I = : 2 1 1 A second eigenvector is therefore : Notice that the two eigenvectors are or- 2 thogonal to each other. 3 2 1 The usual matrix of eigenvectors that you would choose is M = : 1 2 The columns are orthogonal, but they don't have length one, so this is not what is called an \orthogonal matrix." We must normalize the columns, which gives the orthogonal matrix 2 1 p5 p5 Q = 1 2 p5 p5 ! Now the columns of Q are orthogonal and of length one. You can easily check that T 1 T Q Q = I: This is the same as saying that Q = Q . Now we diagonalize A: 2 p 1 p 2 1 1 T 5 5 5 5 6 2 p5 p5 Q AQ = Q AQ = 1 2 1 p5 2 p5 2 9 5 5 p5 p5 ! 5 0 = 0 10 (as you can check!) 2nd example: Let 0 1 0 A = 1 0 0 0 0 0 1 1 This is a \block diagonal" matrix, meaning@ thatA it can be written as B 0 A = ; 0 C where B and C are square matrices. Here, B is 2 2 and C is 1 1. The 0's represent matrices of all zeros, which are generally not square. The eigenvalues of a block diagonal matrix can be found from the eigenvalues of the individual matrices in the block. In this case, the eigenvalues of B are 1 and 1, and the eigenvalue of C is 1. They are all real, as required by part (i) of the spectral theorem. For A; the eigenvalues are 1 = 1; with algebraic multiplicity two, and 2 = 1, with algebraic multiplicity 1. We now nd eigenvectors. 1 1 0 A 1I = 1 1 0 0 0 0 0 1 @ A 4 1 Since the rst two rows are linearly dependent, we see that v1 = 1 and v2 = 0 0 1 1 @ A 1 are eigenvectors. (Other eigenvectors could be chosen, but let's stick with 0 1 1 @theseA for the moment.) The geometric multiplicity equals the algebraic multiplicity, as is required for a diagonalizable matrix. Also 1 1 0 A 2I = 1 1 0 : 0 0 0 2 1 @ 1 A Any eigenvector is a multiple of v3 = 1 . Notice that this eigenvector is 0 0 1 orthogonal to v1 and v2, as stated in part@ (ii) ofA the spectral theorem. We now want to diagonalize A using an orthogonal matrix Q. Our rst guess is that we can use the eigenvectors to form Q: This would give 1 1 1 Q = 1 1 1 : 0 0 1 0 1 @ A But the rst column is not orthogonal to the second column. Also, none of the columns have length one. We can x the second problem easily, while the rst problem requires a little thought. The solution is to choose di erent eigenvectors for 1: In a large matrix this can be done using the Gram-Schmidt method. Here we can do it by inspection. 1 0 Instead of choosing 1 ; choose 0 : This is still an eigenvector, and now it 0 1 1 0 1 1 is orthogonal to each@ of theA other eigenvectors.@ A The nal step is to normalize, and we get 1 0 1 p2 p2 Q = 1 0 1 : 0 p2 p2 1 0 1 0 @ A 5 1 0 0 T T 1 We now want Q AQ = D = 0 1 0 ; or, since Q = Q , 0 0 0 1 1 @ A AQ = QD: This is pretty easy to check. Homework. Due on Wednesday, December 9 (There will be one or two further problems assigned later this week. These will be due on Friday, Dec. 11. ) 1. Prove that for any 2 2 matrix, whether or not it is diagonalizable, the sum of the eigenvalues is the trace and the product of the eigenvalues is the determinant. 2. pg. 583, # 2 3. pg:583, # 5 6.
Details
-
File Typepdf
-
Upload Time-
-
Content LanguagesEnglish
-
Upload UserAnonymous/Not logged-in
-
File Pages6 Page
-
File Size-