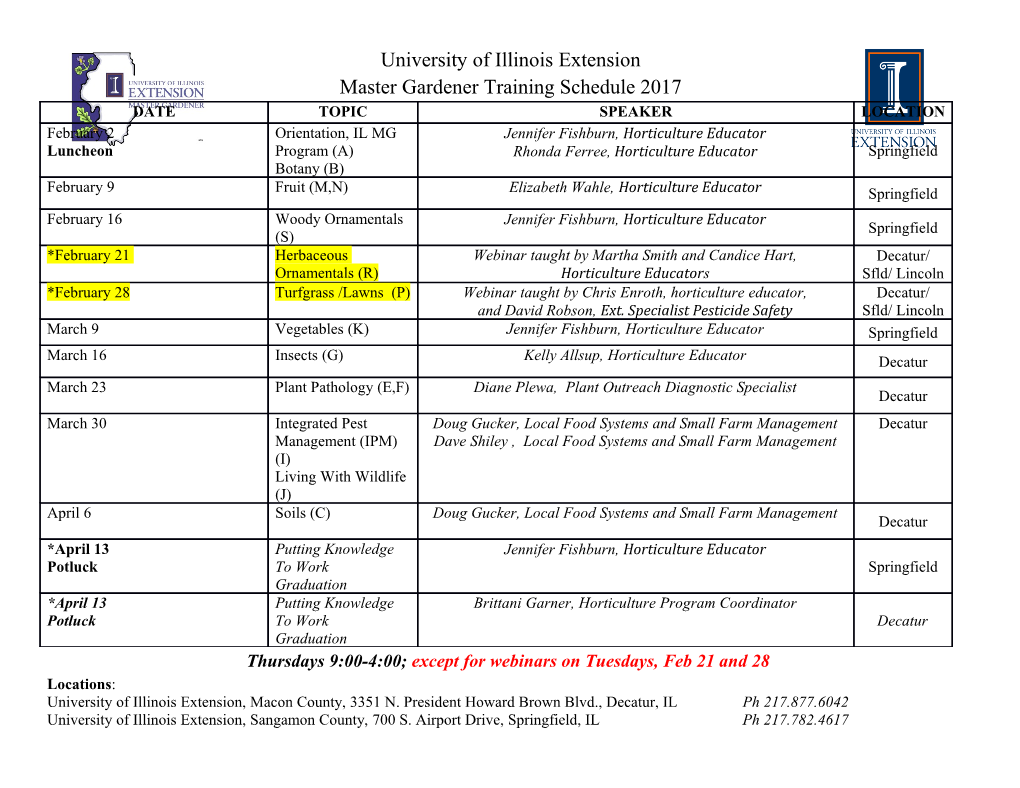
Staff Working Paper/Document de travail du personnel 2019-28 Tail Index Estimation: Quantile- Driven Threshold Selection by Jon Danielsson, Lerby M. Ergun, Laurens de Haan and Casper G. de Vries Bank of Canada staff working papers provide a forum for staff to publish work-in-progress research independently from the Bank’s Governing Council. This research may support or challenge prevailing policy orthodoxy. Therefore, the views expressed in this paper are solely those of the authors and may differ from official Bank of Canada views. No responsibility for them should be attributed to the Bank. www.bank-banque-canada.ca Bank of Canada Staff Working Paper 2019-28 August 2019 Tail Index Estimation: Quantile-Driven Threshold Selection by Jon Danielsson,1 Lerby M. Ergun,2 Laurens de Haan3 and Casper G. de Vries4 1 London School of Economics 2 Financial Markets Department Bank of Canada Ottawa, Ontario, Canada K1A 0G9 [email protected] 3 Erasmus University Rotterdam 4 Erasums University Rotterdam Tinbergen Institute ISSN 1701-9397 © 2019 Bank of Canada Acknowledgements Corresponding author Lerby M. Ergun. We thank the Netherlands Organisation for Scientific Research Mozaiek grant [grant number: 017.005.108] and the support of the Economic and Social Research Council (ESRC) in funding the Systemic Risk Centre [grant number ES/K002309/1] i Abstract The selection of upper order statistics in tail estimation is notoriously difficult. Methods that are based on asymptotic arguments, like minimizing the asymptotic MSE, do not perform well in finite samples. Here, we advance a data-driven method that minimizes the maximum distance between the fitted Pareto type tail and the observed quantile. To analyze the finite sample properties of the metric, we perform rigorous simulation studies. In most cases, the finite sample-based methods perform best. To demonstrate the economic relevance of choosing the proper methodology, we use daily equity return data from the CRSP database and find economically relevant variation between the tail index estimates. Bank topics: Financial stability; Econometric and statistical methods JEL codes: C01, C14, C58 ii 1 Introduction In various research fields, like geography and economics, distributions ex- hibit heavy tails, e.g., the scaling behaviour described by the power laws of Pareto. In this literature, the tail index, α, is the shape parameter in the power that determines how heavy the tail is (a higher α corresponds to a thinner tail). The most popular estimator for the tail index is by Hill (1975), which is the quasi-maximum likelihood estimator. In the statistical literature, there is an ongoing debate on the number of tail observations k that are used in the estimation of α. The choice of k leads to a trade-off between the bias and variance of the estimator. Although practitioners tend to use \Eye-Ball" methods (Resnick and Starica, 1997), the typical approach suggested in the statistical literature is choosing k by minimizing the asymp- totic mean squared error (MSE). The methods that are used to find k are asymptotically consistent but have unsatisfactory finite sample properties. This paper proposes a novel approach for choosing the optimal k, labelled k∗. The methodology is based on fitting the tail of a heavy-tailed distribution by minimizing the maximum deviation in the quantile dimension. We show this method outperforms other methods put forth in the literature. Hall(1990) and Danielsson et al.(2001) use a bootstrap procedure to mini- mize the MSE. Drees and Kaufmann(1998) exploit the same bias and vari- ance trade-off but use the maximum random fluctuation of the estimator to locate the point where the trade-off is optimal. These methods are based on asymptotic arguments, but these methods may not perform very well in finite samples. The shortcomings of the currently available methods motivate our new ap- proach. In this paper, we propose using the penalty function of the Kolmogorov- Smirnov (KS) test statistic to fit the tail of the distribution. The KS statistic is measured as the maximum difference between the empirical distribution and a parametric distribution in the probability dimension. Clauset et al. (2009) use this metric to find the optimal k. In a response, Drees et al. (2018) find that the KS statistic chooses values that are too low for the op- timal k and therefore introduces a large variances for the Hill estimate. In this paper, we use KS statistic, but with a twist. Instead of minimizing the distance between the empirical distribution and the parametric distribution in the probability dimension, we minimize the distance in the quantile di- mension. This measure is henceforth referred to as the KS-distance metric. All heavy-tailed distributions in the sense of regular variation (see below) are characterized by ultimate power law behaviour. For a large subset of 2 distributions, the tails, to a first-order expansion at infinity, correspond to the tail of a Pareto distribution. The estimates of the shape parameter α use k of the highest order statistics, assuming that these order statistics fit well to a Pareto tail. By varying k, we are able to simultaneously fit the empirical distribution and elicit k∗. The particular choice of the metric is mainly motivated by the fact that small deviations in the probability distribution lead to increasingly large dis- tortions in the quantile dimension. Deep into the tail, the difference in the probability dimension is of order 1=n, but in the quantile dimension this is of 1=α order (n=k) . This effect is amplified as one moves deeper into the tail of the distribution. Basing the metric in the quantile domain therefore puts a natural emphasis on fitting tail observations as opposed to the centre obser- vations. Furthermore, by focusing on the maximum, the metric is not diluted by the numerous centre observations. These intuitive arguments are backed by rigorous simulation analyses and tests. In the simulation study, we draw i.i.d. samples from the Fr´echet, sym- metric stable and the Student-t distribution. For these distribution families, Hall's second-order expansion of the cumulative distribution function (CDF) holds. Given this expansion, Hall and Welsh(1985) derive the theoretical k∗, which minimizes the asymptotic MSE. This allows us to study the relation- ship between the chosen k∗ and the estimated tail index. Furthermore, we employ dependent time series in the simulation exercise. The autoregressive conditional heteroskedastic (ARCH) stochastic processes by Engle(1982) model the volatility clustering in time-series data. For the ARCH process, we do not know the variance and the bias of the Hill estimator. However, the tail index is known. Therefore, we are able to evaluate the performance of the methods under the clustering of extremes. To test the performance of the KS-distance metric, we analyze it from four different perspectives. Firstly, we test the KS-distance metric against vari- ous other penalty functions. We use the MSE, mean absolute error and the metric used in Dietrich et al.(2002) to benchmark the performance of our penalty function. To evaluate the ability of different metrics to correctly penalize errors, we use results derived by Hall and Welsh(1985). Hall and Welsh derive the theoretical k∗ for the class of distributions that adhere to Hall's second-order expansion of the CDF. These results stipulate the be- haviour of k∗ as a function of the sample size and α. 3 Secondly, we contrast the performance of the KS-distance metric with the other existing methods. We find that the KS-distance metric and the auto- mated Eye-Ball method outperform other heuristic and statistical method- ologies. For the Student-t, symmetric stable and the Fr´echet distributions, the competing methodologies do not reproduce the expected patterns for k∗ as derived by Hall and Welsh(1985). This leads to a larger bias in ^α for less- heavy-tailed distributions. The theoretically motivated methodologies often choose a high value for k∗ (too many order statistics close to the centre of the distribution), which corresponds to a large bias. For the ARCH processes, the methods by Drees and Kaufmann(1998), Danielsson et al.(2001) and the fixed sample fraction introduce a large bias in the Hill estimator. For the dependent time series, the automated Eye-Ball method and the KS-distance metric produce small biases. This is comforting as most economic time series exhibit volatility clustering. In addition to estimating the tail index, we model the quantile estimates for the various competing methods, since the ultimate goal is to produce/obtain reliable estimates of the possible losses. When we analyze the quantile esti- mates, the KS-distance metric and the automated Eye-Ball method perform best for the quantiles greater than the 99% probability level. For the quan- tiles further towards the centre of the distribution, other methods produce smaller errors. Both the KS-distance metric and the automated Eye-Ball method have a tendency to pick a small k∗ and therefore fit the tail close to the maximum. The other methodologies often use a larger number of order statistics and consequently fit better closer towards the centre of the distri- bution. In the last section of this paper, we show that the choice of k∗ can be eco- nomically important. For this purpose we use individual daily stock price in- formation from the Centre for Research in Security Prices (CRSP) database. For 17,918 individual stocks, we estimate the left and right tail index of the equity returns. We measure the average absolute difference between the es- timated α and find that the average difference between the methods ranges from 0.39 to 1.44. These differences are outside of the confidence interval of the Hill estimator.
Details
-
File Typepdf
-
Upload Time-
-
Content LanguagesEnglish
-
Upload UserAnonymous/Not logged-in
-
File Pages50 Page
-
File Size-