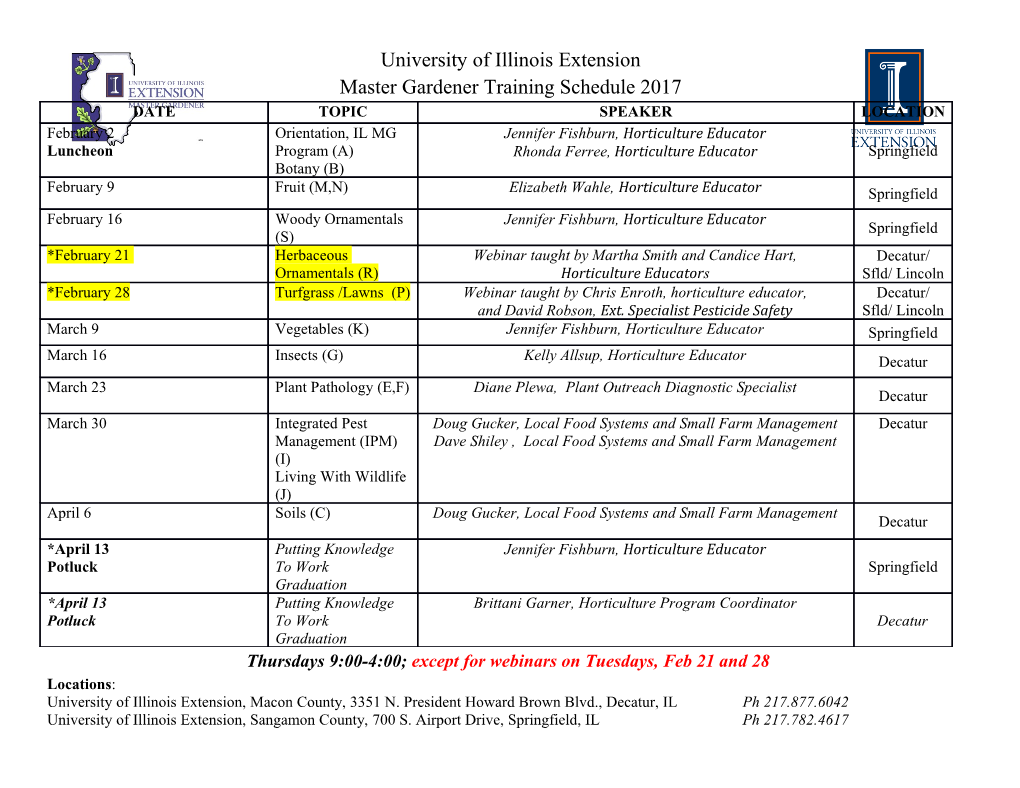
THE DEVELOPMENT OF A FUZZY EXPERT SYSTEM TO HELP TOP DECISION MAKERS IN POLITICAL AND INVESTMENT DOMAINS A thesis submitted in partial fulfillment of the requirements for the degree of Doctor of Philosophy By Sameera Alshayji School of Information Systems, Computing and Mathematics Brunel University May 2012 1 A B S T R A C T The world’s increasing interconnectedness and the recent increase in the number of notable regional and international events pose greater and greater challenges for political decision- making, especially the decision to strengthen bilateral economic relationships between friendly nations. Typically, such critical decisions are influenced by certain factors and variables that are based on heterogeneous and vague information that exists in different domains. A serious problem that the decision-maker faces is the difficulty in building efficient political decision support systems (DSS) with heterogeneous factors. One must take many factors into account, for example, language (natural or human language), the availability, or lack thereof, of precise data (vague information), and possible consequences (rule conclusions). The basic concept is a linguistic variable whose values are words rather than numbers and are therefore closer to human intuition. A common language is thus needed to describe such information which requires human knowledge for interpretation. To achieve robustness and efficiency of interpretation, we need to apply a method that can be used to generate high-level knowledge and information integration. Fuzzy logic is based on natural language and is tolerant of imprecise data. Fuzzy logic’s greatest strength lies in its ability to handle imprecise data, and it is perfectly suited for this situation. In this thesis, we propose to use ontology to integrate the scattered information resources from the political and investment domains. The process started with understanding each concept and extracting key ideas and relationships between sets of information by constructing object paradigm ontology. Re-engineering according to the object- paradigm (OP) provided quality for the developed ontology where conceptualization can provide more expressive, reusable object and temporal ontology. Then fuzzy logic has been integrated with ontology. And a fuzzy ontology membership value that reflects the strength of an inter-concept relationship to represent pairs of concepts across ontology has been consistently used. Each concept is assigned a fixed numerical value representing the concept consistency. Concept consistency is computed as a function of strength of all the relationships associated 2 with the concept. Fuzzy expert systems enable one to weigh the consequences (rule conclusions) of certain choices based on vague information. Rule conclusions follow from rules composed of two parts, the if antecedent (input) and the then consequent (output). With fuzzy expert systems, one uses fuzzy logic toolbox graphical user interface (GUI) tools to build up a fuzzy inference system (FIS) to aid in decision-making. This research includes four main phases to develop a prototype architecture for an intelligent DSS that can help top political decision makers. 3 TABLE OF CONTENTS ABSTRACT 2 LIST OF FIGURES 6 LIST OF TABLES 8 ACKNOWLEDGMENTS 10 SUPPORTING PUBLICATIONS 11 CHAPTER ONE 12 INTRODUCTION 12 1.1 Overview 12 1.2 Current challenges 13 1.3 Key issues 19 1.4 Regional events 21 1.5 Problem formulation 27 1.6 Existing methods 27 1.7 Aims and objectives 29 1.8 Motivation 29 1.9 Structure of the thesis 31 CHAPTER TWO 34 BACKGROUND 34 2.1 Introduction Error! Bookmark not defined. 2.2 Ontology Error! Bookmark not defined. 2.3 Fuzzy ontology 39 2.4 Domains of application 37 2.5 Fuzzy set and membership 39 2.6 Fuzzy logic uses 39 2.7 Ontology in the e-government domain 40 2.8 Existing tools to edit ontology 40 2.9 Fuzzy cognitive mapping (FCM) 43 CHAPTER THREE 45 METHODOLOGY 45 3.1 Introduction 45 3.2 Purpose of the ontology 49 3.3 Proposed ontology 47 3.3.1 Describe the concepts 52 3.3.2 Construct object paradigm (OP) paradigm ontology and use Protégé-owl editor (clarify the generation of domain ontology) 53 3.3.3 Construct fuzzy cognitive map theory and fuzzy causal algebra (define the domain ontology ext- ended as fuzzy ontology) 54 3.3.4 Construct fuzzy expert system 54 3.4 Summary 55 CHAPTER FOUR 59 DOMAIN ONTOLOGY CONSTRUCTION 59 4.1 Introduction 59 4.2 Input the unstructured data 59 4.3 Specify the definition of related concept in the domain and their relationships 58 4.3.1 The concept of the office of the decision-maker (political domain) 59 4.3.2 The concept of the prime minister's office domain (political domain) 60 4.3.3 The concept of the Kuwait Fund for Arab Economic Development (investment domain) 60 4.3.4 The concept of the General Authority for Investment (investment domain) 61 4.3.5 The concept of the Ministry of Foreign Affairs (political domain) 62 4.3.5.1 The concept of the bilateral relationships between countries 62 4.3.5.2 The concept of nuclear affairs 63 4.3.5.3 The concept of Middle East peace 64 4.3.5.4 The concept of relations between neighboring countries 64 4.3.5.5 The concept of the fight against terrorism 65 4 4.3.5.6 The concept of the agreement 65 4.4 Clarify the generation of domain ontology 69 4.4.1 Illustrative case: engineering agreement ontology in the Ministry of Foreign Affairs (political dom- ain) by constructing an object paradigm ontology and using the Protégé owl editor 68 A. using object paradigm (op) ontology 68 B. Presenting some properties and relations that hold the agreement concepts 71 C. Presenting the agreement concept by using Protégé OWL editing tools ontology. 73 4.4.2 Illustrative case: engineering the office of the decision maker ontology (political domain) 79 4.4.3 Illustrative case: engineering a position ontology in the Prime Minister’s Office (political domain)82 4.4.4 Illustrative case: engineering a loan ontology in the Kuwait Fund for Development (investment do- main) 84 4.4.5 Illustrative case: engineering (the bilateral trade concept) in the General Authority for Investment (investment domain) 88 4.5 Define the domain ontology extended as fuzzy ontology 90 4.5.1 Construct fuzzy logic and membership in a political domain 91 4.5.1.1 Case study –bilateral relationship 92 4.5.2 Construct Fuzzy ontology structures and semantic relations for political and investment doma- ins: 96 4.5.3 Construct fuzzy cognitive map theory (fcm) in political domain: fcm model for evaluation 98 4.5.4 Use of fuzzy causal algebra to clarify the relationships between factors: 102 4.5.5 Conclusion 105 4.5.6 Construct fuzzy expert system in the political domain 107 4.5.6.1 Introduction 107 4.5.6.2 Methodology 108 4.5.6.3 Data and modeling scenario 110 4.5.6.4 First step: construction of an fis editor 111 4.5.6.5 Identify variables 112 4.5.6.6 Second step: construct the membership function editor 114 4.5.6.7 Third step: construct the rules-rule editor 124 4.5.6.8 Rules viewer 128 4.5.6.9 Fourth step: defuzzify the result 129 4.6 Apply the fuzzy ontology to the specific domain. 130 4.6.1 Test 1 130 4.6.2 Test 2 132 4.6.3 Surface viewer 135 CHAPTER FIVE 136 CONCLUSIONS AND FUTURE WORK 138 5.1 conclusion Error! Bookmark not defined. 5.2 contribution 148 5.3 future work 150 REFERENCES ERROR! BOOKMARK NOT DEFINED. 5 LIST OF FIGURES FIGURE 3.1: THE PROCESS OF CONSTRUCTION OF FUZZY ONTOLOGY FOR SPESIFIC DOMAINS ................. 50 FIGURE 3.2: THE DEVELOPED PROCESS OF CONSTRUCTION OF FUZZY ONTOLOGY .................................. 51 FIGURE 4.1: THE OFFICE OF POLITICAL DECISION - MAKING ONTOLOGY .................................................. 62 FIGURE 4.2: ENGINEERING PROPERTIES RELATING TO AN AGREEMENT ................................................... 71 FIGURE 4.3: ENGINEERING RELATIONSHIP RELATING TO THE AGREEMENT ……………………………..72 FIGURE 4.4: THE OP AGREEMENT ONTOLOGY ......................................................................................... 73 FIGURE 4.5: SOME INDIVIDUALS IN POLITICAL AND INVESTMENT DOMAIN……………………………..76 FIGURE 4.6: BILATERAL AGREEMENT CLASS HAS DIFFERENT SUBCLASSES CONCEPT .. ............................ 77 FIGURE 4.7: OWLVIZ DISPLAY OF THE ASSERTED AND INFERREDHIERARCHIES ....................................... 78 FIGURE 4.8: A MODEL OF AGREEMENT ONTOLOGY ................................................................................. 79 FIGURE 4.9: MODEL OF THE MINISTRY FOR FOREIGN AFFAIRS ORGANIZATION PRESENTED BY PROTÉGÉ -OWL ONTOLOGY EDITING TOOL .............................................................................................................. 80 FIGURE 4.10: MODEL OF THE AGERRMENT TYPE CONCEPT PRESENTED BY PROTÉGÉ -OWL ONTO - LOGY EDITITING TOOL ............................................................................................................................. 81 FIGURE 4.11: EACH 'COUNTRYNAME ' CLASS HAVE DIFFERENT CONNECTION .......................................... 82 FIGURE 4.12: EACH COUNTRY HAS DIFFERENT CATEGORY ..................................................................... 83 FIGURE 4.13: POLITICAL OFFICE OP ONTOLOGY ...................................................................................... 84 FIGURE 4.14: OP ONTOLOGY RELATING TO THE POSITION CONCEPT ........................................................ 86 FIGURE 4.15: DESIGNING PROPERTIES RELATED TO THE MINUTES OF THE MEETING IN THE PRIME MINISTER 'S OFFICE ..........................................................................................................................................
Details
-
File Typepdf
-
Upload Time-
-
Content LanguagesEnglish
-
Upload UserAnonymous/Not logged-in
-
File Pages152 Page
-
File Size-