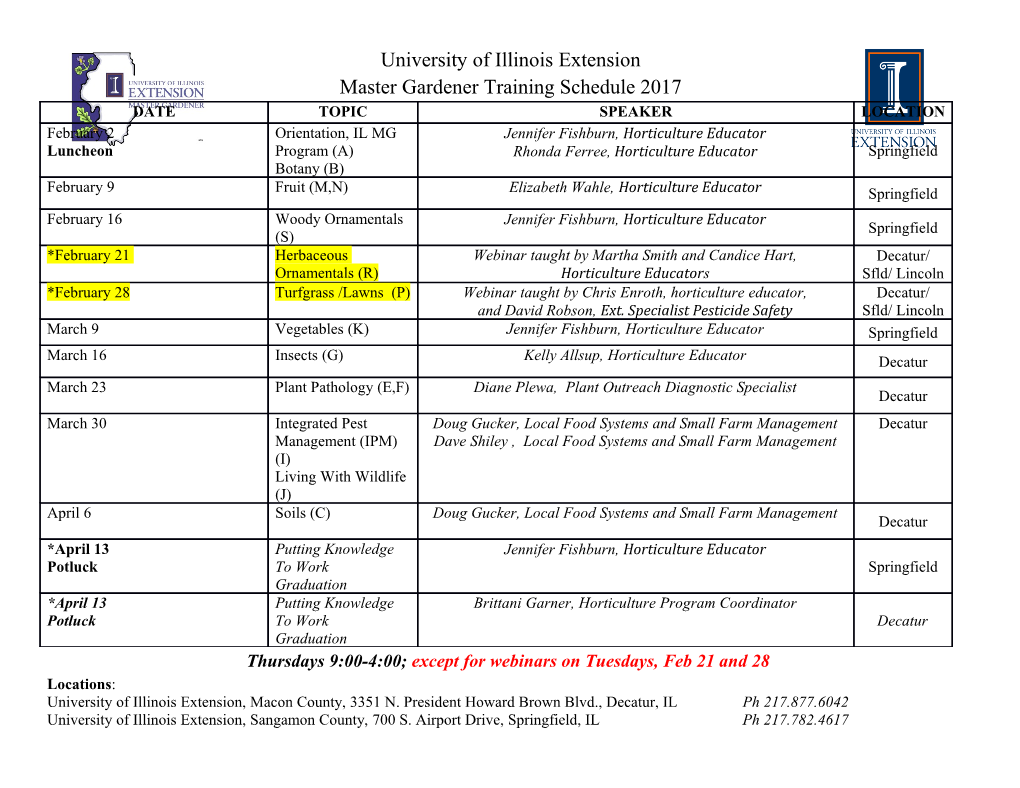
Unit 10: Split-Plot Designs, Repeated Measures, and Expected Mean Squares STA 643: Advanced Experimental Design Derek S. Young 1 Learning Objectives I Become familiar with whole plots and subplots as they pertain to split-plot designs I Know how to analyze a split-plot design I Understand the different sources of experimental error in split-plot designs I Know how to calculate standard errors for treatment factor means in a split-plot design I Become familiar with split-split-plot and split-block designs I Understand repeated measures designs and their connection to split-plot designs I Know how to handle ranked data in a repeated measures design I Become familiar with the steps for determining expected mean squares 2 Outline of Topics 1 Split-Plot Designs 2 Split-Split-Plot Designs 3 Split-Block Designs 4 Repeated Measures Designs 5 Handling Ranked Data 6 Expected Mean Squares 3 Outline of Topics 1 Split-Plot Designs 2 Split-Split-Plot Designs 3 Split-Block Designs 4 Repeated Measures Designs 5 Handling Ranked Data 6 Expected Mean Squares 4 Example: Agricultural Experiment We motivate our discussion by considering an agricultural investigation to study the effects of two irrigation methods (factor A) and two fertilizers (factor B) on yield of a crop. Let A1 and A2 be the two levels of factor A and B1 and B2 be the two levels of factor B. Four fields are available as the EUs. The four treatments { A1B1, A1B2, A2B1, and A2B2 { are assigned at random to the four fields in a CRD. Since there are four treatments and four EUs, there will be no df for estimation of error as shown in the abbreviated ANOVA table below. However, if the fields could be divided into smaller fields, replicates of each factor-level combination could be obtained and the error variance could then be estimated. In this case, the smaller fields become the MUs. Unfortunately, in this investigation, it is not possible to apply different irrigation methods (factor A) in areas smaller than a field (the EU), although different fertilizer types (factor B) could be applied in relatively small areas. Such a situation is where a split-plot design can be used. Source of Variation df Factor A 1 Factor B 1 AB Interaction 1 Error 0 Total 3 5 Background on Split-Plot Designs I As noted in the motivating example, one factor sometimes requires more experimental material for its evaluation than a second factor in factorial experiments. I In agricultural studies, a factor { such as irrigation method { may only be applicable (or best-suited) for large plots, while another factor { such as fertilizers { may be applied easily to smaller plots. I The larger treatment is applied to what we call the whole plots, which is then split into smaller subplots. Designs that accommodate this allocation of treatments are called split-plot designs. I The usage of the term plots stems from split-plot designs being developed for agricultural studies; while still commonly found in agriculture, split-plot designs are also used in laboratory, industrial, and social science experiments. I The primary advantage of a split-plot design is that it allows us to design an experiment when one factor requires considerably more experimental material than another factor, or accommodate the situation where there is an opportunity to study responses to a second factor while efficiently utilizing resources. I The primary disadvantage of a split-plot design is the potential loss in treatment comparisons and an increase in complexity of the statistical analysis (which we will see). 6 Randomization for Split-Plot Designs I In split-plot designs, the usual randomization of treatment combinations to the EUs is altered to accommodate the particular requirements of the experiment. I In the usual CRD, all treatment combinations are assigned randomly to the EUs. I However, in the split-plot design, each level of the second factor is combined with a single level of the first factor (i.e., the factor requiring more experimental material), which has been assigned to the whole plot. I In other words, at the first level of randomization, the whole plot treatments are randomly assigned to whole plots; at the second level, the subplot treatments are randomly assigned to the subplots. 7 Example: Agricultural Experiment As we noted in the agricultural experiment, the irrigation methods (factor A) can only be applied to one field as a whole. In the figure below, we see that the four fields have been randomly assigned to one of the two factor levels of A. Within each field (the whole plot), it is divided into two subplots. Each of these subplots is then randomly assigned to one of the two levels of factor B. Through this split-plot design, we have increased our total number of EUs N from 4 to 8. Field&1 Field&2 Field&3 Field&4 A2 A1 A1 A2 B1 B2 B1 B2 Subplot&Factor&B B2 B1 B2 B1 Whole&Plot& Factor&A 8 Two Experimental Errors for Split-Plot Designs I In the analysis of split-plot designs, we must account for the presence of two different sizes of EUs used to test the effect of the whole plot treatment and subplot treatment. I Factor A effects are estimated using the whole plots and factor B and the A × B interaction effects are estimated using the subplots. I Since the size of whole plot and subplots are different, they have different precisions. I Moreover, two separate errors account for the fact that observations from different subplots within each whole plot may be positively correlated, since it is natural to assume that EUs adjacent to one another are likely to respond similarly. I For example, consider neighboring field plots, students in a classroom, or the batch of some raw material in an industrial experiment. 9 Two Experimental Errors for Split-Plot Designs I We assume that I there is a correlation ρ between observations on any two subplots in the same whole plot, and I observations from two different whole plots are uncorrelated. I Given the above assumptions and assuming that there are b subplots for each whole plot, then I the error variance for the main effects of A on a per-subplot basis is σ2[1 + (b − 1)ρ], and I the error variance per subplot for the main effects of B and the interaction A × B is σ2(1 − ρ). I These different error variances result in a different SS partitioning for the ANOVA. I The partitions for blocking and factor effects remain the same as for a factorial design, but the experimental error is partitioned into two components: one for the whole-plot treatment factor and one for the subplot treatment factor and interaction. 10 Split-Plot Model I For the split-plot design, a mixed-model formulation is used with separate random error effects for the whole-plot units and subplot units. I Placing the whole-plot treatment in an RCBD, a linear model for the split-plot design is Yijk = µ + αi + ρk + dik + βj + (αβ)ij + ijk; (1) where I µ is the overall mean (a constant); I i = 1; : : : ; a, j = 1; : : : ; b, and k = 1; : : : ; n; Pa I αi are the (fixed) effects of factor A subject to the constraint αi = 0; Pni=1 I ρk are the (fixed) effects of block k subject to the constraint k=1 ρk = 0; I dik are the (random) effects for the whole-plot random error and are iid normal with mean 0 and 2 variance σd; Pb I βj are the (fixed) effects of factor B subject to the constraint j=1 βj = 0; I (αβ)ij are the (fixed) effects due to the interaction of A and B subject to the constraint Pa Pb i=1(αβ)ij = j=1(αβ)ij = 0; 2 I ijk is the subplot (random) error and are iid normal with mean 0 and variance σ ; and I dik and ijk are assumed independent of one another. I Note that randomization of treatments is what justifies the assumption of independence for the two random errors and the equal correlation between the errors for subplot units on the same whole-plot unit. 11 SS Decomposition I The total deviation for the split-plot model on the previous slide is total deviation: (yijk − y¯···); which is the sum of the following components: block deviation: (¯y··k − y¯···) factor A deviation: (¯yi·· − y¯···) whole-plot error: (¯yi·k − y¯i·· − y¯··k +y ¯···) factor B deviation: (¯y·j· − y¯···) interaction AB deviation: (¯yij· − y¯i·· − y¯·j· +y ¯···) subplot error: (yijk − y¯ij· − y¯i·k +y ¯i··) 12 SS Decomposition I The SS of the decomposition on the previous slide (where the sums of cross-products drop out) yields Block effect: = Factor A effect: = Whole-plot error: = Factor B effect: = Interaction AB effect: = Subplot error: = 13 ANOVA for Split-Plot Designs I Finally, we can obtain the following ANOVA table: Source df SS MS E(MS) Whole Plots P 2 2 2 k ρk Block (n − 1) SSBlk MSBlk σ + bσd + ab n−1 P 2 2 2 i αi A (a − 1) SSA MSA σ + bσd + nb a−1 2 2 Whole-Plot Error (a − 1)(n − 1) SSWP MSWP σ + bσd Subplots P β2 2 j j B (b − 1) SSB MSB σ + na b−1 P P (αβ)2 2 i j ij AB (a − 1)(b − 1) SSAB MSAB σ + n (a−1)(b−1) Subplot Error a(b − 1)(n − 1) SSSP MSSP σ2 Total abn − 1 SSTot I The E(MS) for the whole-plot error and the subplot error reflect the differences in the variability for the two different types of EUs { namely, the expected error variances for the whole plots are larger than those for the subplots.
Details
-
File Typepdf
-
Upload Time-
-
Content LanguagesEnglish
-
Upload UserAnonymous/Not logged-in
-
File Pages87 Page
-
File Size-