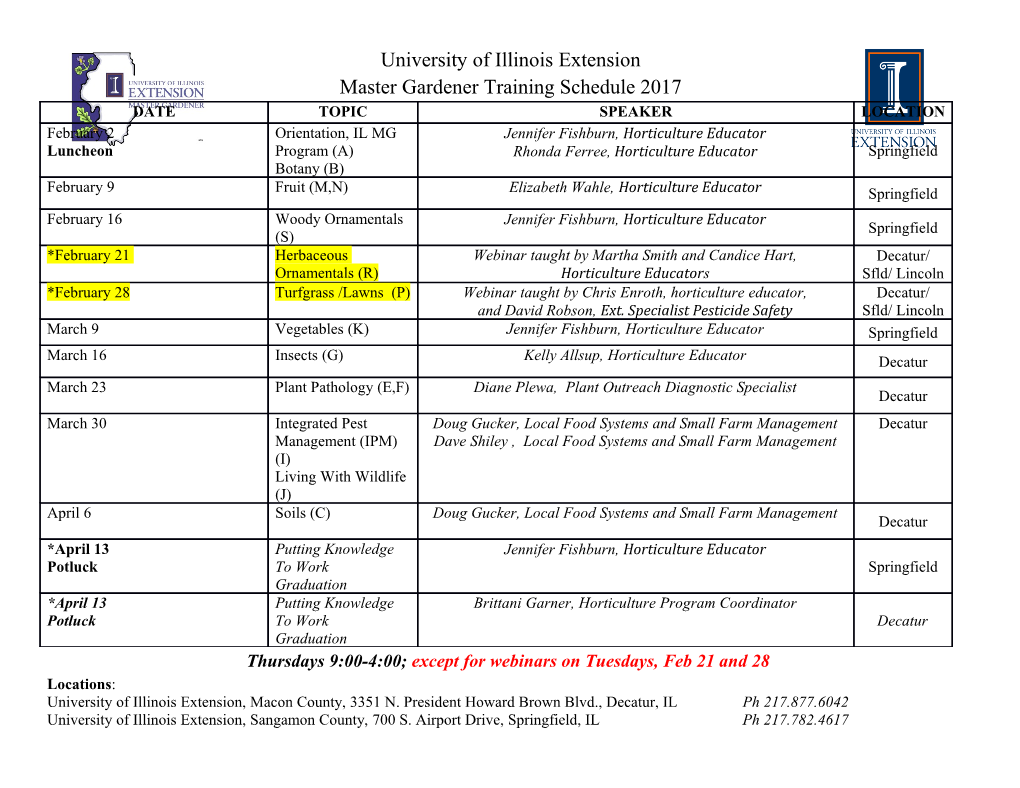
Molar and Molecular Models of Performance for Rewarding Brain Stimulation Yannick-André Breton A Thesis In the Department of Psychology Presented in Partial Fulfillment of the Requirements For the Degree of Doctor of Philosophy (Psychology) at Concordia University Montreal, Quebec, Canada August, 2013 c Yannick-André Breton, 2013 CONCORDIA UNIVERSITY SCHOOL OF GRADUATE STUDIES This is to certify that the thesis prepared By: Yannick-André Breton Molar and molecular models of performance Entitled: for rewarding brain stimulation and submitted in partial fulfillment of the requirements for the degree of Doctor of Philosophy (Psychology) complies with the regulations of the University and meets the accepted standards with respect to originality and quality. Signed by the final examining committee: Richard Courtemanche Chair C. Randy Gallistel External Examiner Lea Popovic External to Program Andreas Arvanitogiannis Examiner Rick Gurnsey Examiner Peter Shizgal Thesis Supervisor Approved by C. Andrew Cha p man Chair of Department or Graduate Program Director 29/10/2013 Joanne Locke Dean of Faculty Abstract Molar and Molecular Models of Performance for Rewarding Brain Stimu- lation Yannick-André Breton, Ph.D. Concordia University, 2013 This dissertation reports analyses of performance for rewarding brain stimu- lation in a three-part sequential task. A session of self-stimulation was composed of three trial types, during which the strength and opportunity cost of electrical stim- ulation were kept constant. Within a trial, a lever allowed animals to harvest brain stimulation rewards as soon as the lever had been held for a set, cumulative amount of time. When the time spent holding reached this criterion, the lever retracted and a burst of rewarding electrical stimulation was delivered. A flashing house light and 10s inter-trial interval signalled the start of a new trial. Rats were presented with strong/inexpensive/certain stimulation on one trial, a randomly selected strength, cost and risk on the next trial, and weak/inexpensive/certain stimulation on the third trial of a sequence. The sequence then repeated. Rewards during the second trial of this sequence were delivered with cued probabilities ranging from 0.5 to 1.0. The current thesis evaluates the ability of a previously published (molar) model of performance during a trial to accurately detect the effect of risk on payoff but not reward intensity. Although animals were less willing to work for stimulation trains that may not be delivered than those delivered with certainty, risk did not change the relative reward produced by stimulation. We also present evidence on a fine time scale that self-stimulating rats develop a model of their world. The first pause made - iii - as a trial began was a function of the payoff the animal had yet to receive, indicat- ing that rats had a model of the triad sequence. Analysis of the conditions under which pauses were uncharacteristic also provides evidence of what this model might be. Analysis of the fine scale of performance provides evidence that animals had a model of the stability of trial conditions. Finally, we present a (molecular) model of performance for brain stimulation rewards in real-time. Our results demonstrate that rats develop a model of the testing paradigm and can adjust to changes in reward contingencies with as few as one exemplar. -iv- Acknowledgements Sir Isaac Newton famously wrote in his letter to Robert Hooke that “if I have seen further, it is by standing on the shoulders of giants.” Obviously, I wouldn’t dare compare myself to the father of classical mechanics, or this thesis to the Principia Mathematica. I would, however, like to start by thanking all of those who made this possible, all of those who made this bearable, and all of those who contributed to the thinking that I attempted (and in some rare instances, accomplished) to communicate here. First, I owe a great deal to Dr. Peter Shizgal and the support he has pro- vided over the course of my graduate studies. He has forgotten more about reward than I could ever learn in a lifetime. Our weekly, bi-weekly, and monthly meetings, sometimes spent strolling the grounds of the University, have provided most of the reasoning that has gone into the experiments and analyses described here. While we’re at it, I would like to thank the Shizgal lab members, present and past, for all the help you gave along the way. Rebecca Solomon graciously allowed me to use the data she collected as part of her own graduate work for many of the studies presented here. I’d also like to especially thank Dr. Kent Conover, who has been absolutely instrumental to my learning MATLAB. On a similar note, our multi-way, multi-timezone teleconferences with Dr. Pe- ter Dayan and his brilliant student, Ritwik Niyogi, have provided so much fodder for intellectual discourse that, given enough time and energy, a completely separate dissertation could also be written. I look forward to continuing our amicable collab- oration. I have been blessed with an outstanding committee, whose contributions can- not be ignored. Thank you Drs. Andreas Arvanitogiannis, Rick Gurnsey, and Lea Popovic for your insightful questions on the many drafts this document has gone through. Thanks also go to Dr. C. Randy Gallistel for his idea to use Bayesian -v- methods in chapter 4—the exercise has been truly instructive and will continue to serve me well in future endeavours. The Centre for Studies in Behavioural Neurobiology, and especially its techni- cal officer, David Munro, and system manager, Steve Cabillio, are owed much grati- tude. Without them, the PREF3 computerized system for data acquisition would still be a collection of Med-Associates levers and plexiglas sheets in a closet somewhere. In fact, I’d like to extend my thanks to the students of the CSBN, who provided the necessary moral support when times were tough, the necessary scientific scepticism when bouncing ideas, and the necessary companionship when came time to celebrate. It has been an honour and a pleasure to work alongside you all. Family, friends, and partner—thank you. Thank you mom, dad, Sacha, Michael, Andrew, Mark, Mehdi, Stephanie, Dean, Sherri, Amélie, Erin, and, obviously, Tiana, for dealing with my craziness while writing this. I really couldn’t ask for a better support system if I wanted to. Thank you for being there, for not slapping me silly, and, when warranted, for figuratively slapping sense into me. Many thanks to Dr. A. David Redish for his understanding, and the entire Redish lab for their help. I promise to get right back to post-doctoral work as soon as humanly possible. Finally, I would like to thank some of the people who paid for this. Financial support was provided by a Bourse de doctorat en Recherche grant from the Fonds Québécois de la Recherche sur la Nature et les Technologies. One last note: if I forgot to mention you, but should have, my apologies. Writing a thesis is a lot like what I’m told is involved in tending to a newborn: your sleep-wake cycle is disrupted, your eating patterns are erratic, you obsess over little things, and you worry about screwing up in some major way. There’s also some crying in the middle of the night, but that’s beside the point. It’s easy to lose track of everyone who had a hand in your success. Thank you! -vi- Dedication For Miguelina Gomez-Walsh and Jean-Daniel Breton. - vii - Table of Contents List of Figures xiii 1 Background 1 1.1Abriefhistoryofthepsychophysicsofreward............. 2 1.1.1 The patterning of behaviour ................... 5 1.2Actionselection.............................. 6 1.2.1 Classical conditioning ....................... 7 1.2.2 Instrumental conditioning .................... 8 1.2.3 Experimentalcontrol....................... 10 1.2.4 “Cognitive”actionselection................... 12 1.3 Computational models .......................... 13 1.3.1 Markov decision processes .................... 16 1.3.2 Unsupervised learning ...................... 22 1.3.3 The matching law ......................... 33 1.4Neuralsubstrates............................. 35 1.4.1 Medial forebrain bundle ..................... 35 1.4.2 Orbitofrontal cortex and task modelling ............ 37 1.4.3 Ventralstriatumandvalueupdating.............. 38 1.4.4 Dorsalstriatumandresponse-outcomegating......... 38 1.5Thisthesis................................. 39 1.5.1 A computational molar model .................. 39 1.5.2 Worldmodels........................... 42 1.5.3 A computational molecular model ................ 43 2 A computational molar model of performance for brain stimulation reward 45 - viii - 2.1Introduction................................ 45 2.1.1 The Shizgal Mountain Model .................. 46 2.1.2 Probability discounting ...................... 57 2.2Methods.................................. 63 2.2.1 Surgicalprocedure........................ 63 2.2.2 Behaviouralprotocol....................... 64 2.2.3 Statisticalanalysis........................ 69 2.3Results................................... 71 2.3.1 Stability of Fhm and Pe ...................... 71 2.3.2 Effects of probability on Fhm and Pe .............. 73 2.3.3 Magnitude of the difference in Fhm and Pe ........... 79 2.4Discussion................................. 82 2.4.1 Utility of the mountain model .................. 82 2.4.2 Aratprospecttheory?...................... 84 2.4.3 Generaldiscussion........................ 88 3 The rat’s world model of session structure 90 3.1Introduction................................ 90 3.1.1 WorldModel..........................
Details
-
File Typepdf
-
Upload Time-
-
Content LanguagesEnglish
-
Upload UserAnonymous/Not logged-in
-
File Pages352 Page
-
File Size-