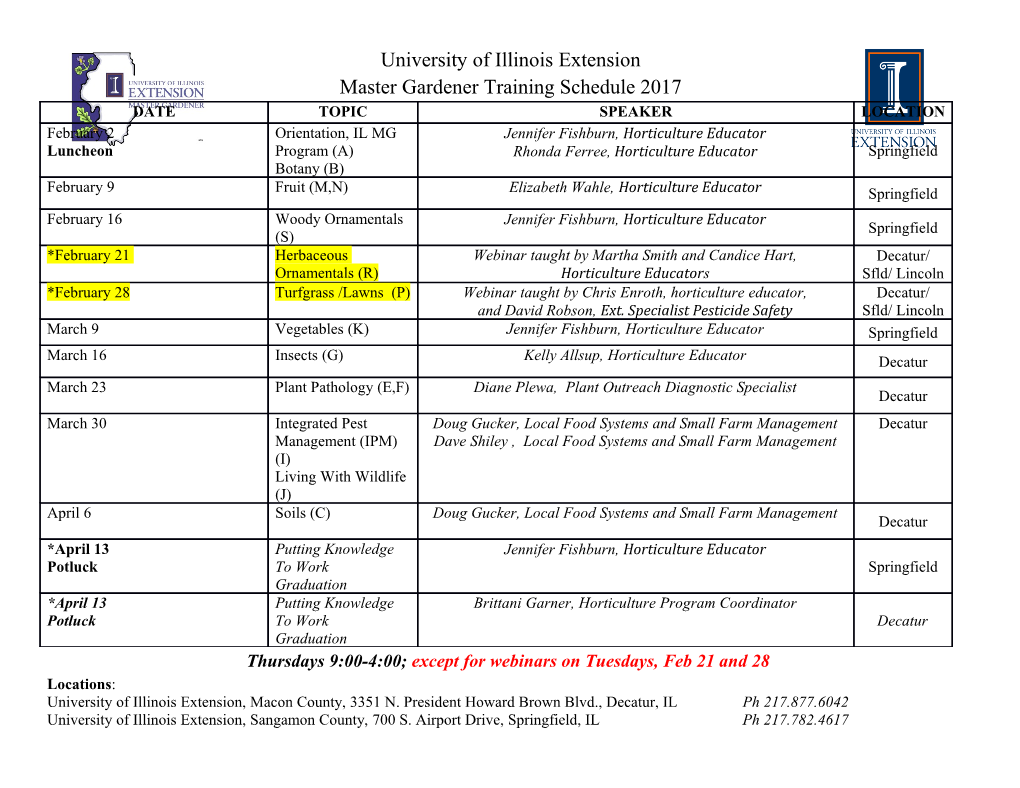
Compilers Optimization Yannis Smaragdakis, U. Athens (or ig ina l s lides by Sam Guyer @Tu fts ) What we already saw z Lowering FlFrom language-lllevel cons ttttructs to mac hine-lllevel cons tttructs z At this point we could generate machine code z Output of lowering is a correct translation z What’s left to do? z Map from lower-level IR to actual ISA z Maybe some register management (could be required) z Pass off to assembler z Whyyp have a separate assembler? z Handles “packing the bits” Assembly addi <target>, <source>, <value> MhiMachine 0010 00ss ssst tttt iiii iiii iiii iiii 2 But first… z The compiler “understands” the program z IR captures program semantics z Lowering: semantics-preserving transformation z Why not d o oth ers ? z Compppiler optimizations z Oh great, now my program will be optimal! z Sorry, it’s a misnomer z What is an “optimization”? 3 Optimizations z What are they? z Code transformations z Improve some metric z Metrics z Performance: time, instructions, cycles AthAre these me titrics equ ilt?ivalent? z Memory z Memory hierarchy (reduce cache misses) z Reduce memory usage z Code Size z Energy 4 Big picture Errors Semantic chars Scanner tokens Parser AST checker Optimizations Checked Instruction IR lowering AST tuples selection assembly Register assembly Machine Assembler ∞ registers allocation k registers code 5 Big picture Errors Optimizations Semantic chars Scanner tokens Parser AST checker Checked Instruction IR lowering AST tuples selection assembly Register assembly Machine Assembler ∞ registers allocation k registers code 6 Why optimize? z High-level constructs may make some A[i][j] = A[i][j-1]+11] + 1 optimizations difficult t = A + i*row + j or impossible: ssoj = A + i*row + j – 1 (*t) = (*s) + 1 z High-level code may be more desirable z Program at high level z Focus on design; clean, modular implementation z Let compiler worry about gory details z Premature optimization is the root of all evil! 7 Limitations z What are optimizers good at? z Consistent and thorough z Find all opportunities for an optimization z Uniformly apply the transformation z What are they not good at? z Asyypmptotic comp lexit y z Compilers can’t fix bad algorithms z Compilers can’t fix bad data structures z There’s no magic 8 Requirements z Safety z Preserve the semantics of the program z What does that mean? z Profitability z Will it help our metric? z Do we need a guarantee of improvement? z Risk z How will interact with other optimizations? z How will it affect other stages of compilation? 9 Example: loop unrolling for ((;i=0; i<100; i++ ) for ((;i=0; i<25; i++ ){) { *t++ = *s++; *t++ = *s++; *t++ = *s++; *t++ = *s++; *t++ = *s++; } z Safety: z Always safe; getting loop conditions right can be tricky. z Profitability z Depends on hardware – usually a win – why? z Risk? z Increases size of code in loop z May not fit in the instruction cache 10 Optimizations z Manyyy, many optimizations invented z Constant folding, constant propagation, tail-call elimination, redundancy elimination, dead code elimination, loop- invariant code motion, loop splitting, loop fusion, strength reduction, array scalarization, inlining, cloning, data prefetching, parallelization. .etc . z How do they interact? z Optimist: we get the sum of all improvements! z RlitRealist: many are iditin direct oppos ition 11 Rough categories z Traditional optimizations z Transform the program to reduce work z Don’t change the level of abstraction z Resource allocation z Map program to specific hardware properties z Register allocation z Instruction scheduling, parallelism z Data streaming, prefetching z EblitEnabling trans forma tions z Don’t necessarily improve code on their own z Inlining, loop unrolling 12 Constant propagation z Idea z If the value of a variable is known to be a constant at compile-time, replace the use of variable with constant n = 10; n = 10; c = 2; c = 2; for (i=0;i<n;i++) for (i=0;i<10;i++) s = s +i*+ i*c; s = s +i*+ i*2; z Safety z Prove the value is constant z Notice: z May interact favorably with other optimizations, like loop unrolling – now we know the trip count 13 Constant folding z Idea z If operand s are known a t compil e-time, eva lua te expression at compile-time r=3141*10;r = 3.141 * 10; r=3141;r = 31.41; z What do we need to be careful of? z IthIs the resu ltthlt the same as if execu tdtted at runti ti?me? z Overflow/underflow, rounding and numeric stability z Often repeated throughout compiler x = A[2]; t1 = 2*4; t2 = A + t1; x = *t2; 14 Partial evaluation z Constant propaggggation and folding together z Idea: z Evaluate as much of the ppgrogram at compile-time as possible z More sophisticated schemes: z Simulate data structures, arrays z SbliSymbolic execu tifthdtion of the code z Caveat: floating point z Preserv ing the error c harac ter is tics o f floa ting po in t va lues 15 Algebraic simplification z Idea: z Apply the usual algebraic rules to simplify expressions a * 1 a a/1 a a * 0 0 a + 0 a b || false b z Reppyppyppeatedly apply to complex expressions z Many, many possible rules z Associativity and commutativity come into play 16 Common sub-expression elimination z Idea: z If program computes the same expression multiple times, reuse the value. a = b + c; tb+t = b + c c = b + c; a = t; d = b + c; c = t; d=b+c;d = b + c; z Safety: z Subexpression can only be reused until operands are redefined z Often occurs in address computations z Array inde xing and str u ct/field accesses 17 Dead code elimination z Idea: z If the result of a computation is never used, then we can remove the computation x=y+1;x = y + 1; y = 1; y = 1; x = 2 * z; x = 2 * z; z Safety z Variable is dead if it is never used after defined z Remove code that assigns to dead variables z This may, in turn, create more dead code z Dead-code elimination usually works transitively 18 Copy propagation z Idea: z After an assignment x = y, replace any uses of x with y x = y; x = y; if (x>1) if (y>1) s = x+f(x); s = y+f(y); z SftSafety: z Only apply up to another assignment to x, or z …another assignment to y! z What if there was an assignment y = z earlier? z Apply transitively to all assignments 19 Unreachable code elimination z Idea: z Eliminate code that can never be executed #define DEBUG 0 . if (DEBUG) print(“Current value = “, v); z Different implementations z High-level: look for if (false) or while (false) z Low-level: more difficult z Code is just labels and gotos z TthhkihblblkTraverse the graph, marking reachable blocks 20 How do these things happen? z Who would write code with: z Dead code z Common subexpressions z CttConstant express ions z Copies of variables z TthTwo ways they occur z High-level constructs – we’ve already seen examples z Other optimizations z Copy propagation often leaves dead code z Enabling transformations: inlining, loop unrolling, etc. 21 Loop optimizations z Program hot-spots are usually in loops z Most programs: 90% of execution time is in loops z What are possible exceptions? OS k ernel s, compil ers an d in terpre ters z Loops are a good place to expend extra effort z Numerous loop optimizations z Often expensive – complex analysis z For languages like Fortran, very effective z What about C? 22 Loop-invariant code motion z Idea: z If a computation won’t change from one loop iteration to the next, move it outside the loop t1 = x *x; for (i=0;i<N;i++) for (i=0;i<N;i++) A[i] = A[i] + x*x; A[i] = A[i] + t1; z Safety: z Determine when expressions are invariant z Just check for variables with no assignments? z Useful for array address computations z Not visible at source level 23 Strength reduction z Idea: z Replace expensive operations (mutiplication, division) with cheaper ones (addition, subtraction, bit shift) z Traditionally applied to induction variables z Variables whose value depends linearly on loop count z Special analysis to find such variables v = 0; for (i=0;i<N;i++) for (i=0;i<N;i++) v = 4*i; A[v] = . A[v] = . v = v + 4; 24 Strength reduction z Can also be applied to simple arithmetic operations: x * 2 x + x x * 22c^c x<<c x/2^c x>>c z Typical example of premature optimization z Programmers use bit-shift instead of multiplication z “2”ihdtdtd“x<<2” is harder to understand z Most compilers will get it right automatically 25 Inlining z Idea: z Replace a function call with the body of the callee z Safety z What about recursion? z Risk z Code size z Most compilers use heuristics to decide when z Has been cast as a knapppsack problem 26 Inlining z What are the benefits of inlining? z Eliminate call/return overhead z Customize callee code in the context of the caller z Use actual arguments z Push into copy of callee code using constant prop z Apply other optimizations to reduce code z Hardware z Eliminate the two jumps z Keep the pipeline filled z Critical for OO languages z Methods are often small z Encapsu lation, modu larity force code apart 27 Inlining z In C: z At a call-site, decide whether or not to inline z (Often a heuristic about callee/caller size) z Look up the callee z Replace call with body of callee z What about Java? class A { void M() {…} } z What complicates this? class B extends A { void M() {…} } z Virtual methods z Even worse? void foo(A x) z Dynamic class loading { x.
Details
-
File Typepdf
-
Upload Time-
-
Content LanguagesEnglish
-
Upload UserAnonymous/Not logged-in
-
File Pages79 Page
-
File Size-