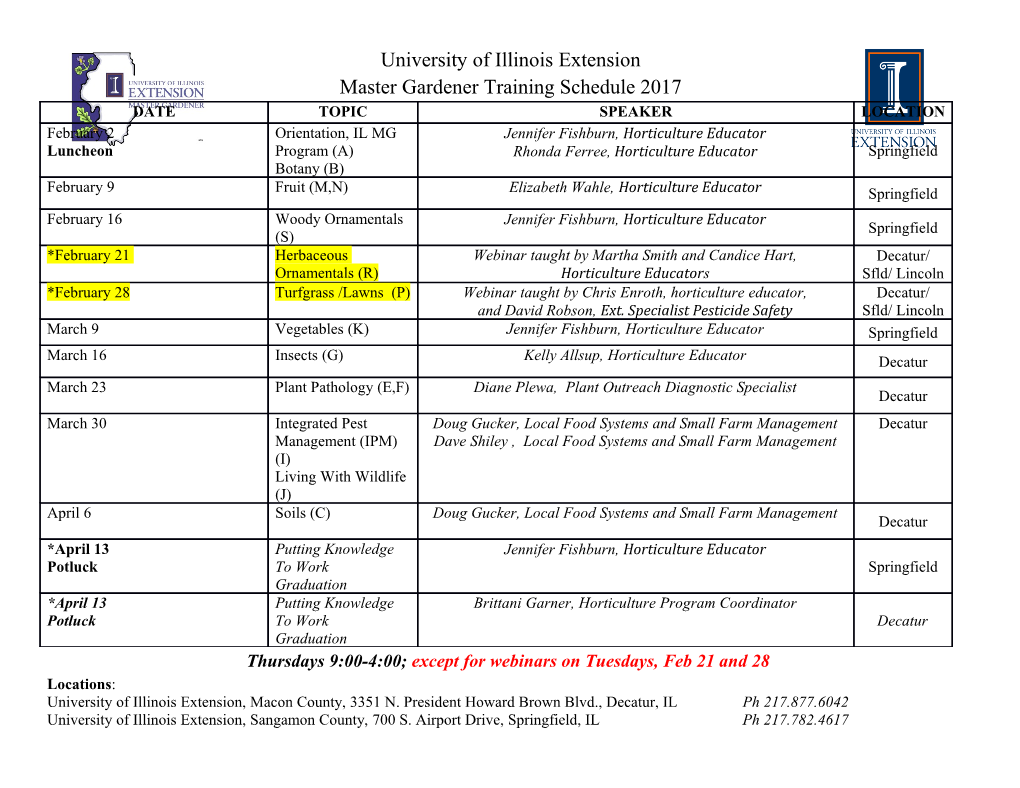
PATTERN'RECOGNITION' AND MACHINE'LEARNING CHAPTER'5:'NEURAL'NETWORKS Include(Nonlinearity(g(x)(in(Output( with(Respect(to(Input Yk(X)(=(g((! wki(xi( +(wk0((),(k(=(1,(…,(K Y1(X)$…$$$$Yk(X) g($) g($) Output w_11$$$$$$$$$$$$$$$$$$$$$$$$$$$$$$$w_1d$$$$$$$$$$$$$$$$$$w_0 Input x_1$$$x_2$$$$$x_3$$$$x_4$$$$$…$$$$$$$$x_d$$$$$$$$$$$$$$$$x_0 Using$sigmoid$nonlinearity$and$normal$class$conditional$probability,$the$ output$oF$a$NN$discriminant$is$interpreted$as$posteriori$probabilities$p(x|Ck) Mapping'Arbitrary'Boolean'Function Input&vector:' length'd,'all'components'0'or'1 Output: 1:'if'the'given'input'is'class'A,'0:'if'input'from'class'B Total'2^d'inputs;'say'K'are'in'class'A,'2^d'C K'in'B 2&layers&of&FF&NN Input'size'2^d;'Hidden'size'K;'Output'size'1;'hardlim threshold'funct. Weights Input'C>'Hidden:'1'if'given'input'is'in'A'and'has'1at'the'node;'C1'otherw Hidden'C>'Out:'all'1;'Bias/hidden:'1Cb:if'node'K'has'b'ones;' Prove!:'this'NN'gives'1'if'input'from'A,'0'from'B. Mapping'Arbitrary'Function' with'34layer'FFNN Single'neuron'threshold 4>'half4space 2'layer'NN 4>'convex'region Output'bias:'4M(hidden'u.)'gives'logical'AND 3'layer'NN 4>'any'region'!!! Subdivide'input'into'approx.'hypercubes A'cluster'of'd'1st'hidden'nodes'maps'one'cube Bias'41'means'logical'OR'at'output Can'produce'any'combination'of'input'cubes' 3"Layer(Neural(Network((1(hidden(layer) Kolmogorov(Approximation(Theorem( (1957) Discovered(independently(of(NNs Related(to(Hilbert’s 23(unsolved(problems/1900 #13:(Can(a(function(of(several(variaBles(Be(represented(as(a( comBination(of(functions(of(a(few(variaBle.(Arnold:(yes(N 3( variaBle(with(2! Kolmogorov(AN: Any(multivariaBle(continuous(function(can(Be(expressed(as( superposition(of(functions(of(one(variaBle((a(small(numBer( of(components) Limitations: not(constructive,(too(complicated Example:)3+layer,)feedforward)ANN) with)supervised)learning Motivation)for)3+layer:)Kolmogorov)Representation)Theorem y y8 9 9 8 w94 w w w87 w 95 w w96 86 84 85 w97 4 5 6 7 w61 w w w53 w63 w w41 w51 w 52 62 w 73 42 w72 43 w71 1 2 3 x2 x3 x1 Example:)3+layer,)FF)ANN(cont’d) Assume:)transfer)function)is)just)summation v v v 4 5 6 v7 4 5 6 7 w61 w w62 w53 w63 w w41 w51 w 52 w 73 42 w72 43 w71 1 2 3 x x x1 2 3 &v # &w w w # 4 41 42 43 &x # Input0to0 $v ! $w w w ! 1 $ 5 ! = $ 42 52 53 !$x ! hidden0 $ 2 ! $v6 ! $w 43 w 62 w 63 ! weights $ ! $ !%$x3 "! %v7 " %w 44 w 72 w 73 " Example:)3+layer,)FF)ANN(cont’d) hidden)to)output)weights &v4 # y w w w w $v ! & 8 # & 84 85 86 87 #$ 5 ! $ ! = $ ! %y9 " %w 94 w 95 w 96 w 97 "$v6 ! $ ! %v7 " y y8 9 9 8 w 94 w w95 w w86 87 w84 w85 96 v v v 4 5 6 v7 Example:&34layer,&FF&ANN(cont’d) Final&matrix&representation&for&linear&system V = WBAX Y = WcbV = WCBWBAX y9 &w 41 w 42 w 43 # y8 $ !&x1 # &y # &w w w w # w w w 8 = 84 85 86 87 $ 42 52 53 !$x ! $ ! $ ! $ 2 ! %y9 " %w 94 w 95 w 96 w 97 "$w 43 w 62 w 63 ! $ !%$x3 "!9 8 Layer1C %w 44 w 72 w 73 " w94 w w w87 w 95 w w96 86 84 85 w97 Layer1B 4 5 6 7 w61 w w w53 w63 w w41 w51 w 52 62 w 73 42 w72 43 w71 Layer1A 1 2 3 x1 x2 x3 Transfer(Functions May(be(a(threshold(function(that(passes(information( ONLY(IF(the(output(exceeds(the(threshold Can(be(a(continuous(function(of(the(input The(output(is(usually(passed(to(the(output(path(of( the(node Example(of(transfer(function:(sigmoid Examples)of)Approximation) (with)3)hidden)nodes)) Approximation+by+gradient+descent Often+not+practical+to+directly+evaluate Use+approximation+of+the+error+function+by+iteration: dE/dw =+0+ Gradient+descent+idea:+! if+we+are+at+a+given+location+ (given+w+parameters+to+be+optimized],+then+change+w+in+ a+way+where+the+gradient+of+the+error+is+the+max W(t+1)+=+W(t)+– !dE/dW Continue+the+iteration+until+Converges+to+the+minimum Not+always+converges! Illustration+of+the+Error+Surface Learning(with( Error(Backpropagation((BP) Learning:( determine(the(weights(of(the(NN Assume: Structure(is(given Transfer(functions(are(given Input(A output(pair(are(given( Supervised(learning(based(on(examples! See:(derivation(of(backpropagation Backpropagation,Learning Paul,Werbos,(1974) PhD,work,Princeton Roots,of,backpropagation Rumelhart,,McClelanD,(1986) PDP,group,at,CMU Popularization,of,the,iDea http://scsnl.stanford.eDu/conferences/NSF_Brain_Network_Dynamics_Jan2007 http://www.archive.org/search.php?query=2007+brain+network+Dynamics Supervised*Learning*Scheme Standard'Backpropagation'– delta'rule Gradient'of'the'sum'squared'error & 'F 'F 'F # ( ) F(w) = ($ , ,..., ! w $ ! % 'w1 'w2 'wQ " Backpropagation'delta9rule N N %F 1 %Fk 1 k k = lim = lim & li z(l"1) j N $# ! N $# ! %wp N k =1 %wlij N k =1 Weight'change'algorithm'iteratively'from'top'layer' N backward new old 1 k k wlij = wlij !% lim & li z(l!1) j N #" $ N k =1 or new old k k wlij = wlij !%& li z(l!1) j Generalized ! -rule 2 1 N where: FYxYxw= * ()! (,) Fw()= lim Fk (); w k kk N !" # N k =1 N batch size #Fw() 1N #Fw() = lim k N !" $ ##wNwppk =1 !!!FFIk kk kkei = k " = zFIli= () li !!!wIwlij li lij l-th layer $$FF$ "#kk ith - node = kk% wz= z kk&'* lij(1) l!! q (1) l j $$Iwli lij()q $ I li kk Iwzli= " liq(1) l! q q ##FF kk==!!kkz ; k ##wIli(1) l" j li lij li ... w k lij k z(1)1l! k z z(1)maxlq! (1)2l! ... k ... z(1)lj! k We have to calculate !li! =1 1 m $F $$zFk "#**2 l: output layer: k *li= k '(%&%& ykk) z= ))2 y kk z 2 k kk3*+*+p lp i li $F 2 $$zli Izli $ li '(,-,- ! k ==k ./p=1 li k 4 $$$Fzk F l: not output layer: k* li== k F0 Ik $Ili kk k( li ) 2 $$zIli li $ z li k 52 !"µl+1 #F #I k * (1)lp+ FI$ k ==%&) kk( li ) '(%&p=1 ##Iz(1)l+ p li !"µl+1 #F # $% k kk& =+='(kk* )*wzFI(1)l+ p lr( li ) // -.'(pr=1 ##Iz(1)l+ p li +, "#µµll++11$F k %%kk k ==&'k **()wFI(1)l+++ pi( li) ! (1)(1) l p wFI l pi li ** ()&'pp==11$I(1)lp+ N %F 1 kk k = !!liz(1) l" j where li is determined iteratively (see above) lim & %wNp N #$ k =1 N algorithm: weight change is new old1 k k wwlij= lij# !"% li z(1) l# j !F N k =1 proportional to (gradient): !wlij $ 0< ! ~small ( learning rate) Convergence)of)Backpropagation 1. Standard)backpropagation)reduces)error)F 9 BUT:)no)guarantee)of)convergence)to)global) minimum 9 Possible)problems: 9 Local)minimum 9 Very)slow)decrease 2. Theorem)on)the)approximation)by)39layer)NN Any)square)integrable)function)can)be)approximated) with)arbitrary)accuracy)by)a)39layer)backpropagation) ANN. 9 BUT:)no)guarantee)that)BP)(delta9rule)or)other)) gives)the)optimum)approximation Theorem'on'opt.'approx.'by'backpropagation D.W.Ruck,)S.K.Rogers,…)IEEE)Trans.)Neur.)Netw.,Vol.)1.pp.296A298,) 1990. Two'classes: p(x):=p(x|w1)P(w1)'+'p(x|w2)P(w2) p(x) @probability'distribution'of'feature'vectors'x''''''''''''''''''''p(x|wi)@ conditional'probability'density'f.'of'class'wi P(wi) @’a@priori’' probability'of'class'wi,'i=1,2.'''''''''''''P(wi|x) @’a@posteriori’' probability'of'class'x'to'belong'to'class'I Bayes'Rule: p(x|wi)P(wi)'='P(wi|x)p(x) Bayes'Discriminant:'''''P(w1|x)'– P(w2|x)'>'0 !select'class'1 THEOREM'(approximation'by'BPNN) An'optimally'selected'BP'NN'approximates'the'Bayesian'(maximum)' discriminant'function. NOTE:'the'actual'approximation'depends'on'the'structure'of'the' network,'class'conditional'probabilities'etc. Local&quadratic&approximation Taylor&expansion&of&error&function&w.r.t.&weights 1st and&2nd order:&gradient&and&Hessian&(H): Gradient&of&the&error: Modifications+of+Standard+Backpropagation 1. Optimum+choice+of+learning+rate: Initialization+of+weights Adaptive+learning+rate Randomization 2. Adding+a+momentum+term w k+1 = w k +#(1! µ)" k xk + µ(w k ! w k-1) 3. Regularization+term+to+SSE e.g.,+sum+of+weights+! pruning Forgetting+rate+! pruning * 2 I = !(yi " yi ) + ε'!| w ij |, i i, j Basic&NN&Architectures Feed$forward*NN Directed&graph&in&which&a&path&never&visits&the&same&node&twice Relatively&simple&behavior Example:&MLP&for&classification,&pattern&recognition Feedback*or*Recurrent*NNs Contains&loops&of&directed&edges&going&forward&and&also&backward Complicated&oscillations&might&occur Example:&Hopfield&NN,&Elman&NN&for&speech&recogn. Random*NNs More&realistic,&very&complex.
Details
-
File Typepdf
-
Upload Time-
-
Content LanguagesEnglish
-
Upload UserAnonymous/Not logged-in
-
File Pages24 Page
-
File Size-