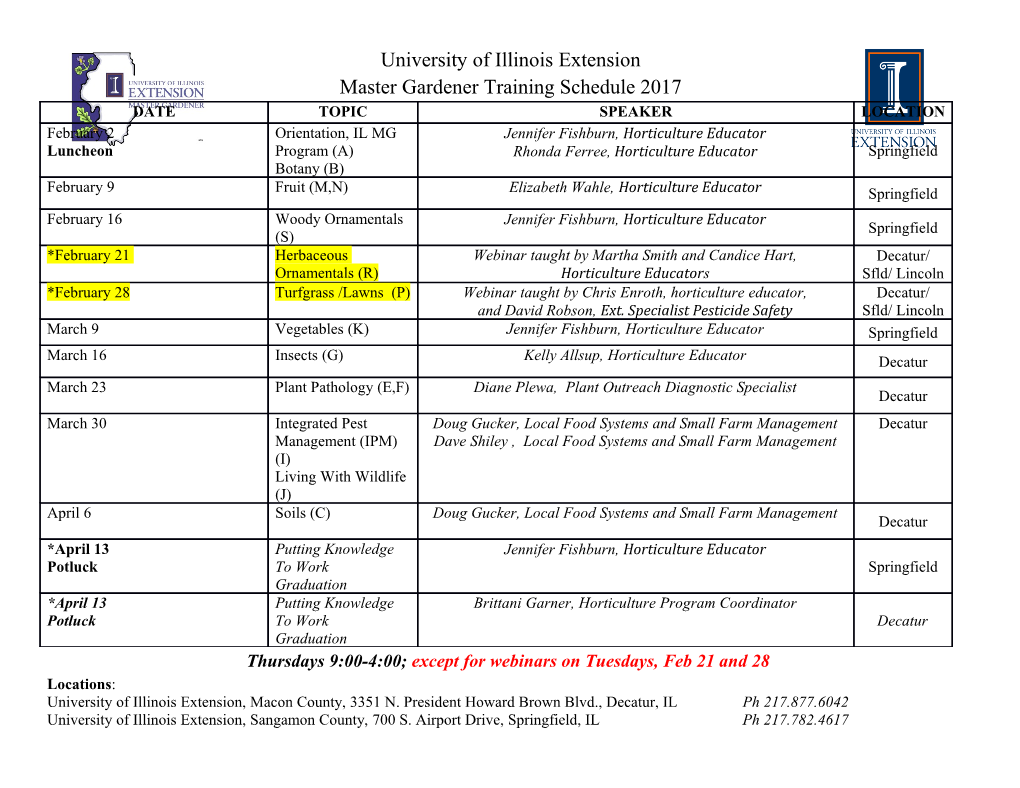
Summer, 2001 Volume 36 Number 2 The Psychologist A publication of the Society for General Psychology Division One of the American Psychological Association Officers of the Society President Lyle Bourne, Jr. Department of Psychology University of Colorado Boulder, CO 80302 Past–President Lewis P. Lipsitt President–Elect Linda Bartoshuk Another opportunity to comment on the passing of time and what Secretary Michael Wertheimer is uppermost. Another half year Department of Psychology is upon us. Have you ever no- University of Colorado Boulder, CO 80302 ticed how frequently dental ap- pointments occur these days? At Treasurer any rate we have had an election Lee H. Matthews and the results are in. Bruce Members-at-Large Overmeir has been chosen as C. Alan Boneau Morton Ann Gernsbacher our next President-Elect while Lynn Hasher Susan Mineka and Bonnie Duncan Luce Strickland were elected to be- Michael Wertheimer Wendy Williams come Members-at-Large of the Executive Committee. Welcome Representative to APA Council Gregory A. Kimble to all. This is the Convention Issue so-called with the Editor: The General Psychologist Society’s upcoming Convention C. Alan Boneau 6518 Ridge Drive program available as a tearout Bethesda, MD 20816 on the last page of this issue. In [email protected] addition we have a message Historian from current Society President Donald Dewsbury Lyle Bourne. Also don’t forget to Editor: Review of General sign up for the Society’s listserv Psychology (see page 45). See you at the Peter Salovey Department of Psychology Convention and Business Meet- Yale University ing. New Haven, CT 06520-8205 [email protected] Summer, 2001, Volume 36 Number 2 The Psychologist Contents Martindale: Aesthetic Preference. 25 Bornstein: Questionnaires. 36 Eglash: Maier’s Fixation Experiment. 41 Society Business Message from President Bourne . 44 Letters to the Editor . .45 Nominations for Fellows . .46 Proposed Bylaw Amendments . 47 Convention Program . .51 The Psychologist HOW DOES THE BRAIN COMPUTE AESTHETIC PREFERENCE? Colin Martindale nodes represent basic features. There is no grandmother University of Maine node; rather one’s grandmother is represented by the nodes coding her features. Distributed models often yield better results but very quickly get very complicated. I have A connectionist or neural-network theory of beauty used localist terminology for purposes of clarity. and aesthetic preference is presented. It is not pos- sible to specify a list of features shared by all things 2. A state of activation. If some nodes are activated that are considered to be beautiful. However, I ar- enough, we are conscious of whatever they code. (Many gue, such things induce similar patterns of activation nodes, such as those controlling motor behavior, operate in the brain. Something is considered to be beautiful outside the realm of consciousness.) The one or two most to the extent that activation is maximized and inhibi- activated nodes correspond to whatever is in the focus of tion of activated nodes is minimized. This simple attention. Less activated nodes are in the fringe of aware- postulate can account for a variety of seemingly ness. Nodes differ in strength—that is, how strongly acti- unrelated aesthetic effects. This is demonstrated for vated they can become (Martindale, 1981, 1991). a sample of 60 such effects. Given that successful 3. A pattern of connections among the nodes. These cognition can be explained by the same postulate, connections can be either excitatory or inhibitory. They the laws of cognition and the laws of aesthetics are comprise our long-term memory and the largely innate isomorphic. Examples showing this are given. The connections involved in sensation and perception. act of creation, a case of extremely successful cog- nition, is thus isomorphic with the perception of 4. Input and output rules concerning how a node adds up something of great beauty. its inputs and how outputs relate to inputs and current Baumgarten (1750) coined the term ‘aesthetics’. He argued activation. The best rule is that activation of a node is a that aesthetics is the “science of sensory cognition.” Good sigmoidal function of its inputs. For example, as excitatory sensory cognition leads to a sense of beauty, whereas imper- input increases, activation of a node increases in a sigmoi- fect sensory cognition leads to a feeling of ugliness or defor- dal fashion that reaches an asymptotic level. This is the mity. Subsequent usage of the term aesthetics has deviated way neurons work. from the way Baumgarten used it. It should not have, as 5. Learning rules. A variety of learning rules have been Baumgarten was closer to the truth than many later aesthetic proposed. Many are variants of Hebb’s (1949) idea that if theorists. It turns out that the laws of aesthetics and of cognition two nodes are simultaneously activated, the strength of are largely isomorphic. Fechner (1876) guessed this might be the positive connection between them is increased. This the case. In founding psychological aesthetics, he set forth a is too simple. At the very least, we have to add a rule that number of principles. He was explicit that most are principles the strength of the inhibitory connection between two of general psychology rather than of aesthetics per se. nodes is increased if one is activated and the other is Cognition and neural networks inhibited. 6. An environment for the network. I have argued that the Cognitive theorists have increasingly turned toward a brain network should be partitioned into modules devoted to metaphor: the mind works like the brain. Because cognition quite specific tasks (Martindale, 1981, 1991). The brain occurs in the brain, this is certainly reasonable. Connectionist involves extreme division of labor. For example, percep- or neural-network theories all postulate nodes and connec- tion of a colored moving form involves activation of neu- tions amongst these nodes. Nodes work like neurons but are rons in quite discrete areas devoted to location, form, simpler, and connections work like axons and dendrites but color, and motion (Treisman, 1992). We need to postulate are again simpler. The reason for this simplification is that we a number of sensory and perceptual modules. We also do not know enough about the brain to attempt a neuron-by- need modules for semantic memory, episodic memory, neuron explanation of cognition. and an action module that initiates response to a stimulus. To build a neural network, we need several components I have also argued that each module is organized into (Rumelhart, Hinton & McClelland, 1986): several layers, with vertical connections being excitatory and lateral inhibition operating on each layer. Vertical 1. A set of nodes. These are similar to neurons but not as connections are usually bidirectional as are lateral inhibi- complicated. In localist models, nodes represent some- tory connections, with amount of inhibition being propor- thing, such as a one’s grandmother. In distributed models, tional to distance between nodes. This is the way the brain Summer, 2001 Volume 36:2 Pages 25 - 35 26 is organized. On any layer of a module I argue that nodes 2. Lightness of colors holding constant hue and satura- are arranged in terms of similarity. The more similar two tion: Lightness is related to preference in a positive mono- things are, the closer the nodes coding them are. Such an tonic fashion (Guilford, 1939). arrangement allows us to explain a variety of cognitive phenomena. 3. Hue preference: There is a fairly universal order of hue preference (Eysenck, 1941). Most people like blue or red Neural network theories ultimately have one explanation best, than green, than orange. Yellow is most peoples’ for everything: how activated the nodes involved in a least favorite color. If we consider spectral colors, this phenomenon are. Why do we perceive something? Be- makes preference a U-shaped function of hue. However, cause a stimulus activated the relevant nodes. Why are we hues differ in their maximal saturation. Maximal satura- attending to this rather than that? Because the nodes tion is also a U-shaped function of hue. If we plot hue coding this are more activated than the nodes coding that. preference as a function of saturation, we obtain a posi- Why do we remember something? Because the nodes tive monotonic function. coding it are sufficiently activated. Why do we forget something? Because the nodes coding the to-be-remem- 4. Color typicality: The more typical of its hue a color is bered item are not activated enough. Desirable cognitive judged to be, the more it is liked (Martindale & Moore, outcomes are explained in terms of maximizing activation 1988). However, typicality is almost perfectly correlated and minimizing inhibition. Undesirable outcomes such as with saturation, so we can explain the typicality effect in forgetting or being confused are attributed to too much terms of saturation. inhibition and not enough activation. 5. Word frequency: Zajonc (1968) showed that there is a We can explain beauty and aesthetic pleasure in the same positive relationship between the frequency with which a way (Martindale, 1984a, 1988): These phenomena result word occurs and preference for the thing denoted by the when activation is maximized and inhibition of activated word. There is a variety of experimental evidence that can nodes is minimized. On any layer of a module, lateral best be explained by postulating that more frequent words inhibition normalizes or keeps activation relatively con- are coded by stronger nodes with lower thresholds than stant. Given this, the crucial factor is often how the acti- are less frequent words. vated nodes are distributed on each layer. The laws of Strength Effects Involving Distribution of Activation cognition and of aesthetic pleasure are isomorphic. We could repeat virtually any experiment in cognitive psy- A variety of aesthetic effects involve maximizing activa- chology for which it were reasonable to ask for preference tion and minimizing inhibition of activated nodes by maxi- judgments and get a pattern of results similar to that mizing the distance among activated nodes.
Details
-
File Typepdf
-
Upload Time-
-
Content LanguagesEnglish
-
Upload UserAnonymous/Not logged-in
-
File Pages31 Page
-
File Size-