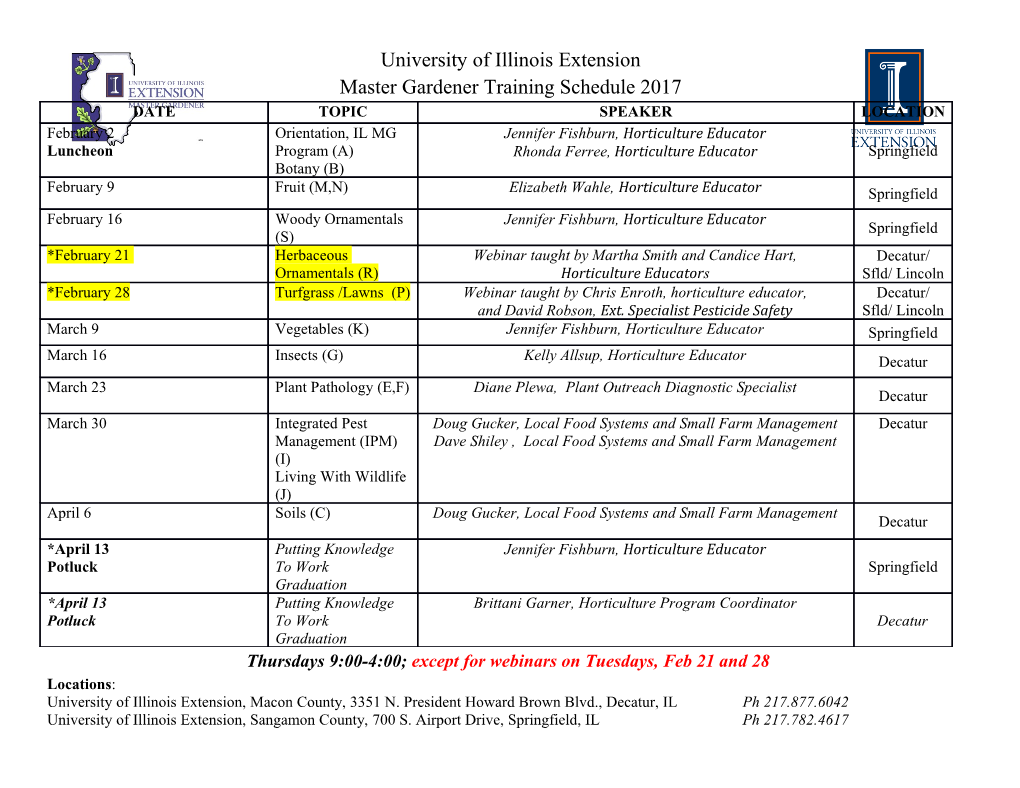
water Article Dynamic Monitoring of Surface Water Area during 1989–2019 in the Hetao Plain Using Landsat Data in Google Earth Engine Ruimeng Wang 1, Haoming Xia 1,2,* , Yaochen Qin 1,2 , Wenhui Niu 1, Li Pan 1, Rumeng Li 1, Xiaoyang Zhao 1, Xiqing Bian 1 and Pinde Fu 1,2 1 College of Environment and Planning, Key Laboratory of Geospatial Technology for the Middle and Lower Yellow River Regions, China Meteorological Administration Henan Key Laboratory of Agrometeorological · Support and Applied Technique, Henan University, Kaifeng 475001, China; [email protected] (R.W.); [email protected] (Y.Q.); [email protected] (W.N.); [email protected] (L.P.); [email protected] (R.L.); [email protected] (X.Z.); [email protected] (X.B.); [email protected] (P.F.) 2 Key Research Institute of Yellow River Civilization and Sustainable Development & Collaborative Innovation Center on Yellow River Civilization of Henan Province, Henan University, Kaifeng 475001, China * Correspondence: [email protected]; Tel.: +86-371-2388-1858 Received: 11 September 2020; Accepted: 23 October 2020; Published: 27 October 2020 Abstract: The spatio-temporal change of the surface water is very important to agricultural, economic, and social development in the Hetao Plain, as well as the structure and function of the ecosystem. To understand the long-term changes of the surface water area in the Hetao Plain, we used all available Landsat images (7534 scenes) and adopted the modified Normalized Difference Water Index (mNDWI), Enhanced Vegetation Index (EVI), and Normalized Difference Vegetation Index (NDVI) to map the open-surface water from 1989 to 2019 in the Google Earth Engine (GEE) cloud platform. We further analyzed precipitation, temperature, and irrigated area, revealing the impact of climate change and human activities on long-term surface water changes. The results show the following. (1) In the last 31 years, the maximum, seasonal, and annual average water body area values in the Hetao Plain have exhibited a downward trend. Meanwhile, the number of maximum, seasonal, and permanent water bodies displayed a significant upward trend. (2) The variation of the surface water area in the Hetao Plain is mainly affected by the maximum water body area, while the variation of the water body number is mainly affected by the number of minimum water bodies. (3) Precipitation has statistically significant positive effects on the water body area and water body number, which has statistically significant negative effects with temperature and irrigation. The findings of this study can be used to help the policy-makers and farmers understand changing water resources and its driving mechanism and provide a reference for water resources management, agricultural irrigation, and ecological protection. Keywords: surface water; Yellow River Basin; Hetao Plain; Google Earth Engine; Landsat images; climate change 1. Introduction As an important part of the land–water cycle, surface water resources play an important role in promoting national economic development and maintaining the balance of terrestrial and aquatic ecosystems, agriculture, and the ecological environment [1]. In terms of global climate change, all kinds of water resources show obvious characteristics of temporal and spatial differentiation [2–5]. It is necessary to strengthen the dynamic monitoring and investigation of water resources, especially for arid and semi-arid areas. The Yellow River Basin is an important ecological barrier and economic Water 2020, 12, 3010; doi:10.3390/w12113010 www.mdpi.com/journal/water Water 2020, 12, 3010 2 of 21 area in China. Ecological protection and high-quality development of the Yellow River Basin have become national strategies [6]. The Hetao Plain, as one of the main areas of the Yellow River Basin, is an important commercial grain base in China. Irrigation directly consumed groundwater and surface water resources. In the process of the gradual development of agriculture, the contradiction between the supply and demand for water has become increasingly prominent. Monitoring the dynamic change of water resources in the Hetao Plain and improving the efficiency of water resources are very important to the economic development and agricultural production in the Hetao Plain. In the past hundred years, due to the intensification of human activities and global climate change, surface water resources have generally experienced problems such as a sharp decline in the water volume, deterioration of the water quality, and area shrinkage, which have changed the form of the surface water hydrological cycle and hydrological dynamics [2,3]. These problems have caused huge losses to the life and agricultural production of the residents in the Hetao Plain and greatly affected the development of the local economy. Problems such as the shrinkage of the surface water area, fragmentation of the water landscape, and deterioration of the ecological environment have aroused widespread concern from all walks of life [7]. The dynamic monitoring of surface water is a key issue in research on resources and environmental change [8,9]. The timely and accurate acquisition of surface water change information is an important foundation for water resource protection, utilization, and sustainable development. Remote sensing images data have the characteristics of wide coverage, high revisit frequency, rich information, and low cost; these with different time-space spectra are used in land use and land cover mapping [10]. The satellite data commonly used for water mapping and change monitoring include the Advanced Very High-Resolution Radiometer (AVHRR) [11], Moderate Resolution Imaging Spectroradiometer (MODIS) [12–15], Systeme Probatoire d’Observation de la Terre (SPOT) [16], Visible Infrared Imaging Radiometer Suite Visible Infrared Imaging Radiometer (VIIRS) [17], Landsat [4,8,15,18–25], and Sentinel [26,27], among others. Landsat images data are characterized by long time series (1972 to present), high spatial resolution, strong spectral consistency, and free access; Landsat images data represent the main data source for monitoring the dynamic changes of water bodies with long time series [21,28–30]. Previous studies have frequently selected a single remote sensing image in a year or in different years at the same time to represent the surface water area and interannual variation [8,9,31,32]. Due to the strong interannual and intra-annual variation characteristics of surface water [8,9], an annual or interannual single image, which was selected to characterize the area and time series of surface water, will lead to inaccuracies of the surface water body area and water body number [31,33–36]. Therefore, the use of all existing long-term sequence images is essential for accurately characterizing the interannual and intra-annual changes in surface water. Surface water extraction algorithms based on remote sensing data can be divided into two categories: machine learning algorithms and traditional algorithms [37]. One type is machine learning algorithms, including Support Vector Machine (SVM) [38], Random Forest (RF) [39], Decision Tree (DT) [40], Deep Learning (DL) [41], etc. Machine learning algorithms require professional knowledge on sample selection and algorithm training, and it is difficult to quickly map large regional scales such as the world and a country [42]. Traditional algorithms based on a combination of bands, including the single-band method and multi-band method, can be divided into the inter-spectral relation method and water index method [43]. The multi-band method can make full use of the spectral difference between water and non-water, and it is better than the single-band method in water extraction [44]. The water index method is a commonly used multi-band extraction method, which is combined with other remote sensing indexes to select the threshold of significant difference between a water body and a non-water body. It has high accuracy in water extraction. At the same time, this method has the advantages of rapid extraction, high accuracy, simplicity, robustness, and good repeatability when extracting large areas of water [9,25,34,42,45–48]. Since 2008, Petabyte-Scale Landsat images have been shared free of charge, making it possible for researchers to use all available Landsat images to better capture surface water change information. Water 2020, 12, 3010 3 of 21 At the same time, the ability of cloud computing has been significantly improved in the recent years,Water 2020 and, 12 it, x has FOR shown PEER REVIEW great application potential in large-scale land cover mapping. For example,3 of 22 the Google Earth Engine (GEE), with high-performance parallel computing capabilities, massive remote Google Earth Engine (GEE), with high-performance parallel computing capabilities, massive remote sensing, and geospatial data, is free to use [25,49]. It facilitates the use of all images archived on sensing, and geospatial data, is free to use [25,49]. It facilitates the use of all images archived on the the platform for global and national land cover remote sensing mapping and land cover change platform for global and national land cover remote sensing mapping and land cover change monitoring [10,34–36,50–54]. Based on the GEE platform, 7534 remote sensing images of Landsat monitoring [10,34–36,50–54]. Based on the GEE platform, 7534 remote sensing images of Landsat Thematic Mapper (TM), Landsat Enhanced Thematic Mapper (ETM+), and Landsat Operational Thematic Mapper (TM), Landsat Enhanced Thematic Mapper (ETM+), and Landsat Operational Land Land Imager (OLI) captured from 1989 to 2019 were obtained by JavaScript programming Imager (OLI) captured from 1989 to 2019 were obtained by JavaScript programming operation. The operation. The method of combining the modified Normalized Difference Water Index (mNDWI), method of combining the modified Normalized Difference Water Index (mNDWI), Enhanced Enhanced Vegetation Index (EVI), and Normalized Difference Vegetation Index (NDVI) was used Vegetation Index (EVI), and Normalized Difference Vegetation Index (NDVI) was used to extract the to extract the accuracy of surface water extraction.
Details
-
File Typepdf
-
Upload Time-
-
Content LanguagesEnglish
-
Upload UserAnonymous/Not logged-in
-
File Pages21 Page
-
File Size-