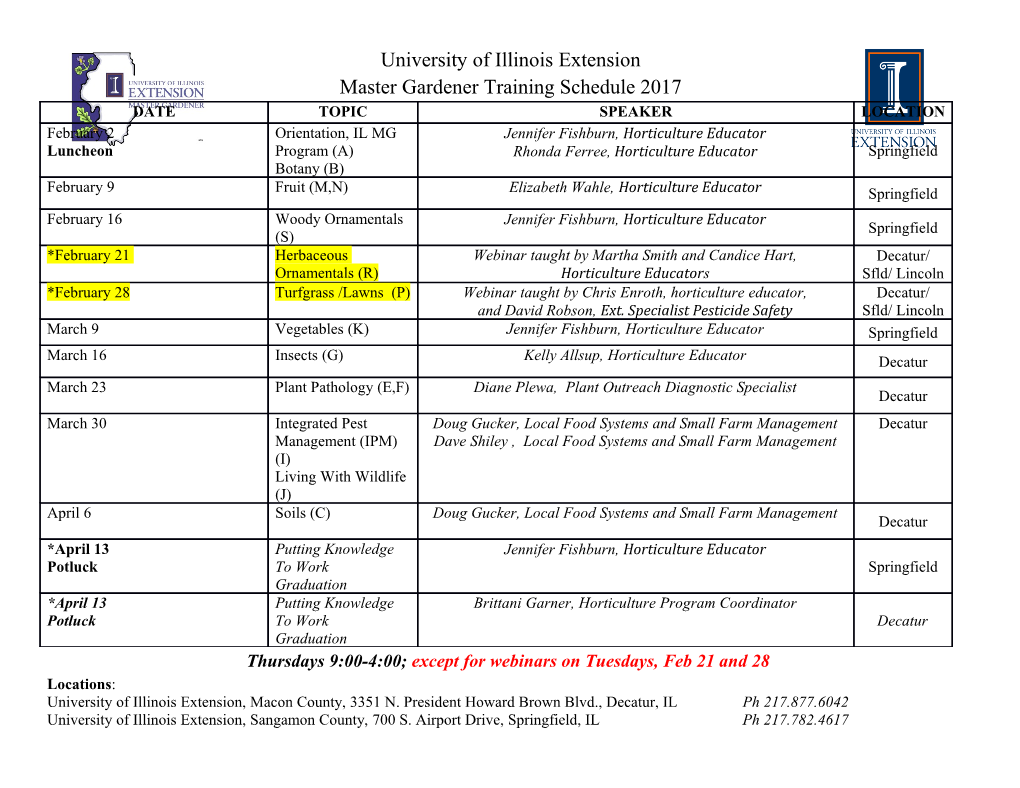
Quantitative genetic methods depending on the nature of the phenotypic trait Pierre de Villemereuil To cite this version: Pierre de Villemereuil. Quantitative genetic methods depending on the nature of the phenotypic trait. Annals of the New York Academy of Sciences, Wiley, 2018, 1422 (1), pp.29-47. 10.1111/nyas.13571. hal-03043413 HAL Id: hal-03043413 https://hal.archives-ouvertes.fr/hal-03043413 Submitted on 7 Dec 2020 HAL is a multi-disciplinary open access L’archive ouverte pluridisciplinaire HAL, est archive for the deposit and dissemination of sci- destinée au dépôt et à la diffusion de documents entific research documents, whether they are pub- scientifiques de niveau recherche, publiés ou non, lished or not. The documents may come from émanant des établissements d’enseignement et de teaching and research institutions in France or recherche français ou étrangers, des laboratoires abroad, or from public or private research centers. publics ou privés. Quantitative genetic methods depending on the nature ofthe phenotypic trait Pierre de Villemereuila aSchool of Biological Sciences, University of Auckland, Auckland (New Zealand) [email protected] Abstract Introduction: the infinitesimal model and its A consequence of the assumptions of the infinitesimal assumptions model, one of the most important theoretical foun- dations of quantitative genetics, is that phenotypic Quantitative genetics is a conceptual and methodolog- traits are predicted to be most often normally dis- ical framework allowing biologists to study the ge- tributed (so-called Gaussian traits). But phenotypic netics of complex phenotypic traits,1,2 i.e. traits in- traits, especially those interesting for evolutionary bi- fluenced by a large number of genes. A central as- ology, might be shaped according to very diverse dis- sumption of the quantitative genetics framework is tributions. In this review, I show how quantitative ge- that traits are normally distributed (hereafter called netics tools have been extended to account for a wider Gaussian traits), and when using several traits in a diversity of phenotypic traits using first the threshold multivariate framework, that their relationships are model, then more recently using generalised linear simple and their number of dimensions is manageable. mixed models. I explore the assumptions behind these In this review, we will describe how new methodolog- models and how they can be used to study the genet- ical development are overcoming these constraints, al- ics of non Gaussian complex traits. I also comment on lowing us to study traits with non-normal error distri- three recent methodological advances in quantitative bution (hereafter called non-Gaussian traits), complex genetics that widen our ability to study new kinds of conditional relationships between traits and high- traits: the use of “modular” hierarchical modelling to dimensionality. e.g. study survival in the context of capture-recapture The foundations of quantitative genetics were es- approaches for wild populations; the use of aster mod- 3 els to study a set of traits with conditional relation- tablished in 1918 by Fisher using what is now known ships (e.g. life-history traits); and finally, the study of as the infinitesimal model. Apart from settling the the- high-dimensional traits such as gene expression. oretical disagreement between Mendelians and bio- metricians about the universality of Mendel’s laws,4 Keywords: quantitative genetics, non Gaussian the infinitesimal model has been used for a century traits, heritability, threshold model, generalised linear with much success, allowing the advent of efficient an- mixed models imal and plant breeding, while also deepening our un- derstanding of evolutionary biology.5 This success is Corresponding author: Pierre de Villemereuil, E- explained not only by the simplicity of the model, but mail: [email protected] also by its robustness to various assumptions.4 This Short title: Quantitative genetics for non classical model assumes that a complex phenotypic trait is in- traits fluenced by an infinite number of loci, all of which have an infinitesimal effect. This generally results in Published in: The Year in Evolutionary Biology the assumption of a Gaussian distribution of the ad- 2017, Annals of the New York Academy of Sciences ditive genetic effects of individuals. The variance of DOI: 10.1111/nyas.13571 these effects is the additive genetic varianceVA, which Accepted version after peer-review. is a parameter of prime interest in quantitative genet- 1 ics. Indeed, the most common way to evaluate how a Expanding the model to more population can respond to selection is through the ra- tio between the additive genetic variance and the total kinds of phenotypic traits 2 phenotypic variance (VP), namely the heritability (h ): The threshold model 2 VA : h = (1) The first extension of the model towards non- VP Gaussian traits dates back to Wright,8 working on the variable number (three or four, hence a binary trait) To estimate VA in modern analyses, the statistical assumptions of the infinitesimal model are best imple- of digits in the guinea pigs. Wright refuted the idea mented using a linear mixed model (LMM), in which that the complex results observed regarding the in- a random effect (with an assumed Gaussian distribu- heritance of this apparently simple phenotypic trait tion) reflecting the variance of additive genetic origin could be explained by an interaction between 3 differ- is included.6,7 In its most simple form, this model can ent genes (the favoured hypothesis at the time) and be written as: proposed a model assuming instead a very large num- ber of genes involved (i.e. the infinitesimal model). But, as explained above, the infinitesimal model nat- ; z = µ + Za + e (2) urally leads to a Gaussian trait and certainly not to a binary one. Wright thus assumed that, because of where z is the vector of observed phenotypic data, µ the mathematical consequences of the infinitesimal is the population mean phenotypic value, Za is a ran- model, “something” (a latent trait, currently referred dom effect of additive genetic origin (e.g. sire/dam to as “liability”, possibly through its use in quantita- effect or relatedness-based effect) and e is the vector tive genetics of disease literature) was normally dis- of residuals. It is important to note that the assump- tributed but that the resulting phenotype was sepa- tion of the infinitesimal model implies that the addi- rated into two categories: values of liability below a tive genetic effects a (also called breeding values) are given threshold would produce, say, three digits while normally distributed following the matrix of related- values above this threshold would produce four dig- ness between individuals in the population (here de- its (see Fig. 1). This idea of applying a threshold toa noted A): Gaussian hypothetical trait can be traced back to even earlier work by Pearson.9 a ∼ N (0; AVA) : (3) Since the residuals e are almost always assumed to be This model became known as the “threshold 10 normally distributed as well (with a variance, called model” and has been increasingly used, especially for the study of Human disease genetics,11 because it residual variance, noted VR), it follows that the pheno- typic trait z is also normally distributed. allows to use the quantitative genetics tools on cate- gorical traits. It was expanded to a “multiple thresh- Commonly used statistical tools in quantitative ge- old model” to account for traits with more than two netics are thus particularly well fitted for the study of categories.12,13 The success of the threshold model has Gaussian traits (i.e. normally distributed, at least con- been such that it has been proposed as a catch-all ditionally on some fixed effects), but they are not a model for any non-Gaussian trait, through transfor- universal tools for any type of phenotypic trait. Cu- mation into a binary trait,14 and its use has been pro- riously, despite the fact that the infinitesimal model moted outside of the quantitative genetics field.15 This is a century old, quantitative genetics remains very success is also due to the fact that statistical imple- dependent on these assumptions of normality. How- mentations of the threshold model have been avail- ever, in the last decade, much effort has been put into able for a long time.10,13,16 developing methods that are compatible with the core assumptions of the infinitesimal model, but that can also accommodate a broader range of characteristics The fact that the observed phenotype is a threshold- for the phenotypic trait under study, especially about based transformation of a Gaussian liability begs the its distribution (but see e.g. the case of gene expres- question of “where” to calculate the additive genetic sion). variance and heritability of the trait: at the level of the 2 reshold Recently, this approach has been successfully used Liability within the animal model framework to study the heritability of binary traits such as fish life-history strategies (migrant/resident),20 bird cooperative be- haviour (helper/non-helper)21 or dispersal status (allo- /phylopatric).22 It has also been shown to be unbi- ased when used with the animal model in a simulation study (see “REMLc” in Ref. 23), though, to the best of 0 my knowledge, the sensitivity to extreme values of p has never been checked when using the animal model. This historical evolution of the threshold model has three consequences. First, the threshold approach is straightforward to implement in practice because one can circumvent explicitly implementing the threshold model by a simple transformation of the heritability estimate. Second, and as a corollary, complex exten- Phenotype 1 Phenotype 2 sions of the model (e.g. multivariate models) are lack- Phenotypic trait ing, although they would be useful for the evolution- ary biology community. This has led to the third con- Figure 1: Graphic representation of the threshold sequence: the threshold model was “absorbed” into model.
Details
-
File Typepdf
-
Upload Time-
-
Content LanguagesEnglish
-
Upload UserAnonymous/Not logged-in
-
File Pages21 Page
-
File Size-