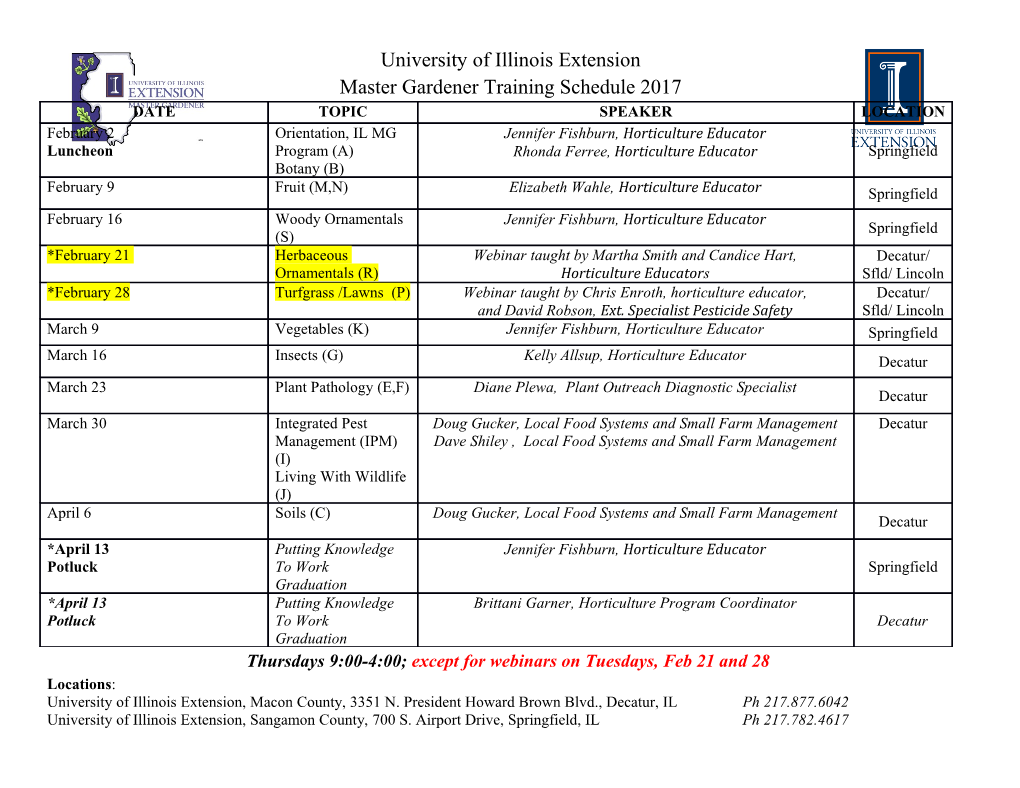
Finding Equilibria in Large Sequential Games of Imperfect Information∗ Andrew Gilpin Tuomas Sandholm Computer Science Department Computer Science Department Carnegie Mellon University Carnegie Mellon University Pittsburgh, PA, USA Pittsburgh, PA, USA [email protected] [email protected] ABSTRACT agent(s). Consequently, the optimal action of one agent can Finding an equilibrium of an extensive form game of imper- depend on the others. Game theory provides a normative fect information is a fundamental problem in computational framework for analyzing such strategic situations. In partic- game theory, but current techniques do not scale to large ular, it provides solution concepts that define what rational games. To address this, we introduce the ordered game iso- behavior is in such settings. The most famous and impor- morphism and the related ordered game isomorphic abstrac- tant solution concept is that of Nash equilibrium [36]. It is tion transformation. For a multi-player sequential game of a strategy profile (one strategy for each agent) in which no imperfect information with observable actions and an or- agent has incentive to deviate to a different strategy. How- dered signal space, we prove that any Nash equilibrium in ever, for the concept to be operational, we need algorithmic an abstracted smaller game, obtained by one or more appli- techniques for finding an equilibrium. cations of the transformation, can be easily converted into Games can be classified as either games of perfect informa- a Nash equilibrium in the original game. We present an al- tion or imperfect information. Chess and Go are examples gorithm, GameShrink, for abstracting the game using our of the former, and, until recently, most game playing work isomorphism exhaustively. Its complexity is O˜(n2), where n has been on games of this type. To compute an optimal is the number of nodes in a structure we call the signal tree. strategy in a perfect information game, an agent traverses It is no larger than the game tree, and on nontrivial games the game tree and evaluates individual nodes. If the agent it is drastically smaller, so GameShrink has time and space is able to traverse the entire game tree, she simply computes an optimal strategy from the bottom-up, using the principle complexity sublinear in the size of the game tree. Using 1 GameShrink, we find an equilibrium to a poker game with of backward induction. In computer science terms, this is 3.1 billion nodes—over four orders of magnitude more than done using minimax search (often in conjunction with α- in the largest poker game solved previously. We discuss β-pruning to reduce the search tree size and thus enhance speed). Minimax search runs in linear time in the size of the several electronic commerce applications for GameShrink. 2 To address even larger games, we introduce approximation game tree. methods that do not preserve equilibrium, but nevertheless The differentiating feature of games of imperfect informa- yield (ex post) provably close-to-optimal strategies. tion, such as poker, is that they are not fully observable: when it is an agent’s turn to move, she does not have access Categories and Subject Descriptors: I.2 [Artificial In- to all of the information about the world. In such games, telligence], F. [Theory of Computation], J.4 [Social and Be- the decision of what to do at a point in time cannot gener- havioral Sciences]: Economics. ally be optimally made without considering decisions at all General Terms: Algorithms, Economics, Theory. other points in time (including ones on other paths of play) Keywords: game theory, sequential games of imperfect in- because those other decisions affect the probabilities of be- formation, automated abstraction, equilibrium finding, com- ing at different states at the current point in time. Thus puter poker. the algorithms for perfect information games do not solve games of imperfect information. 1. INTRODUCTION For sequential games with imperfect information, one could In environments with more than one agent, an agent’s try to find an equilibrium using the normal (matrix) form, outcome is generally affected by the actions of the other where every contingency plan of the agent is a pure strategy for the agent.3 Unfortunately (even if equivalent strategies ∗This material is based upon work supported by the Na- tional Science Foundation under ITR grants IIS-0121678 and IIS-0427858, and a Sloan Fellowship. 1This actually yields a solution that satisfies not only the Nash equilibrium solution concept, but a stronger solution Permission to make digital or hard copies of all or part of this work for concept called subgame perfect Nash equilibrium [45]. personal or classroom use is granted without fee provided that copies are 2 not made or distributed for profit or commercial advantage and that copies This type of algorithm still does not scale to huge bear this notice and the full citation on the first page. To copy otherwise, to trees (such as in chess or Go), but effective game-playing republish, to post on servers or to redistribute to lists, requires prior specific agents can be developed even then by evaluating interme- permission and/or a fee. diate nodes using a heuristic evaluation and then treating those nodes as leaves. EC'06, June 11–15, 2006, Ann Arbor, Michigan, USA. 3 Copyright 2006 ACM 1-59593-236-4/06/0006 ...$5.00. An ²-equilibrium in a normal form game with any are replaced by a single strategy [27]) this representation is Theorem 2 Let Γ be a game with ordered sig- generally exponential in the size of the game tree [52]. nals, and let F be an information filter for Γ. Let By observing that one needs to consider only sequences of F 0 be an information filter constructed from F by moves rather than pure strategies [41, 46, 22, 52], one arrives one application of the ordered game isomorphic at a more compact representation, the sequence form, which abstraction transformation, and let σ0 be a Nash is linear in the size of the game tree.4 For 2-player games, equilibrium strategy profile of the induced game 0 there is a polynomial-sized (in the size of the game tree) ΓF 0 (i.e., the game Γ using the filter F ). If σ is linear programming formulation (linear complementarity in constructed by using the corresponding strategies 0 the non-zero-sum case) based on the sequence form such that of σ , then σ is a Nash equilibrium of ΓF . strategies for players 1 and 2 correspond to primal and dual The proof of the theorem uses an equivalent character- variables. Thus, the equilibria of reasonable-sized 2-player ization of Nash equilibria: σ is a Nash equilibrium if and games can be computed using this method [52, 24, 25].5 only if there exist beliefs µ (players’ beliefs about unknown However, this approach still yields enormous (unsolvable) information) at all points of the game reachable by σ such optimization problems for many real-world games, such as that σ is sequentially rational (i.e., a best response) given µ, poker. where µ is updated using Bayes’ rule. We can then use the 1.1 Our approach fact that σ0 is a Nash equilibrium to show that σ is a Nash equilibrium considering only local properties of the game. In this paper, we take a different approach to tackling We also give an algorithm, GameShrink, for abstracting the difficult problem of equilibrium computation. Instead the game using our isomorphism exhaustively (Section 4). of developing an equilibrium-finding method per se, we in- Its complexity is O˜(n2), where n is the number of nodes in stead develop a methodology for automatically abstracting a structure we call the signal tree. It is no larger than the games in such a way that any equilibrium in the smaller game tree, and on nontrivial games it is drastically smaller, (abstracted) game corresponds directly to an equilibrium in so GameShrink has time and space complexity sublinear in the original game. Thus, by computing an equilibrium in the size of the game tree. We present several algorithmic and the smaller game (using any available equilibrium-finding data structure related speed improvements (Section 4.1), algorithm), we are able to construct an equilibrium in the and we demonstrate how a simple modification to our al- original game. The motivation is that an equilibrium for gorithm yields an approximation algorithm (Section 5). the smaller game can be computed drastically faster than for the original game. 1.2 Electronic commerce applications To this end, we introduce games with ordered signals (Sec- Sequential games of imperfect information are ubiquitous, tion 2), a broad class of games that has enough structure for example in negotiation and in auctions. Often aspects which we can exploit for abstraction purposes. Instead of of a player’s knowledge are not pertinent for deciding what operating directly on the game tree (something we found action the player should take at a given point in the game. to be technically challenging), we instead introduce the use On the trivial end, some aspects of a player’s knowledge are of information filters (Section 2.1), which coarsen the infor- never pertinent (e.g., whether it is raining or not has no mation each player receives. They are used in our analysis bearing on the bidding strategy in an art auction), and such and abstraction algorithm. By operating only in the space aspects can be completely left out of the model specification. of filters, we are able to keep the strategic structure of the However, some aspects can be pertinent in certain states game intact, while abstracting out details of the game in a of the game while they are not pertinent in other states, way that is lossless from the perspective of equilibrium find- and thus cannot be left out of the model completely.
Details
-
File Typepdf
-
Upload Time-
-
Content LanguagesEnglish
-
Upload UserAnonymous/Not logged-in
-
File Pages10 Page
-
File Size-