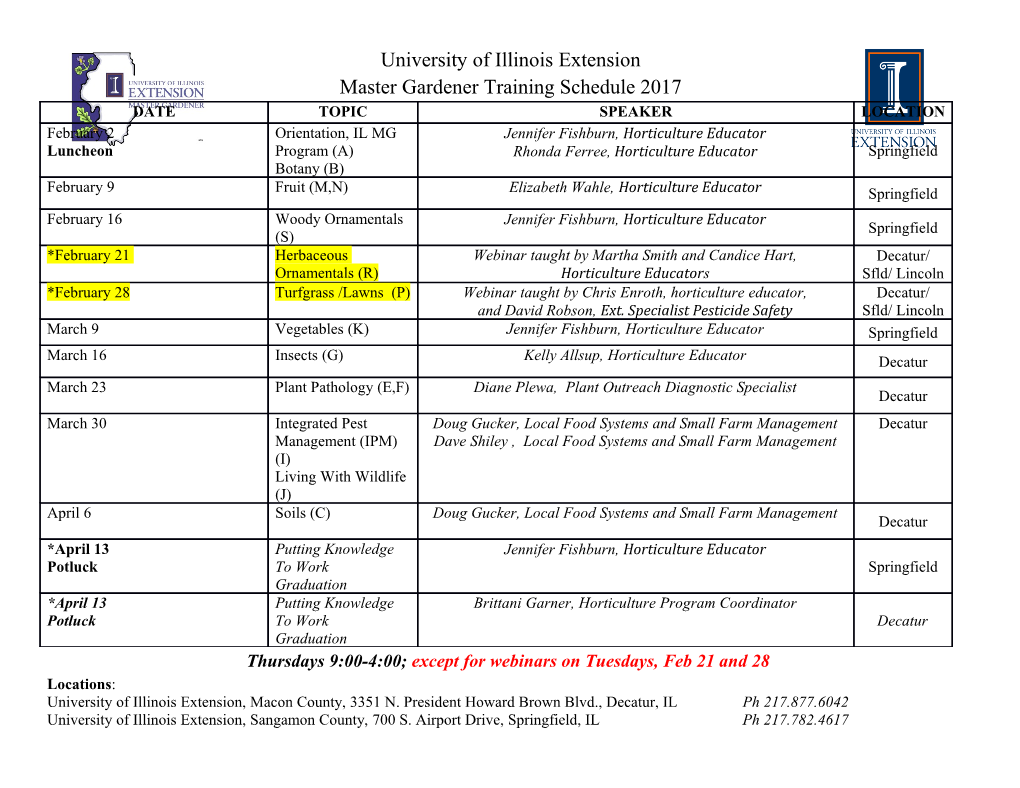
Unit 9: Confounding and Fractional Factorial Designs STA 643: Advanced Experimental Design Derek S. Young 1 Learning Objectives I Understand what it means for a treatment to be confounded with blocks I Know how generalized interactions are used in confounding I Know how to construct and analyze incomplete block designs for 2k and 3k factorial designs I Become familiar with half-fraction and quarter-fraction designs I Understand how we use aliasing and design generators for fractional factorial designs I Understand the resolution of a design I Understand how to use the Plackett-Burman designs for the purpose of screening large number of factors 2 Outline of Topics 1 Confounding 2 Fractional Factorial Designs 3 Design Resolution 3 Outline of Topics 1 Confounding 2 Fractional Factorial Designs 3 Design Resolution 4 Leveraging Factorial Treatment Designs I Recall that we designed and analyzed experiments that involved k factors, each at two levels { i.e., 2k factorial designs. k I A major advantage of 2 factorial designs is that they help determine whether the factors act independently or if they interact with one another as they affect the EUs. k k I By far, 2 and 3 (i.e., three levels of each factor) factorial designs are the most common, but as the number of factors increases, then the number of treatment combinations rapidly increases. I Thus, we turn to incomplete block designs to help control experimental error, while simultaneously accomplishing what we hope to answer with a factorial design. I We can leverage the factorial arrangement to provide effective incomplete block designs and subsequent analyses. 5 Example: Popcorn Experiment If you have ever made a bag of microwaveable popcorn, you probably noticed that there are always unpopped kernels at the bottom of the bag. An experiment involving (approximately) 3.5 ounce bags of popcorn was designed. Three factors are studied: brand of popcorn (a cheap brand and a costly brand), amount of time in the microwave (4 minutes and 6 minutes), and percent power of the microwave (75% and 100%). Thus, this is a 23 factorial design. The weight of the remaining unpopped kernels (in ounces) after popping is recorded. See the table below. Note that the observation in the second row is 3.5 oz. For this bag, virtually none of the popcorn popped. The first column is the "run order"; i.e., the order in which the treatments were ran. Run Order Brand (A) Time (B) Power (C) Kernels (Y) 8 Cheap 4 75 3.1 1 Costly 4 75 3.5 2 Cheap 6 75 1.6 4 Costly 6 75 1.2 3 Cheap 4 100 0.7 5 Costly 4 100 0.7 7 Cheap 6 100 0.5 6 Costly 6 100 0.3 6 Example: Popcorn Experiment I Below is the design matrix for the full model with the two-way interactions and three-way interaction, reported in standard order. I The low levels of each factor are denoted by a \-" { these are the levels of \Cheap", \4", and \75". I The high levels of each factor are denoted by a \+" { these are the levels of \Costly", \6", and \100". Std. Order I A B C AB AC BC ABC Y 1 + - - - + + + - y111 = 3:1 2 + + - - - - + + y211 = 3:5 3 + - + - - + - + y121 = 1:6 4 + + + - + - - - y221 = 1:2 5 + - - + + - - + y112 = 0:7 6 + + - + - + - - y212 = 0:7 7 + - + + - - + - y122 = 0:5 8 + + + + + + + + y222 = 0:3 7 Example: Popcorn Experiment A few notes about the design matrix on the previous slide: I The use of \+" and \-" symbols is so that we can define contrasts of coefficients of +1 and -1 for each of the treatment combinations. I The column \I" contains all \+", which is used to estimate the grand mean with a divisor of 23 (or more generally, 2k). I We say that the matrix is in standard order because the pattern of "+"s and "-"s in the columns (factors) have been arranged a certain way. Namely, column A has successive pairs of \-" and \+" signs, column B has pairs of \-" signs followed by pairs of \+" signs, and column C has four \-" signs followed by four \+" signs. In general, the kth column has 2k−1 of the \-" signs followed by an equal number of \+" signs. Thus, you can construct a design matrix in standard order for an arbitrary 2k factorial design. I The coefficients for any interaction is the product of the columns of coefficients that comprise that interaction. For example, ABC in row 7 is found by (-1)(+1)(+1)=(-1), or simply, a \-" sign. 8 Example: Popcorn Experiment Below are the treatment means plots. We see that there is a potential interaction due to the magnitude of the responses. Recall that no interaction is present if lines at two levels of a treatment are parallel to each other. Power = 75% Power = 100% 1 1 1 3.5 0.7 Time Time 1 4 1 4 1 2 6 2 6 3.0 0.6 2.5 2 0.5 2.0 Amount of Kernels (oz) Amount of Kernels (oz) 0.4 2 1.5 2 2 0.3 cheap costly cheap costly Brand Brand 9 Example: Popcorn Experiment B C Simple Effect of Cheap Brand to Costly Brand (A) 4 75 µ^211 − µ^111 = 3:5 − 3:1 = 0:4 6 75 µ^221 − µ^121 = 1:2 − 1:6 = −0:4 4 100 µ^212 − µ^112 = 0:7 − 0:7 = 0:0 6 100 µ^222 − µ^122 = 0:3 − 0:5 = −0:2 A C Simple Effect of 4 Minutes to 6 Minutes (B) Cheap 75 µ^121 − µ^111 = 1:6 − 3:1 = −1:5 Costly 75 µ^221 − µ^211 = 1:2 − 3:5 = −2:3 Cheap 100 µ^122 − µ^112 = 0:5 − 0:7 = −0:2 Costly 100 µ^222 − µ^212 = 0:3 − 0:7 = −0:4 A B Simple Effect of 75% Power to 100% Power (C) Cheap 4 µ^112 − µ^111 = 0:7 − 3:1 = −2:4 Costly 4 µ^212 − µ^211 = 0:7 − 3:5 = −2:8 Cheap 6 µ^122 − µ^121 = 0:5 − 1:6 = −1:1 Costly 6 µ^222 − µ^221 = 0:3 − 1:2 = −0:9 Note: µ^ijk = yijk 10 Example: Popcorn Experiment 1 Grand Mean: y¯··· = 8 y··· = 1:45 Main Effects: Factor A: = Factor B: = Factor C: = Two-Factor Interactions: 1 1 AB: (y111 + y112 + y221 + y222) − (y211 + y212 + y121 + y122) = 1:325 − 1:575 = −0:250 4 4 1 1 AC: (y111 + y121 + y212 + y222) − (y211 + y221 + y112 + y122) = 1:425 − 1:475 = −0:050 4 4 1 1 BC: (y111 + y211 + y122 + y222) − (y121 + y221 + y112 + y212) = 1:850 − 1:050 = 0:800 4 4 Three-Factor Interaction: 1 1 ABC: ((y222−y122)−(y212−y112))− ((y221−y121)−(y211−y111)) = −0:050−(−0:200) = 0:150 4 4 11 Example: Popcorn Experiment Analysis of Variance Table Response: bullets Df Sum Sq Mean Sq F value Pr(>F) brand 1 0.005 0.005 0.1111 0.79517 time 1 2.420 2.420 53.7778 0.08628 . power 1 6.480 6.480 144.0000 0.05293 . brand:time 1 0.125 0.125 2.7778 0.34404 brand:power 1 0.005 0.005 0.1111 0.79517 time:power 1 1.280 1.280 28.4444 0.11800 Residuals 1 0.045 0.045 --- Signif. codes: 0 *** 0.001 ** 0.01 * 0.05 . 0.1 1 Since this is a 23 factorial design without replicates, we use the three-way interaction as our estimate of error. Moreover, the three-way interaction effect appears minor given that the effect calculated on the previous slide is only 0.150, which is smaller compared to most of the other calculated effects. In the above ANOVA table, it appears that all of the two-way interactions are not significant at the 0.05 level. While we could systematically remove each interaction based on the highest p-value, we will simply remove all two-way interactions from the analysis and focus on the main effects. 12 Example: Popcorn Experiment Analysis of Variance Table Response: bullets Df Sum Sq Mean Sq F value Pr(>F) brand 1 0.005 0.0050 0.0137 0.91232 time 1 2.420 2.4200 6.6529 0.06137 . power 1 6.480 6.4800 17.8144 0.01347 * Residuals 4 1.455 0.3637 --- Signif. codes: 0 *** 0.001 ** 0.01 * 0.05 . 0.1 1 In the above ANOVA table, it appears that brand is not statistically significant give it has such a large p-value. This, again, is not unexpected given that the estimated main effect of -0.050 is small. So, we remove the effect due to brand. 13 Example: Popcorn Experiment Analysis of Variance Table Response: bullets Df Sum Sq Mean Sq F value Pr(>F) time 1 2.42 2.420 8.2877 0.034635 * power 1 6.48 6.480 22.1918 0.005286 ** Residuals 5 1.46 0.292 --- Signif. codes: 0 *** 0.001 ** 0.01 * 0.05 . 0.1 1 In the above ANOVA table, we are left with both time and power as significant effects on the amount of kernels left after popping. In all of these ANOVA tables, the F -tests were tested against the MSE. 14 Contrasts I In general, the contrast among treatment means (main effect or interaction) is X lAB··· = cty¯t; t where t is the index over the different treatments and ct = ±1 are the coefficients for the contrasts, which are determined by the \+"s and \-"s in the column of the design matrix for treatment AB ··· .
Details
-
File Typepdf
-
Upload Time-
-
Content LanguagesEnglish
-
Upload UserAnonymous/Not logged-in
-
File Pages60 Page
-
File Size-