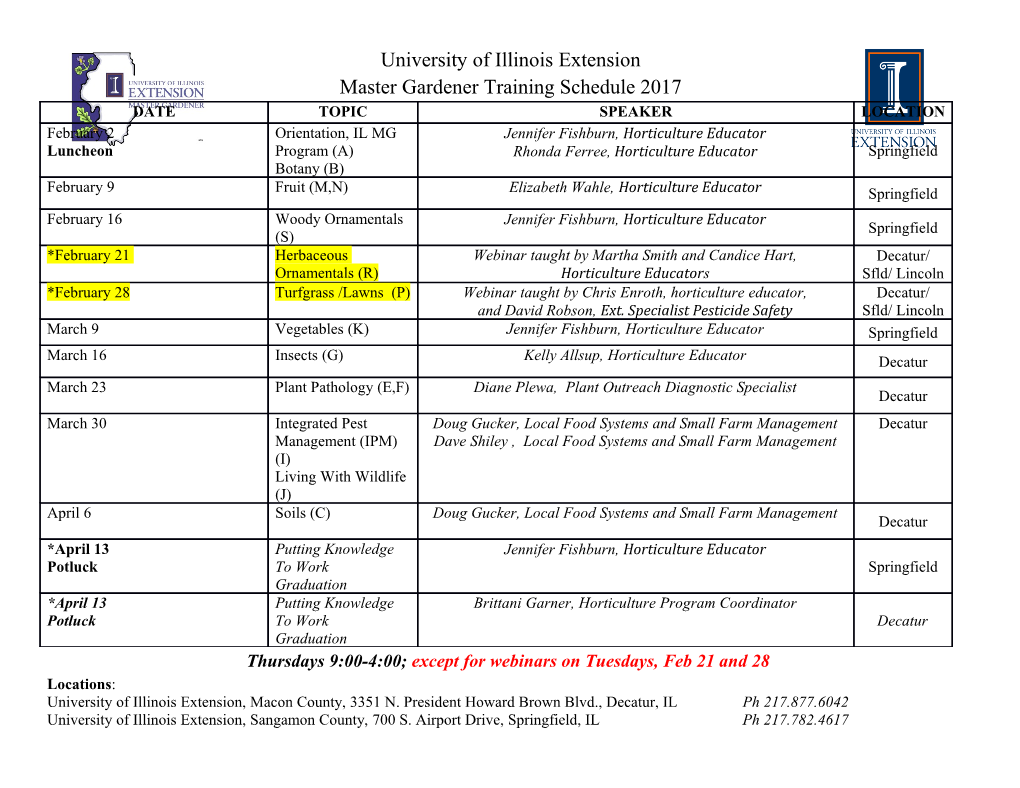
Survival Analysis APTS 2016/17 Ingrid Van Keilegom ORSTAT KU Leuven Glasgow, August 21-25, 2017 Basic concepts Nonparametric estimation Hypothesis testing in a nonparametric setting Proportional Basic concepts hazards models Parametric survival models What is ‘Survival analysis’ ? Basic concepts Survival analysis (or duration analysis) is an area of Nonparametric estimation statistics that models and studies the time until an Hypothesis event of interest takes place. testing in a nonparametric setting In practice, for some subjects the event of interest Proportional cannot be observed for various reasons, e.g. hazards models • the event is not yet observed at the end of the study Parametric • another event takes place before the event of interest survival models • ... In survival analysis the aim is to model ‘time-to-event data’ in an appropriate way to do correct inference taking these special features of the data into account. Examples Basic concepts Medicine : Nonparametric • time to death for patients having a certain disease estimation • time to getting cured from a certain disease Hypothesis testing in a • time to relapse of a certain disease nonparametric setting Agriculture : Proportional hazards • time until a farm experiences its first case of a certain models disease Parametric survival models Sociology (‘duration analysis’) : • time to find a new job after a period of unemployment • time until re-arrest after release from prison Engineering (‘reliability analysis’) : • time to the failure of a machine Common functions in survival analysis Basic concepts Let T be a non-negative continuous random variable, Nonparametric representing the time until the event of interest. estimation Hypothesis Denote testing in a nonparametric F(t) = P(T ≤ t) distribution function setting f (t) probability density function Proportional hazards models For survival data, we consider rather Parametric survival S(t) survival function models H(t) cumulative hazard function h(t) hazard function mrl(t) mean residual life function Knowing one of these functions suffices to determine the other functions. Survival function : Basic concepts S(t) = P(T > t) = 1 − F(t) Nonparametric estimation Hypothesis Probability that a randomly selected individual will testing in a nonparametric survive beyond time t setting Decreasing function, taking values in [0; 1] Proportional hazards models Equals 1 at t = 0 and 0 at t = 1 Parametric survival models Cumulative hazard function : H(t) = − log S(t) Increasing function, taking values in [0; +1] S(t) = exp(−H(t)) Hazard function (or hazard rate) : Basic P(t ≤ T < t + ∆t j T ≥ t) concepts h(t)= lim Nonparametric ∆t!0 ∆t estimation 1 P(t ≤ T < t + ∆t) Hypothesis = lim testing in a P(T ≥ t) ∆t!0 ∆t nonparametric f (t) −d d setting = = log S(t) = H(t) Proportional S(t) dt dt hazards models Parametric h(t) measures the instantaneous risk of dying right survival models after time t given the individual is alive at time t Positive function (not necessarily increasing or decreasing) The hazard function h(t) can have many different shapes and is therefore a useful tool to summarize survival data Hazard functions of different shapes Basic concepts 10 Nonparametric estimation Exponential Weibull, rho=0.5 Hypothesis 8 Weibull, rho=1.5 Bathtub testing in a nonparametric setting 6 Proportional hazards models Hazard Parametric 4 survival models 2 0 0 5 10 15 20 Time Basic concepts Mean residual life function : Nonparametric The mrl function measures the expected remaining estimation Hypothesis lifetime for an individual of age t. As a function of t, we testing in a have nonparametric R 1 setting S(s)ds mrl(t) = t Proportional ( ) hazards S t models This result is obtained from 1 Parametric R (s − t)f (s)ds survival mrl(t) = E(T − t j T > t)= t models S(t) Mean life time : Z 1 Z 1 E(T ) = mrl(0) = sf (s)ds = S(s)ds 0 0 Incomplete data Basic concepts Censoring : Nonparametric • For certain individuals under study, the time to the event estimation of interest is only known to be within a certain interval Hypothesis testing in a • Ex : In a clinical trial, some patients have not yet died at nonparametric setting the time of the analysis of the data Proportional ) Only a lower bound of the true survival time is known hazards models (right censoring) Parametric survival Truncation : models • Part of the relevant subjects will not be present at all in the data • Ex : In a mortality study based on HIV/AIDS death records, only subjects who died of HIV/AIDS and recorded as such are included (right truncation) Censoring and truncation do not only take place in Basic concepts ‘time-to-event’ data. Nonparametric estimation Examples Hypothesis testing in a Insurance : Car accidents involving costs below a nonparametric setting certain threshold are often not declared to the Proportional insurance company hazards models ) Left truncation Parametric Ecology : Chemicals in river water cannot be detected survival models below the detection limit of the laboratory instrument ) Left censoring Astronomy : A star is only observable with a telescope if it is bright enough to be seen by the telescope ) Left truncation Basic concepts Nonparametric estimation Right censoring Hypothesis Only a lower bound for the time of interest is known testing in a nonparametric setting T = survival time Proportional C = censoring time hazards models ) Data : (Y ; δ) with Parametric survival Y = min(T ; C) models δ = I(T ≤ C) Type I right censoring Basic All subjects are followed for a fixed amount of time concepts ! all censored subjects have the same censoring time Nonparametric estimation Ex : Type I censoring in animal study Hypothesis testing in a nonparametric setting Proportional hazards models Parametric survival models Basic concepts Nonparametric Type II right censoring estimation Hypothesis All subjects start to be followed up at the same time and testing in a nonparametric follow up continues until r individuals have experienced setting the event of interest (r is some predetermined integer) Proportional hazards ! The n − r censored items all have a censoring time models equal to the failure time of the r th item. Parametric survival Ex : Type II censoring in industrial study : all lamps are models put on test at the same time and the test is terminated when r of the n lamps have failed. Basic concepts Random right censoring Nonparametric estimation The study itself continues until a fixed time point but Hypothesis subjects enter and leave the study at different times testing in a nonparametric ! censoring is a random variable setting ! censoring can occur for various reasons: Proportional – end of study hazards models – lost to follow up Parametric – competing event (e.g. death due to some cause other survival than the cause of interest) models – patient withdrawing from the study, change of treatment, ... Ex : Random right censoring in a cancer clinical trial Basic concepts Example : Random right censoring in HIV study Nonparametric estimation Study enrolment: January 2005 - December 2006 Hypothesis testing in a Study end: December 2008 nonparametric setting Objective: HIV patients followed up to death due to Proportional hazards AIDS or AIDS related complication (time in month from models confirmed diagnosis) Parametric survival Possible causes of censoring : models • death due to other cause • lost to follow up / dropped out • still alive at the end of study Table: Data of 6 patients in HIV study Basic Patient id Entry Date Date last seen Status Time Censoring concepts 1 18 March 2005 20 June 2005 Dropped out 3 0 Nonparametric 2 19 Sept 2006 20 March 2007 Dead due to AIDS 6 1 estimation 3 15 May 2006 16 Oct 2006 Dead due to accident 5 0 4 01 Dec 2005 31 Dec 2008 Alive 37 0 Hypothesis 5 9 Apr 2005 10 Feb 2007 Dead due to AIDS 22 1 testing in a 6 25 Jan 2005 24 Jan 2006 Dead due to AIDS 12 1 nonparametric setting Proportional hazards models Parametric survival models Left censoring Basic Some subjects have already experienced the event of concepts interest at the time they enter in the trial Nonparametric estimation Only an upper bound for the time of interest is known Hypothesis ) Data : (Y ; δ ) with testing in a ` ` nonparametric setting Y` = max(T ; C`) Proportional δ` = I(T > C`) hazards models C` = censoring time Parametric survival Ex : Left censoring in malaria trial models • Children between 2 and 10 years are followed up for malaria • Once children have experienced malaria, they will have antibodies in their blood against the Plasmodium parasite • Children entered at the age of 2 might have already been in touch with the parasite Basic concepts Interval censoring Nonparametric estimation The event of interest is only known to occur within a Hypothesis certain interval (L; U) testing in a nonparametric setting Contrary to right and left censoring, we never observe Proportional the exact survival time hazards models Typically occurs if diagnostic tests are used to assess Parametric the event of interest survival models Ex : Interval censoring in malaria trial ! The exact time to malaria is between the last negative and the first positive test Basic concepts Truncation : Individuals of a subset of the population of Nonparametric interest do not appear in the sample estimation Hypothesis testing in a Left truncation nonparametric setting Occurs often in studies where a subject must first meet Proportional a particular condition before he/she can enter in the hazards models study and followed up for
Details
-
File Typepdf
-
Upload Time-
-
Content LanguagesEnglish
-
Upload UserAnonymous/Not logged-in
-
File Pages183 Page
-
File Size-