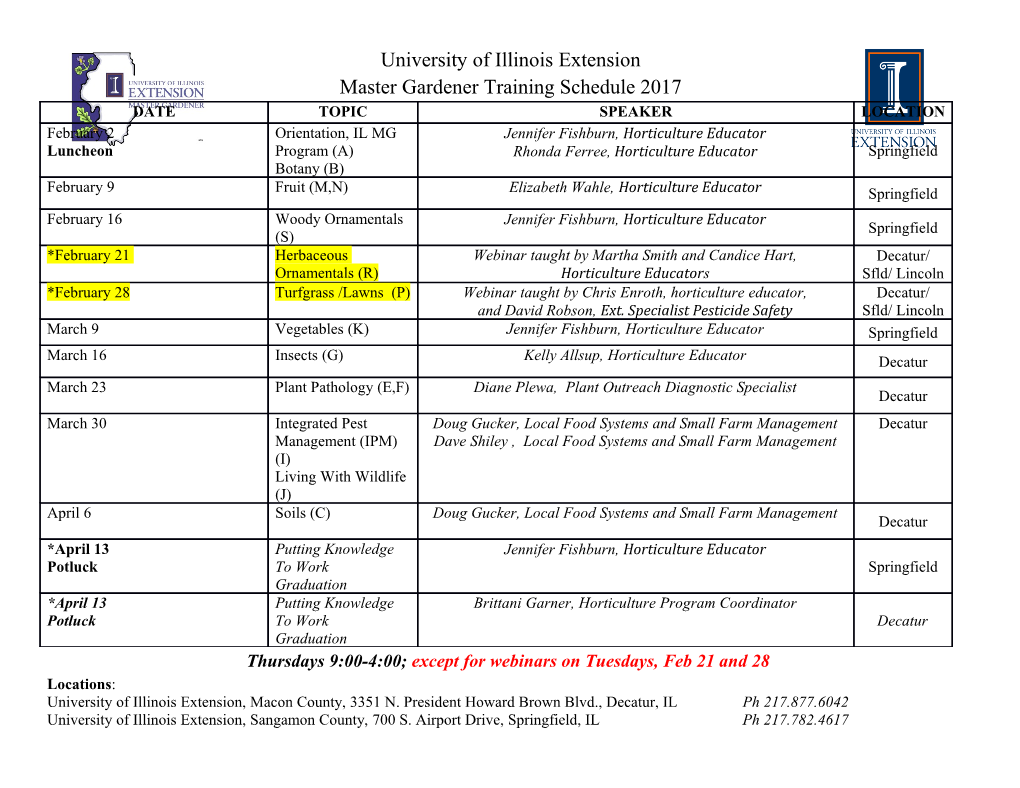
Constructing, comparing, and reconstructing networks by Brennan Klein B.A. in Cognitive Science & Psychology, Swarthmore College A dissertation submitted to The Faculty of the College of Science of Northeastern University in partial fulfillment of the requirements for the degree of Doctor of Philosophy November 19, 2020 Dissertation Committee Alessandro Vespignani, Chair Samuel V. Scarpino, Co-chair Tina Eliassi-Rad Laurent H´ebert-Dufresne 1 Acknowledgements The thanks I give in this section will never|and can never|fully capture the extent of my gratitude. I cherish the friends, mentors, collaborators, and altogether supportive people in my life. Because of them, I have grown immensely as a scientist. Because of them, I have grown even more as a person. Because of them, I can go forth into this next stage of my life, full of a deep faith in what's to come, supported by a network of endlessly kind and brilliant people. My dissertation committee|Laurent H´ebert-Dufresne, Tina Eliassi-Rad, Sam Scarpino, and Alex Vespignani|is a perfect example of this network of support. It has been a privilege to share this dissertation with them over these last few years. One of the greatest joys in my life has been my friendship with Conor Heins. We grew up together in science, and there are ideas that I simply cannot grasp without his presence. If there is one thing I have learned throughout my short career in science, it is the irreplaceable role that friendship has in driving discovery. To my parents, Marsha and Don, I owe so much. This dissertation would not exist|and I would not be a scientist|without my brother, Jason. Documents like these are, ironically, never comprehensive enough. I spent months compiling a list of all the people I wanted to thank, all the memories I wanted to share, to reminisce over, to try and inspire through. At the same time, I am writing this document in the midst of a year of devastation from the COVID-19 pandemic. For some strange and sad reason, I cannot bring myself to write the names of every person I want to acknowledge. As a result this section may seem artificially short or otherwise rushed. In place of a fuller list of acknowledgements, I make this promise to these cherished people in my life: That the acknowledgements will come in person, sporadically, surprisingly, over next several years of our lives together. I hope we recognize each other. 2 Abstract of Dissertation Complex networks are the syntax of complex systems; they are models that allow us to study phenomena across nature and society. And because they are models, the famous \all models are wrong, but some are useful" quotation rings especially true. We need to use the right networks to properly study complex systems, and in order to do so, the methods we use to create and analyze networks must be fit for purpose. This motivation has guided much of my dissertation, and in it, I explore three related themes around constructing, comparing, and reconstructing complex networks. In the first chapter, I describe a theoretical and computational infrastructure that allows us to ask whether a given network captures the most informative scale to model the dynamics in the system. We see that many real world networks (especially heterogeneous networks) exhibit an information holarchy whereby a coarse-grained, macroscale representation of the network has more effective information than the original microscale network. In the next chapter, I consider the challenging problem of comparing pairs of networks and quantifying their differences. These tools are broadly referred to as \graph distance" measures, and there are dozens used throughout Network Science. However, unlike in other domains of Network Science where rigorous benchmarks have been established to compare our surplus of tools, there is still no theoretically-grounded benchmark for characterizing these tools. To address this, collaborators and I proposed that simple, well-understood ensembles of random networks are natural benchmarks for network comparison methods. In this chapter, I characterize over 20 different graph distance measures, and I show how this simple within-ensemble graph distance can lead to the development of new tools for studying complex networks. The final chapter is an example of exactly that: I show how the within-ensemble graph distance can be used to characterize and evaluate different techniques for reconstructing networks from time series data. Tying together the original theme of using the \right" network, this chapter addresses one of the most fundamental challenges in Network Science: how to study networks when the network structure is not known. Whether it's reconstructing the network of neurons from time series of their activity, or identifying whether one stock's price fluctuations cause changes in another's, this problem is ubiquitous when studying complex systems; not only that, there are (again) dozens of techniques for transforming time series data into a network. In this chapter, I measure the within-ensemble graph distance between pairs of networks that have been reconstructed from time series data using a given reconstruction technique. What I find is that different reconstruction techniques have characteristic distributions of distances and that certain techniques are either redundant or underspecified given other more comprehensive methods. Ultimately, the goal of this dissertation is to stress the importance of rigorous standards for the suite of tools we have in Network Science, which ultimately becomes an argument about how to make Network Science more useful as a science. 3 Table of Contents Acknowledgements.....................................2 Abstract of Dissertation..................................3 Table of Contents......................................4 List of Figures.......................................7 List of Tables........................................ 14 Chapter 1 Introduction................................. 15 1.1 Science in Network Science...................... 16 1.1.1 What makes a science a science?.............. 17 1.2 Theory in Network Theory...................... 20 1.2.1 Networks as data objects................... 22 1.2.2 Networks as generative models of data........... 22 1.2.3 Networks as hypotheses.................... 23 1.3 The current dissertation....................... 24 Chapter 2 Constructing: Informative higher scales in complex networks...... 26 2.1 Introduction.............................. 26 2.2 Results................................. 28 2.2.1 Effective information..................... 28 2.2.2 Determinism and degeneracy................. 31 2.2.3 Effective information in real networks............ 32 2.2.4 Causal emergence in complex networks........... 34 2.2.5 Network macroscales..................... 36 2.2.6 Causal emergence reveals the scale of networks....... 37 2.2.7 Causal emergence in real networks.............. 39 2.3 Discussion............................... 40 2.4 Materials and Methods........................ 42 2.4.1 Selection of real networks................... 42 2.4.2 Creating consistent macro-nodes............... 43 2.4.3 Greedy algorithm for causal emergence........... 43 2.5 Follow-up research: Biological networks............... 44 2.5.1 Background: Noise in biological systems.......... 44 2.5.2 Effectiveness of interactomes across the tree of life..... 47 2.5.3 Causal emergence across the tree of life........... 48 2.5.4 Resilience of macroscale interactomes............ 49 2.5.5 Discussion........................... 52 2.5.6 Protein interactome data................... 55 2.5.7 Robustness of causal emergence............... 55 4 Chapter 3 Comparing: The within-ensemble graph distance............. 61 3.1 Introduction.............................. 61 3.1.1 Formalism of graph distances................ 62 3.1.2 This study........................... 64 3.2 Methods................................ 64 3.2.1 Ensembles........................... 64 3.2.2 Graph distance measures................... 66 3.2.3 Description of experiments.................. 67 3.3 Results................................. 69 3.3.1 Results for homogeneous graph ensembles......... 69 3.3.2 Results for sparse heterogeneous ensembles......... 75 3.4 Discussion............................... 79 Chapter 4 Reconstructing: Comparing ensembles of reconstructed networks.... 82 4.1 Introduction to the netrd package.................. 82 4.1.1 Network reconstruction from time series data........ 84 4.1.2 Simulated network dynamics................. 84 4.1.3 Comparing networks using graph distances......... 84 4.1.4 Related software packages.................. 84 4.2 Introduction to the ( ; ; ) ensemble............... 86 4.2.1 Framing: A distributionG D R of ground truths.......... 87 4.2.2 The ( ; ; ) ensemble.................... 90 4.3 Methods................................G D R 92 4.3.1 The standardized graph distance............... 92 4.3.2 Description of experiments.................. 93 4.4 Results................................. 93 4.5 Discussion............................... 96 Chapter 5 Conclusion.................................. 100 References.......................................... 102 Appendices......................................... 118 6.1 Chapter 2 Appendix......................... 118 6.1.1 Table of key terms...................... 118 6.1.2 Effective information calculation............... 118 6.1.3 Effective information of common network structures.... 121 6.1.4 Network motifs as causal relationships........... 124 6.1.5 Table of
Details
-
File Typepdf
-
Upload Time-
-
Content LanguagesEnglish
-
Upload UserAnonymous/Not logged-in
-
File Pages146 Page
-
File Size-